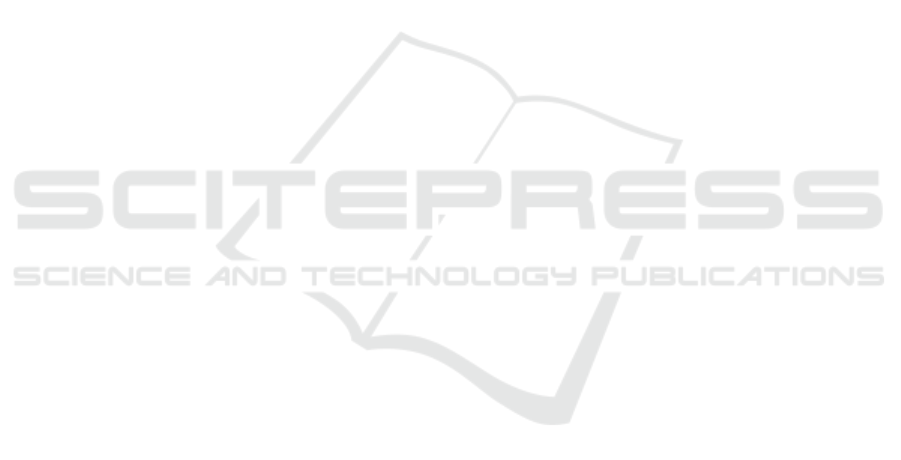
defects on the prominent areas of paper images de-
tected by an edge detector, is the first technique in the
literature that aims to utilize visual anomaly detection
approaches in paper applications. Through the evalu-
ation of our method on the paper images, we demon-
strate that the proposed method not only has the su-
perior ability to detect and locate different types of
unknown anomalies but also can properly deal with
the inherent complexities of the paper web defect de-
tection problem such as the effects of large and small
noises, as well as the presence of irrelevant objects
and dirty backgrounds. Showing high accuracy, low
false detection rate and low false negative rate makes
our approach a suitable candidate for detecting paper
irregularities in real-world applications.
REFERENCES
Adarsh, P., Rathi, P., and Kumar, M. (2020). Yolo v3-tiny:
Object detection and recognition using one stage im-
proved model. In 2020 6th international conference
on advanced computing and communication systems
(ICACCS), pages 687–694. IEEE.
Ahola, T. and Juuso, E. (2006). Paper web break sensi-
tivity monitoring in modern paper machines. In Pro-
ceedings of the 11th International Conference, IPMU
2006, Information Processing and Management of
Uncertainty in Knowledge-based Systems, 2006, Les
Cordeliers, Paris, volume 1, pages 518–525.
Alabadi, M. and Celik, Y. (2020). Anomaly detection for
cyber-security based on convolution neural network :
A survey. In 2020 International Congress on Human-
Computer Interaction, Optimization and Robotic Ap-
plications (HORA), pages 1–14.
An, J. and Cho, S. (2015). Variational autoencoder based
anomaly detection using reconstruction probability.
Bergmann, P., L
¨
owe, S., Fauser, M., Sattlegger, D., and Ste-
ger, C. (2018). Improving unsupervised defect seg-
mentation by applying structural similarity to autoen-
coders. arXiv preprint arXiv:1807.02011.
Bionda, A., Frittoli, L., and Boracchi, G. (2022). Deep au-
toencoders for anomaly detection in textured images
using cw-ssim. In International Conference on Image
Analysis and Processing, pages 669–680. Springer.
Bonissone, P. P. and Goebel, K. (2002). When will it
break? a hybrid soft computing model to predict time-
to-break margins in paper machines. In Applications
and Science of Neural Networks, and Fuzzy Systems,
volume 4787, pages 53–64. SPIE.
Canny, J. (1986). A computational approach to edge de-
tection. IEEE Transactions on pattern analysis and
machine intelligence, (6):679–698.
Chandola, V., Banerjee, A., and Kumar, V. (2009).
Anomaly detection: A survey. ACM computing sur-
veys (CSUR), 41(3):1–58.
Dias, M., Lourenc¸o, N., Silva, C., and Moniz, S. (2021).
The break point: A machine learning approach to web
breaks in paper mills. In Congress of APDIO, the Por-
tuguese Operational Research Society, pages 73–85.
Dini, A. and Rahtu, E. (2022). Tpsad: Learning to detect
and localize anomalies with thin plate spline transfor-
mation. 2022 26th International Conference on Pat-
tern Recognition (ICPR), pages 4744–4750.
Dini, A. and Rahtu, E. (2023). Visual anomaly detec-
tion and localization with a patch-wise transformer
and convolutional model. In International Joint Con-
ference on Computer Vision, Imaging and Computer
Graphics Theory and Applications. SCITEPRESS.
Fernando, T., Gammulle, H., Denman, S., Sridharan, S.,
and Fookes, C. (2021). Deep learning for medical
anomaly detection–a survey. ACM Computing Sur-
veys (CSUR), 54(7):1–37.
Haile, A., Gelebo, G. G., Tesfaye, T., Mengie, W., Mebrate,
M. A., Abuhay, A., and Limeneh, D. Y. (2021). Pulp
and paper mill wastes: utilizations and prospects for
high value-added biomaterials. Bioresources and Bio-
processing, 8:1–22.
Hassan, M. and Bhagvati, C. (2012). Structural similarity
measure for color images. International Journal of
Computer Applications, 43(14):7–12.
Li, C.-L., Sohn, K., Yoon, J., and Pfister, T. (2021). Cut-
paste: Self-supervised learning for anomaly detection
and localization. In Proceedings of the IEEE/CVF
conference on computer vision and pattern recogni-
tion, pages 9664–9674.
Liu, J., Xie, G., Wang, J., Li, S., Wang, C., Zheng, F., and
Jin, Y. (2023). Deep industrial image anomaly detec-
tion: A survey. arXiv preprint arXiv:2301.11514, 2.
Pang, G., Shen, C., Cao, L., and Hengel, A. V. D. (2021).
Deep learning for anomaly detection: A review. ACM
computing surveys (CSUR), 54(2):1–38.
Rippel, O. and Merhof, D. (2023). Anomaly detection for
automated visual inspection: A review. Bildverar-
beitung in der Automation: Ausgew
¨
ahlte Beitr
¨
age des
Jahreskolloquiums BVAu 2022, pages 1–13.
Sorsa, T., Koivo, H., and Korhonen, R. (1992). Application
of neural networks in the detection of breaks in a paper
machine. IFAC Proceedings Volumes, 25(4):219–224.
Tsai, D.-M. and Jen, P.-H. (2021). Autoencoder-based
anomaly detection for surface defect inspection. Ad-
vanced Engineering Informatics, 48:101272.
Yang, J., Xu, R., Qi, Z., and Shi, Y. (2021). Visual
anomaly detection for images: A survey. arXiv
preprint arXiv:2109.13157.
Yi, J. and Yoon, S. (2020). Patch svdd: Patch-level svdd for
anomaly detection and segmentation. In Proceedings
of the Asian conference on computer vision.
Anomaly Detection and Localization for Images of Running Paper Web in Paper Manufacturing
685