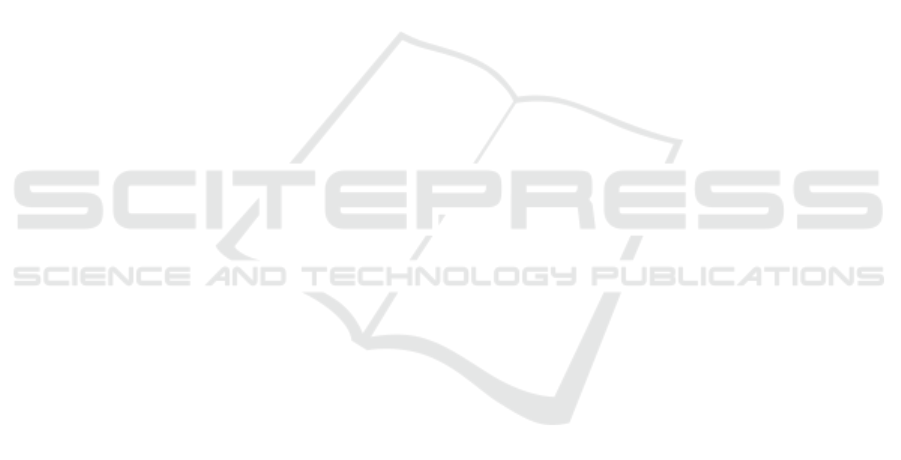
REFERENCES
Bengio, Y., Louradour, J., Collobert, R., and Weston, J.
(2009). Curriculum learning. In Proceedings of the
26th Annual International Conference on Machine
Learning, ICML ’09, page 41–48, New York, NY,
USA. Association for Computing Machinery.
Boominathan, L., Kruthiventi, S. S. S., and Babu, R. V.
(2016). Crowdnet: A deep convolutional network for
dense crowd counting. Proceedings of the 24th ACM
international conference on Multimedia.
Cao, X., Wang, Z., Zhao, Y., and Su, F. (2018). Scale aggre-
gation network for accurate and efficient crowd count-
ing. In ECCV.
Chan, A. B. and Vasconcelos, N. (2009). Bayesian poisson
regression for crowd counting. In 2009 IEEE 12th
International Conference on Computer Vision, pages
545–551.
Chen, K., Loy, C. C., Gong, S., and Xiang, T. (2012). Fea-
ture mining for localised crowd counting. In BMVC.
Chen, X. and Gupta, A. K. (2015). Webly supervised learn-
ing of convolutional networks. 2015 IEEE Interna-
tional Conference on Computer Vision (ICCV), pages
1431–1439.
Fan, Z., Zhang, H., Zhang, Z., Lu, G., Zhang, Y., and Wang,
Y. (2022). A survey of crowd counting and density es-
timation based on convolutional neural network. Neu-
rocomputing, 472:224–251.
Fang, Y., Zhan, B., Cai, W., Gao, S., and Hu, B. (2019).
Locality-constrained spatial transformer network for
video crowd counting. In 2019 IEEE International
Conference on Multimedia and Expo (ICME), pages
814–819.
Gao, C., Wang, P., and Gao, Y. (2019). Mobilecount:
An efficient encoder-decoder framework for real-time
crowd counting. In Pattern Recognition and Computer
Vision: Second Chinese Conference, PRCV 2019,
Xi’an, China, November 8–11, 2019, Proceedings,
Part II, page 582–595. Springer-Verlag.
Gouiaa, R., Akhloufi, M. A., and Shahbazi, M. (2021). Ad-
vances in convolution neural networks based crowd
counting and density estimation. Big Data Cogn.
Comput., 5:50.
Gu, S. and Lian, Z. (2022). A unified multi-task learning
framework of real-time drone supervision for crowd
counting. ArXiv, abs/2202.03843.
He, K., Zhang, X., Ren, S., and Sun, J. (2016). Deep resid-
ual learning for image recognition. 2016 IEEE Con-
ference on Computer Vision and Pattern Recognition
(CVPR), pages 770–778.
