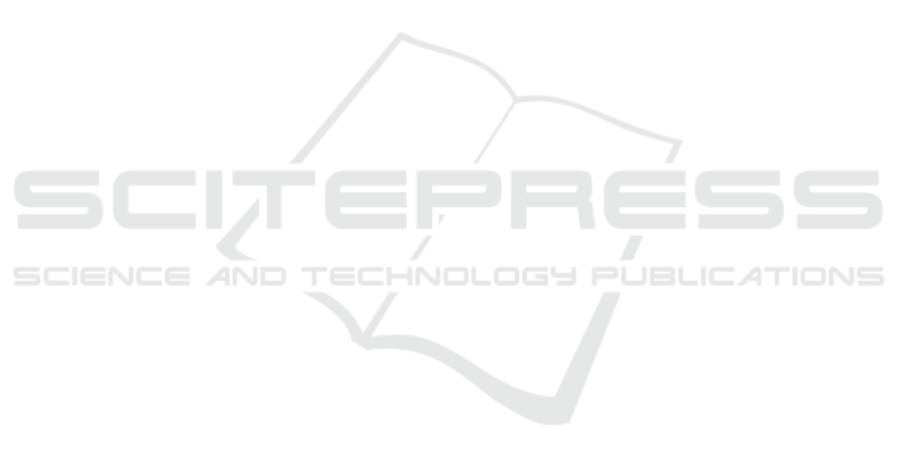
works. IEEE Transactions on Pattern Analysis and
Machine Intelligence, 38(2):295–307.
Dosovitskiy, A., Beyer, L., Kolesnikov, A., Weissenborn,
D., Zhai, X., Unterthiner, T., Dehghani, M., Minderer,
M., Heigold, G., Gelly, S., et al. (2021). An image is
worth 16x16 words: Transformers for image recogni-
tion at scale. In International Conference on Learning
Representations.
He, J., Yuan, Q., Li, J., Xiao, Y., Liu, X., and Zou, Y.
(2022). DsTer: A dense spectral transformer for re-
mote sensing spectral super-resolution. International
Journal of Applied Earth Observation and Geoinfor-
mation, 109:102773.
Ibrahim, M. R., Benavente, R., Lumbreras, F., and Ponsa,
D. (2022). 3DRRDB: Super Resolution of Multiple
Remote Sensing Images using 3D Residual in Resid-
ual Dense Blocks. In 2022 IEEE/CVF Conference on
Computer Vision and Pattern Recognition Workshops
(CVPRW), pages 322–331.
Ibrahim, M. R., Benavente, R., Ponsa, D., and Lumbreras,
F. (2024). Unveiling the Influence of Image Super-
Resolution on Aerial Scene Classification. In Vas-
concelos, V., Domingues, I., and Paredes, S., edi-
tors, Progress in Pattern Recognition, Image Analysis,
Computer Vision, and Applications, Lecture Notes in
Computer Science, pages 214–228.
Jiang, K., Wang, Z., Yi, P., Jiang, J., Xiao, J., and Yao,
Y. (2018). Deep Distillation Recursive Network for
Remote Sensing Imagery Super-Resolution. Remote
Sensing, 10(11):1700.
Kim, J., Lee, J. K., and Lee, K. M. (2016). Accurate Im-
age Super-Resolution Using Very Deep Convolutional
Networks. In 2016 IEEE Conference on Computer
Vision and Pattern Recognition (CVPR), pages 1646–
1654.
Lanaras, C., Bioucas-Dias, J., Galliani, S., Baltsavias,
E., and Schindler, K. (2018). Super-resolution of
Sentinel-2 images: Learning a globally applicable
deep neural network. ISPRS Journal of Photogram-
metry and Remote Sensing, 146:305–319.
Ledig, C. and et al. (2017). Photo-Realistic Single Im-
age Super-Resolution Using a Generative Adversar-
ial Network. In 2017 IEEE Conference on Computer
Vision and Pattern Recognition (CVPR), pages 105–
114.
Liang, J., Cao, J., Sun, G., Zhang, K., Van Gool, L., and
Timofte, R. (2021). SwinIR: Image Restoration Using
Swin Transformer. In 2021 IEEE/CVF International
Conference on Computer Vision Workshops (ICCVW),
pages 1833–1844.
Lim, B., Son, S., Kim, H., Nah, S., and Lee, K. M. (2017).
