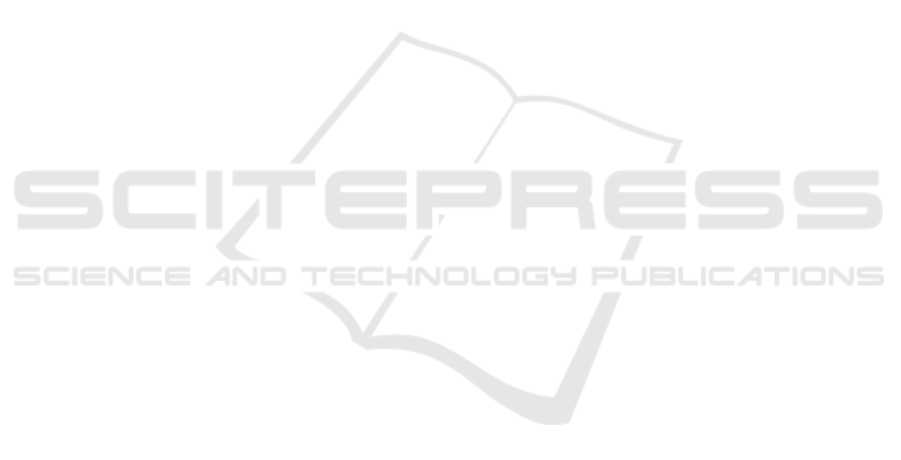
extreme learning machine. Cognitive Computation,
12(3):513–527.
Buckner, R. L., Krienen, F. M., Castellanos, A., Diaz, J. C.,
and Yeo, B. T. (2011). The organization of the hu-
man cerebellum estimated by intrinsic functional con-
nectivity. Journal of neurophysiology, 106(5):2322–
2345.
Bullmore, E. and Sporns, O. (2009). Complex brain net-
works: graph theoretical analysis of structural and
functional systems. Nature Reviews Neuroscience,
10(3):186–198.
Burges, C. J. (1998). A tutorial on support vector machines
for pattern recognition. Data mining and knowledge
discovery, 2(2):121–167.
Chandra, A., Dervenoulas, G., and Politis, M. (2019). Mag-
netic resonance imaging in Alzheimer’s disease and
mild cognitive impairment. Journal of Neurology,
266(6):1293–1302.
Chen, X., Li, B., Jia, H., Feng, F., Duan, F., Sun, Z., Ca-
iafa, C. F., and Sol
´
e-Casals, J. (2022). Graph em-
pirical mode decomposition-based data augmentation
applied to gifted children MRI analysis. Frontiers in
Neuroscience, 16:866735.
Errica, F., Podda, M., Bacciu, D., and Micheli, A. (2020).
A fair comparison of graph neural networks for graph
classification. In International Conference on Learn-
ing Representations (ICLR 2020).
Esteban, O., Markiewicz, C. J., Blair, R. W., Moodie, C. A.,
Isik, A. I., Erramuzpe, A., Kent, J. D., Goncalves, M.,
DuPre, E., Snyder, M., et al. (2019). fMRIPrep: a ro-
bust preprocessing pipeline for functional MRI. Na-
ture methods, 16(1):111–116.
Fey, M. and Lenssen, J. E. (2019). Fast graph represen-
tation learning with PyTorch Geometric. In ICLR
Workshop on Representation Learning on Graphs and
Manifolds.
Greve, D. N. and Fischl, B. (2009). Accurate and robust
brain image alignment using boundary-based registra-
tion. Neuroimage, 48(1):63–72.
Han, S., Sun, Z., Zhao, K., Duan, F., Caiafa, C. F., Zhang,
Y., and Sol
´
e-Casals, J. (2024). Early prediction of de-
mentia using fMRI data with a graph convolutional
network approach. Journal of Neural Engineering.
Submitted, under review.
Hanik, M., Demirtas¸, M. A., Gharsallaoui, M. A., and
Rekik, I. (2022). Predicting cognitive scores with
graph neural networks through sample selection learn-
ing. Brain Imaging and Behavior, 16(3):1123–1138.
Huijser, D. (2021). Functional connectivity changes and
cognitive deficits in Alzheimer’s disease: A review.
