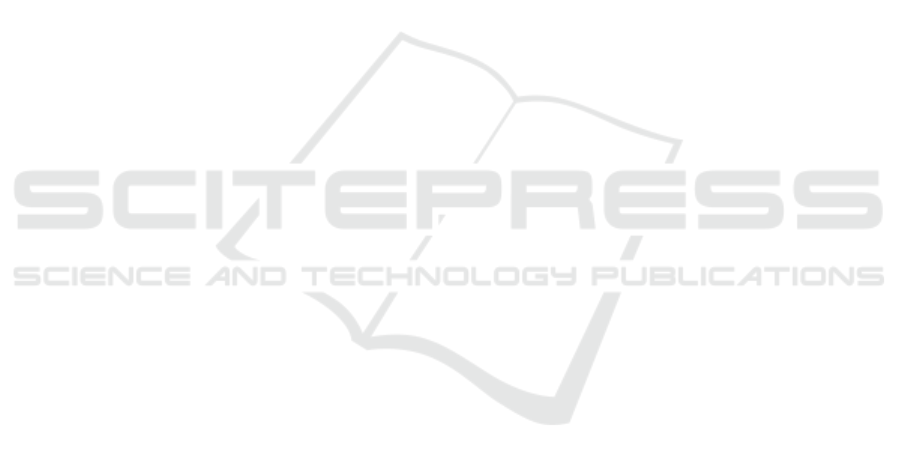
documents. Proceedings of the IEEE, 87(7):1181–
1196.
Castillo Camacho, I. and Wang, K. (2022). Convolu-
tional neural network initialization approaches for im-
age manipulation detection. Digital Signal Process-
ing, 122:103376.
Choi, J.-H., Lee, H.-Y., and Lee, H.-K. (2013). Color laser
printer forensic based on noisy feature and support
vector machine classifier. Multimedia Tools and Ap-
plications, 67:363–382.
Cruz, F., Sidere, N., Coustaty, M., d’Andecy, V. P., and
Ogier, J.-M. (2017). Local binary patterns for doc-
ument forgery detection. In International Conference
on Document Analysis and Recognition (ICDAR), vol-
ume 1, pages 1223–1228.
Cruz, F., Sid
`
ere, N., Coustaty, M., Poulain D’Andecy, V.,
and Ogier, J. (2018). Categorization of document im-
age tampering techniques and how to identify them.
In International Workshop on Computational Foren-
sics (IWCF), pages 117–124.
Fridrich, J. and Kodovsky, J. (2012). Rich models for ste-
ganalysis of digital images. IEEE Transactions on In-
formation Forensics and Security, 7(3):868–882.
Glorot, X. and Bengio, Y. (2010). Understanding the dif-
ficulty of training deep feedforward neural networks.
In International Conference on Artificial Intelligence
and Statistics (AISTATS), pages 249–256.
Golan, I. and El-Yaniv, R. (2018). Deep anomaly detection
using geometric transformations. In Advances in Neu-
ral Information Processing Systems (NeurIPS), pages
9781–9791.
Gomez-Kr
¨
amer, P. (2022). Verifying document intergrity.
In Multimedia Security 2: Biometrics, Video Surveil-
lance and Multimedia Encryption, volume 2, pages
59–89. Wiley-ISTE.
Gomez-Kr
¨
amer, P., Rouis, K., Diallo, A. O., and Coustaty,
M. (2023). Printed and scanned document authentica-
tion using robust layout descriptor matching. Multi-
media Tools and Applications.
He, K., Zhang, X., Ren, S., and Sun, J. (2015). Delving deep
into rectifiers: Surpassing human-level performance
on imagenet classification. In International Confer-
ence on Computer Vision (ICCV), pages 1026–1034.
He, K., Zhang, X., Ren, S., and Sun, J. (2016). Deep
residual learning for image recognition. In Conference
on Computer Vision and Pattern Recognition (CVPR),
pages 770–778.
Huang, K., Tian, X., Yu, H., Yu, M., and Yin, A. (2019). A
high capacity watermarking technique for the printed
document. Electronics, 8(12):1403.
James, H., Gupta, O., and Raviv, D. (2020). OCR graph fea-
tures for manipulation detection in documents. CoRR,
abs/2009.05158.
Joren, H., Gupta, O., and Raviv, D. (2022). Learning
document graphs with attention for image manipula-
tion detection. In International Conference on Pat-
tern Recognition and Artificial Intelligence (ICPRAI),
pages 263–274.
Joshi, S. and Khanna, N. (2020). Source printer classifi-
cation using printer specific local texture descriptor.
Transactions on Information Forensics and Security,
15:160–171.
Nandanwar, L., Shivakumara, P., Pal, U., Lu, T., Lopresti,
D., Seraogi, B., and Chaudhuri, B. B. (2021). A new
method for detecting altered text in document images.
In International Conference of Pattern Recognition
and Artificial Intelligence (ICPRAI), pages 93–108.
Raiko, T., Valpola, H., and LeCun, Y. (2012). Deep learning
made easier by linear transformations in perceptrons.
In Conference on Artificial Intelligence and Statistics
(AISTATS), pages 924–932.
Robbins, H. and Monro, S. (1951). A stochastic approxima-
tion method. The Annals of Mathematical Statistics,
pages 400–407.
Schlegl, T., Seeb
¨
ock, P., Waldstein, S. M., Schmidt-Erfurth,
U., and Langs, G. (2017). Unsupervised anomaly de-
tection with generative adversarial networks to guide
marker discovery. In International Conference on
Information Processing in Medical Imaging (IPMI),
pages 146–157.
Shang, S., Kong, X., and You, X. (2015). Document forgery
detection using distortion mutation of geometric pa-
rameters in characters. Journal of Electronic Imaging,
24(2):1 – 10.
Tan, L. and Sun, X. (2011). Robust text hashing for content-
based document authentication. Information Technol-
ogy Journal, 10(8):1608–1613.
VISAPP 2024 - 19th International Conference on Computer Vision Theory and Applications
574