Enhanced Deep Residual Networks for Single Im-
age Super-Resolution. In 2017 IEEE Conference on
Computer Vision and Pattern Recognition Workshops
(CVPRW), pages 1132–1140.
Liu, Z., Lin, Y., Cao, Y., Hu, H., Wei, Y., Zhang, Z., Lin, S.,
and Guo, B. (2021). Swin Transformer: Hierarchical
Vision Transformer using Shifted Windows. In 2021
IEEE/CVF International Conference on Computer Vi-
sion (ICCV), pages 9992–10002.
Shi, W., Caballero, J., Huszar, F., Totz, J., Aitken, A. P.,
Bishop, R., Rueckert, D., and Wang, Z. (2016). Real-
Time Single Image and Video Super-Resolution Us-
ing an Efficient Sub-Pixel Convolutional Neural Net-
work. In 2016 IEEE Conference on Computer Vision
and Pattern Recognition (CVPR), pages 1874–1883.
Tuna, C., Unal, G., and Sertel, E. (2018). Single-frame su-
per resolution of remote-sensing images by convolu-
tional neural networks. International Journal of Re-
mote Sensing, 39(8):2463–2479.
Wang, J., Gao, K., Zhang, Z., Ni, C., Hu, Z., Chen, D., and
Wu, Q. (2021). Multisensor Remote Sensing Imagery
Super-Resolution with Conditional GAN. Journal of
Remote Sensing, 2021:2021/9829706.
Wang, P., Bayram, B., and Sertel, E. (2022a). A compre-
hensive review on deep learning based remote sensing
image super-resolution methods. Earth-Science Re-
views, 232:104110.
Wang, X., Yu, K., Wu, S., Gu, J., Liu, Y., Dong, C., Qiao, Y.,
and Loy, C. C. (2019). ESRGAN: Enhanced Super-
Resolution Generative Adversarial Networks. In Leal-
Taix
´
e, L. and Roth, S., editors, Computer Vision –
ECCV 2018 Workshops, volume 11133, pages 63–79.
Springer International Publishing, Cham.
Wang, Z., Li, L., Xue, Y., Jiang, C., Wang, J., Sun, K., and
Ma, H. (2022b). FeNet: Feature Enhancement Net-
work for Lightweight Remote-Sensing Image Super-
Resolution. IEEE Transactions on Geoscience and
Remote Sensing, 60:1–12.
Woo, S., Park, J., Lee, J.-Y., and Kweon, I. S. (2018).
CBAM: Convolutional Block Attention Module. In
Ferrari, V., Hebert, M., Sminchisescu, C., and Weiss,
Y., editors, Computer Vision – ECCV 2018, Lecture
Notes in Computer Science, pages 3–19.
Xiao, T., Singh, M., Mintun, E., Darrell, T., Dollar, P., and
Girshick, R. (2021). Early Convolutions Help Trans-
formers See Better. In Advances in Neural Informa-
tion Processing Systems, volume 34, pages 30392–
30400.
Zhang, C., Jiang, W., Zhang, Y., Wang, W., Zhao, Q.,
and Wang, C. (2022). Transformer and CNN Hy-
brid Deep Neural Network for Semantic Segmentation
of Very-High-Resolution Remote Sensing Imagery.
IEEE Transactions on Geoscience and Remote Sens-
ing, 60:1–20.
Zhang, K., Li, Y., Zuo, W., Zhang, L., Van Gool, L., and
Timofte, R. (2021). Plug-and-Play Image Restoration
with Deep Denoiser Prior. IEEE Transactions on Pat-
tern Analysis and Machine Intelligence, pages 1–1.
Zhang, Y. and et al. (2018). Residual Dense Network for
Image Super-Resolution. In 2018 IEEE/CVF Con-
ference on Computer Vision and Pattern Recognition,
pages 2472–2481.
Zhang, Y., Li, K., Li, K., Wang, L., Zhong, B., and Fu,
Y. (2018). Image Super-Resolution Using Very Deep
Residual Channel Attention Networks. In Ferrari, V.,
Hebert, M., Sminchisescu, C., and Weiss, Y., editors,
Computer Vision – ECCV 2018, volume 11211, pages
294–310. Springer International Publishing, Cham.
VISAPP 2024 - 19th International Conference on Computer Vision Theory and Applications
582