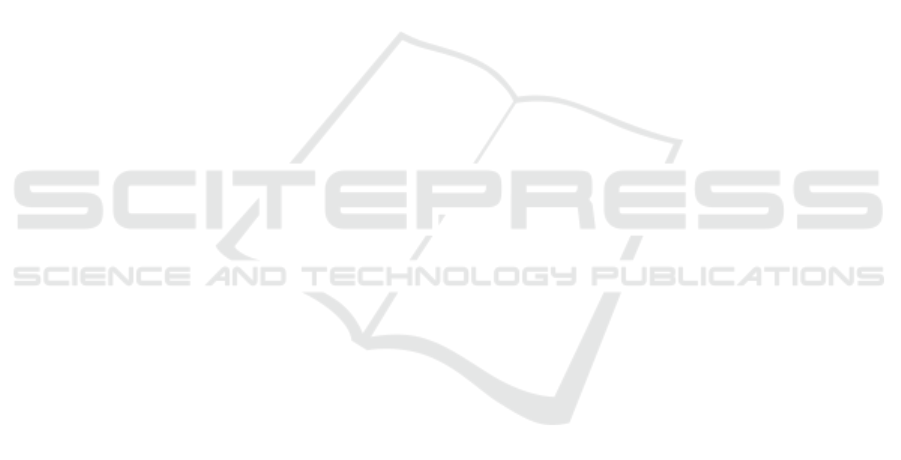
ACKNOWLEDGEMENTS
This work has been partially supported by EU DUCA,
EU CyberSecPro, SYNAPSE, PTR 22-24 P2.01 (Cy-
bersecurity) and SERICS (PE00000014) under the
MUR National Recovery and Resilience Plan funded
by the EU - NextGenerationEU projects.
REFERENCES
Ammenwerth, E., Nyk
¨
anen, P., Rigby, M., and de Keizer,
N. (2013). Clinical decision support systems: need
for evidence, need for evaluation.
Bacci, A., Bartoli, A., Martinelli, F., Medvet, E., and Mer-
caldo, F. (2018). Detection of obfuscation techniques
in android applications. In Proceedings of the 13th In-
ternational Conference on Availability, Reliability and
Security, pages 1–9.
Balestrino, R. and Schapira, A. (2020). Parkinson disease.
European journal of neurology, 27(1):27–42.
Chakraborty, S., Aich, S., Han, E., Park, J., Kim, H.-C.,
et al. (2020). Parkinson’s disease detection from spi-
ral and wave drawings using convolutional neural net-
works: A multistage classifier approach. In 2020 22nd
International Conference on Advanced Communica-
tion Technology (ICACT), pages 298–303. IEEE.
Chatterjee, D. and Kordower, J. H. (2019). Immunother-
apy in parkinson’s disease: Current status and future
directions. Neurobiology of disease, 132:104587.
Chen, H., Liu, J., Hua, C., Feng, J., Pang, B., Cao, D., and
Li, C. (2022). Accurate classification of white blood
cells by coupling pre-trained resnet and densenet with
scam mechanism. BMC bioinformatics, 23(1):1–20.
Cimitile, A., Martinelli, F., Mercaldo, F., et al. (2017). Ma-
chine learning meets ios malware: Identifying mali-
cious applications on apple environment. In ICISSP,
pages 487–492.
Dreiseitl, S. and Binder, M. (2005). Do physicians value de-
cision support? a look at the effect of decision support
systems on physician opinion. Artificial intelligence
in medicine, 33(1):25–30.
Drot
´
ar, P., Mekyska, J., Rektorov
´
a, I., Masarov
´
a, L.,
Sm
´
ekal, Z., and Faundez-Zanuy, M. (2016). Evalu-
ation of handwriting kinematics and pressure for dif-
ferential diagnosis of parkinson’s disease. Artificial
intelligence in Medicine, 67:39–46.
