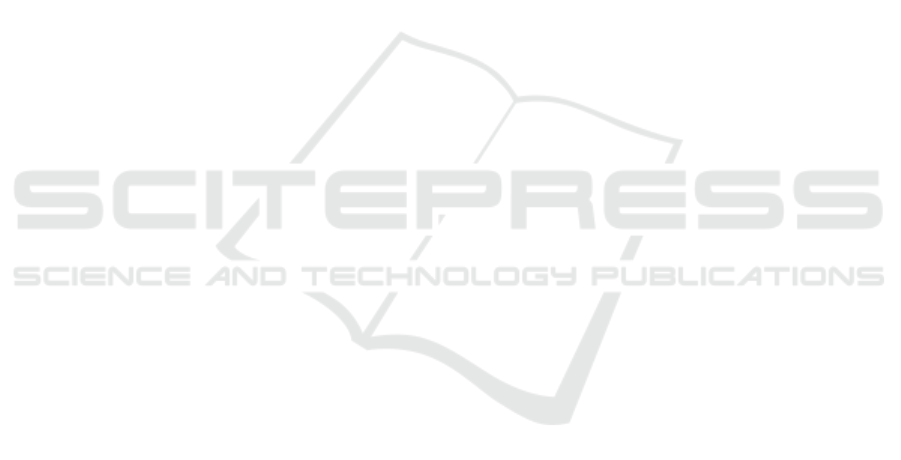
hance the object detection model further. Spatial in-
formation of the objects has been preserved during the
-agnostic detection and ROI pooling stages, ensuring
accurate annotation preparation. The model has been
fine-tuned on weakly generated datasets, focusing on
the targeted class, resulting in improved object detec-
tion capabilities.
Evaluation of the custom-trained model has
demonstrated its effectiveness in detecting and local-
izing the targeted class of objects. The integration
of clustering, weakly generated data, spatial preser-
vation, and custom training has contributed to the
overall success of the proposed approach. This re-
search provides new insights into unsupervised novel
object detection, addressing the challenges of limited
labelled data for novel objects. The methodology pre-
sented in this paper offers a practical framework for
detecting and localizing novel objects in various do-
mains, paving the way for advancements in computer
vision and object detection research. Future work can
focus on extending this approach to real-time applica-
tions and exploring additional techniques to enhance
the accuracy and efficiency of unsupervised novel ob-
ject detection systems.
REFERENCES
Bao, Z., Tokmakov, P., Jabri, A., Wang, Y.-X., Gaidon, A.,
and Hebert, M. (2022). Discovering objects that can
move. In Proceedings of the IEEE/CVF Conference
on Computer Vision and Pattern Recognition, pages
11789–11798.
Blatter, P., Kanakis, M., Danelljan, M., and Van Gool,
L. (2023). Efficient visual tracking with exemplar
transformers. In Proceedings of the IEEE/CVF Win-
ter Conference on Applications of Computer Vision,
pages 1571–1581.
Cheng, G., Wang, J., Li, K., Xie, X., Lang, C., Yao, Y., and
Han, J. (2022). Anchor-free oriented proposal gener-
ator for object detection. IEEE Transactions on Geo-
science and Remote Sensing, 60:1–11.
Fan, Q., Zhuo, W., Tang, C.-K., and Tai, Y.-W. (2020). Few-
shot object detection with attention-rpn and multi-
relation detector. In Proceedings of the IEEE/CVF
conference on computer vision and pattern recogni-
tion, pages 4013–4022.
Girshick, R. (2015). Fast r-cnn. In Proceedings of the IEEE
international conference on computer vision, pages
1440–1448.
He, K., Gkioxari, G., Doll
´
ar, P., and Girshick, R. (2017).
Mask r-cnn. In Proceedings of the IEEE international
conference on computer vision, pages 2961–2969.
H
´
enaff, O. J., Koppula, S., Shelhamer, E., Zoran, D.,
Jaegle, A., Zisserman, A., Carreira, J., and Arand-
jelovi
´
c, R. (2022). Object discovery and representa-
tion networks. In Computer Vision–ECCV 2022: 17th
European Conference, Tel Aviv, Israel, October 23–
27, 2022, Proceedings, Part XXVII, pages 123–143.
Springer.
Hou, L., Lu, K., Xue, J., and Li, Y. (2022). Shape-adaptive
selection and measurement for oriented object detec-
tion. In Proceedings of the AAAI Conference on Arti-
ficial Intelligence, volume 36, pages 923–932.
Kang, B., Liu, Z., Wang, X., Yu, F., Feng, J., and Dar-
rell, T. (2019). Few-shot object detection via feature
reweighting.
Kim, D., Lin, T., Angelova, A., Kweon, I. S., and Kuo, W.
(2021). Learning open-world object proposals without
learning to classify. CoRR, abs/2108.06753.
Kuo, W., Hariharan, B., and Malik, J. (2015). Deepbox:
Learning objectness with convolutional networks.
LaBonte, T., Song, Y., Wang, X., Vineet, V., and Joshi, N.
(2023). Scaling novel object detection with weakly
supervised detection transformers. In Proceedings of
the IEEE/CVF Winter Conference on Applications of
Computer Vision, pages 85–96.
Li, W., Chen, Y., Hu, K., and Zhu, J. (2022a). Oriented
reppoints for aerial object detection. In Proceedings
of the IEEE/CVF Conference on Computer Vision and
Pattern Recognition, pages 1829–1838.
Li, Y., Mao, H., Girshick, R., and He, K. (2022b). Ex-
ploring plain vision transformer backbones for object
detection. In Computer Vision–ECCV 2022: 17th Eu-
ropean Conference, Tel Aviv, Israel, October 23–27,
2022, Proceedings, Part IX, pages 280–296. Springer.
Maaz, M., Rasheed, H., Khan, S., Khan, F. S., Anwer,
R. M., and Yang, M.-H. (2022). Class-agnostic ob-
ject detection with multi-modal transformer. In 17th
European Conference on Computer Vision (ECCV).
Springer.
O. Pinheiro, P. O., Collobert, R., and Dollar, P. (2015).
Learning to segment object candidates. In Cortes, C.,
Lawrence, N., Lee, D., Sugiyama, M., and Garnett,
R., editors, Advances in Neural Information Process-
ing Systems, volume 28. Curran Associates, Inc.
Park, K., Woo, S., Oh, S. W., Kweon, I. S., and Lee, J.-Y.
(2022). Per-clip video object segmentation. In Pro-
ceedings of the IEEE/CVF Conference on Computer
Vision and Pattern Recognition, pages 1352–1361.
Peng, J., Bu, X., Sun, M., Zhang, Z., Tan, T., and Yan, J.
(2020). Large-scale object detection in the wild from
imbalanced multi-labels. CoRR, abs/2005.08455.
Pont-Tuset, J., Arbelaez, P., T.Barron, J., Marques, F., and
Malik, J. (2017). Multiscale combinatorial grouping
for image segmentation and object proposal genera-
tion. IEEE Transactions on Pattern Analysis and Ma-
chine Intelligence, 39(1):128–140.
Qin, H., Yu, C., Gao, C., and Sang, N. (2022). D2t: A
framework for transferring detection to tracking. Pat-
tern Recognition, 126:108544.
Ren, S., He, K., Girshick, R., and Sun, J. (2015). Faster
r-cnn: Towards real-time object detection with region
proposal networks. Advances in neural information
processing systems, 28.
Tang, F. and Ling, Q. (2022). Ranking-based siamese visual
tracking. In Proceedings of the IEEE/CVF Conference
VISAPP 2024 - 19th International Conference on Computer Vision Theory and Applications
700