Idrees, H., Tayyab, M., Athrey, K., Zhang, D., Al-Maadeed,
S. A., Rajpoot, N. M., and Shah, M. (2018). Compo-
sition loss for counting, density map estimation and
localization in dense crowds. ArXiv, abs/1808.01050.
Ionescu, R. T., Alexe, B., Leordeanu, M., Popescu, M. C.,
Papadopoulos, D. P., and Ferrari, V. (2016). How hard
can it be? estimating the difficulty of visual search in
an image. 2016 IEEE Conference on Computer Vision
and Pattern Recognition (CVPR), pages 2157–2166.
Jiang, X., Xiao, Z., Zhang, B., Zhen, X., Cao, X., Doer-
mann, D. S., and Shao, L. (2019). Crowd counting
and density estimation by trellis encoder-decoder net-
works. 2019 IEEE/CVF Conference on Computer Vi-
sion and Pattern Recognition (CVPR), pages 6126–
6135.
Khan, M. A., Hamila, R., and Menouar, H. (2023a). Clip:
Train faster with less data. In 2023 IEEE International
Conference on Big Data and Smart Computing (Big-
Comp), pages 34–39. IEEE.
Khan, M. A., Menouar, H., and Hamila, R. (2022). Revisit-
ing Crowd Counting: State-of-the-art, Trends, and Fu-
ture Perspectives. arXiv preprint arXiv:2209.07271.
Khan, M. A., Menouar, H., and Hamila, R. (2023b). LCD-
net: a lightweight crowd density estimation model for
real-time video surveillance. Journal of Real-Time Im-
age Processing, 20:29.
Kocmi, T. and Bojar, O. (2017). Curriculum learning and
minibatch bucketing in neural machine translation. In
RANLP.
Krizhevsky, A. (2009). Learning multiple layers of features
from tiny images. Technical report.
Li, M., Zhang, Z., Huang, K., and Tan, T. (2008). Estimat-
ing the number of people in crowded scenes by mid
based foreground segmentation and head-shoulder de-
tection. In 2008 19th International Conference on Pat-
tern Recognition, pages 1–4.
Li, S., Zhu, X., Huang, Q., Xu, H., and Kuo, C.-C. J. (2017).
Multiple instance curriculum learning for weakly su-
pervised object detection. ArXiv, abs/1711.09191.
Li, W., Cao, Z., Wang, Q., Chen, S., and Feng, R. (2021).
Learning error-driven curriculum for crowd count-
ing. 2020 25th International Conference on Pattern
Recognition (ICPR), pages 843–849.
Li, Y., Zhang, X., and Chen, D. (2018). Csrnet: Dilated
convolutional neural networks for understanding the
highly congested scenes. 2018 IEEE/CVF Conference
on Computer Vision and Pattern Recognition, pages
1091–1100.
Ma, Z., Wei, X., Hong, X., and Gong, Y. (2019). Bayesian
loss for crowd count estimation with point supervi-
sion. In 2019 IEEE/CVF International Conference
on Computer Vision (ICCV), pages 6141–6150, Los
Alamitos, CA, USA.
Narvekar, S., Peng, B., Leonetti, M., Sinapov, J., Taylor,
M. E., and Stone, P. (2020). Curriculum learning for
reinforcement learning domains: A framework and
survey. J. Mach. Learn. Res., 21:181:1–181:50.
Peng, T., Li, Q., and Zhu, P. F. (2020). Rgb-t crowd count-
ing from drone: A benchmark and mmccn network. In
ACCV.
Platanios, E. A., Stretcu, O., Neubig, G., P
´
oczos, B., and
Mitchell, T. M. (2019). Competence-based curricu-
lum learning for neural machine translation. ArXiv,
abs/1903.09848.
Qi, W., Gao, J., Wei, L., and Yuan, Y. (2020). Pixel-wise
crowd understanding via synthetic data. International
Journal of Computer Vision, 129:225–245.
Sandler, M., Howard, A., Zhu, M., Zhmoginov, A., and
Chen, L.-C. (2018). Mobilenetv2: Inverted residu-
Curriculum for Crowd Counting: Is It Worthy?
589