Katsumi, Y., Quimby, M., Hochberg, D., Jones, A., Brick-
house, M., Eldaief, M. C., Dickerson, B. C., and
Touroutoglou, A. (2023). Association of regional cor-
tical network atrophy with progression to dementia in
patients with primary progressive aphasia. Neurology,
100(3):e286–e296.
Kingma, D. P. and Ba, J. L. (2015). Adam: A method for
stochastic optimization. pages 1–13.
LaMontagne, P. J., Benzinger, T. L., Morris, J. C., Keefe,
S., Hornbeck, R., Xiong, C., Grant, E., Hassenstab, J.,
Moulder, K., Vlassenko, A. G., et al. (2019). OASIS-
3: longitudinal neuroimaging, clinical, and cogni-
tive dataset for normal aging and Alzheimer’s disease.
MedRxiv.
Li, H.-J., Hou, X.-H., Liu, H.-H., Yue, C.-L., He, Y., and
Zuo, X.-N. (2015). Toward systems neuroscience in
mild cognitive impairment and Alzheimer’s disease:
A meta-analysis of 75 fMRI studies. Human brain
mapping, 36(3):1217–1232.
Liu, F., Zhang, Y., Rekik, I., Massoud, Y., and Sol
´
e-Casals,
J. (2022). Graph learning for brain imaging. Frontiers
in Neuroscience, 16:1001818.
Liu, S., Cai, W., Liu, S., Zhang, F., Fulham, M., Feng,
D., Pujol, S., and Kikinis, R. (2015). Multimodal
neuroimaging computing: a review of the applica-
tions in neuropsychiatric disorders. Brain Informatics,
2(3):167–180.
Micheli, A. (2009). Neural network for graphs: A con-
textual constructive approach. IEEE Transactions on
Neural Networks, 20(3):498–511.
Morris, C., Ritzert, M., Fey, M., Hamilton, W. L., Lenssen,
J. E., Rattan, G., and Grohe, M. (2019). Weisfeiler and
leman go neural: Higher-order graph neural networks.
In Proceedings of the AAAI Conference on Artificial
Intelligence, volume 33, pages 4602–4609.
Nair, V. and Hinton, G. E. (2010). Rectified linear units
improve restricted boltzmann machines. In ICML.
Parisot, S., Ktena, S. I., Ferrante, E., Lee, M., Moreno,
R. G., Glocker, B., and Rueckert, D. (2017). Spectral
graph convolutions for population-based disease pre-
diction. In International Conference on Medical Im-
age Computing and Computer-assisted Intervention,
pages 177–185. Springer.
Pruim, R. H., Mennes, M., van Rooij, D., Llera, A., Buite-
laar, J. K., and Beckmann, C. F. (2015). Ica-aroma:
A robust ica-based strategy for removing motion arti-
facts from fMRI data. Neuroimage, 112:267–277.
Qu, Z., Yao, T., Liu, X., and Wang, G. (2023). A graph con-
volutional network based on univariate neurodegen-
eration biomarker for Alzheimer’s disease diagnosis.
IEEE Journal of Translational Engineering in Health
and Medicine.
Scarselli, F., Gori, M., Tsoi, A. C., Hagenbuchner, M.,
and Monfardini, G. (2008). The graph neural net-
work model. IEEE Transactions on Neural Networks,
20(1):61–80.
Schaefer, A., Kong, R., Gordon, E. M., Laumann, T. O.,
Zuo, X.-N., Holmes, A. J., Eickhoff, S. B., and Yeo,
B. T. (2018). Local-global parcellation of the human
cerebral cortex from intrinsic functional connectivity
MRI. Cerebral cortex, 28(9):3095–3114.
Sporns, O., Tononi, G., and K
¨
otter, R. (2005). The hu-
man connectome: a structural description of the hu-
man brain. PLoS computational biology, 1(4):e42.
Srivastava, S., Ahmad, R., and Khare, S. K. (2021).
Alzheimer’s disease and its treatment by different ap-
proaches: A review. European Journal of Medicinal
Chemistry, 216:113320.
Suprano, I. (2019). Cerebral connectivity study by func-
An Insight Into Neurodegeneration: Harnessing Functional MRI Connectivity in the Diagnosis of Mild Cognitive Impairment
665