Gallicchio, C., Micheli, A., and Pedrelli, L. (2018). Deep
echo state networks for diagnosis of parkinson’s dis-
ease. arXiv preprint arXiv:1802.06708.
Gil-Mart
´
ın, M., Montero, J. M., and San-Segundo, R.
(2019). Parkinson’s disease detection from draw-
ing movements using convolutional neural networks.
Electronics, 8(8):907.
G
´
omez-Garc
´
ıa, J. A., Moro-Vel
´
azquez, L., and Godino-
Llorente, J. I. (2019). On the design of automatic
voice condition analysis systems. part ii: Review of
speaker recognition techniques and study on the ef-
fects of different variability factors. Biomedical Sig-
nal Processing and Control, 48:128–143.
He, K., Zhang, X., Ren, S., and Sun, J. (2016). Deep resid-
ual learning for image recognition. In Proceedings of
the IEEE conference on computer vision and pattern
recognition, pages 770–778.
Huang, G., Liu, Z., Pleiss, G., Van Der Maaten, L., and
Weinberger, K. Q. (2019). Convolutional networks
with dense connectivity. IEEE transactions on pat-
tern analysis and machine intelligence, 44(12):8704–
8716.
Huang, P., He, P., Tian, S., Ma, M., Feng, P., Xiao, H.,
Mercaldo, F., Santone, A., and Qin, J. (2022). A vit-
amc network with adaptive model fusion and multiob-
jective optimization for interpretable laryngeal tumor
grading from histopathological images. IEEE Trans-
actions on Medical Imaging, 42(1):15–28.
Huang, P., Tan, X., Zhou, X., Liu, S., Mercaldo, F., and
Santone, A. (2021). Fabnet: fusion attention block
and transfer learning for laryngeal cancer tumor grad-
ing in p63 ihc histopathology images. IEEE Journal
of Biomedical and Health Informatics, 26(4):1696–
1707.
Huang, P., Zhou, X., He, P., Feng, P., Tian, S., Sun, Y., Mer-
caldo, F., Santone, A., Qin, J., and Xiao, H. (2023).
Interpretable laryngeal tumor grading of histopatho-
logical images via depth domain adaptive network
with integration gradient cam and priori experience-
guided attention. Computers in Biology and Medicine,
154:106447.
Impedovo, D. and Pirlo, G. (2018). Dynamic handwriting
analysis for the assessment of neurodegenerative dis-
eases: a pattern recognition perspective. IEEE reviews
in biomedical engineering, 12:209–220.
Impedovo, D. and Pirlo, G. (2019). Online handwriting
analysis for the assessment of alzheimer’s disease and
parkinson’s disease: overview and experimental in-
vestigation. Frontiers In Pattern Recognition And Ar-
tificial Intelligence, pages 113–128.
Kamble, M., Shrivastava, P., and Jain, M. (2021). Digi-
tized spiral drawing classification for parkinson’s dis-
ease diagnosis. Measurement: Sensors, 16:100047.
Khatamino, P., Cant
¨
urk,
˙
I., and
¨
Ozyılmaz, L. (2018). A
deep learning-cnn based system for medical diagno-
sis: An application on parkinson’s disease handwrit-
ing drawings. In 2018 6th International Conference
on Control Engineering & Information Technology
(CEIT), pages 1–6. IEEE.
Kotsavasiloglou, C., Kostikis, N., Hristu-Varsakelis, D., and
Arnaoutoglou, M. (2017). Machine learning-based
classification of simple drawing movements in parkin-
son’s disease. Biomedical Signal Processing and Con-
trol, 31:174–180.
Krizhevsky, A., Sutskever, I., and Hinton, G. E. (2012). Im-
agenet classification with deep convolutional neural
networks. Advances in neural information processing
systems, 25.
Lahmiri, S. and Shmuel, A. (2019). Detection of parkin-
son’s disease based on voice patterns ranking and op-
Spiral Drawing Test and Explainable Convolutional Neural Networks for Parkinson’s Disease Detection
451