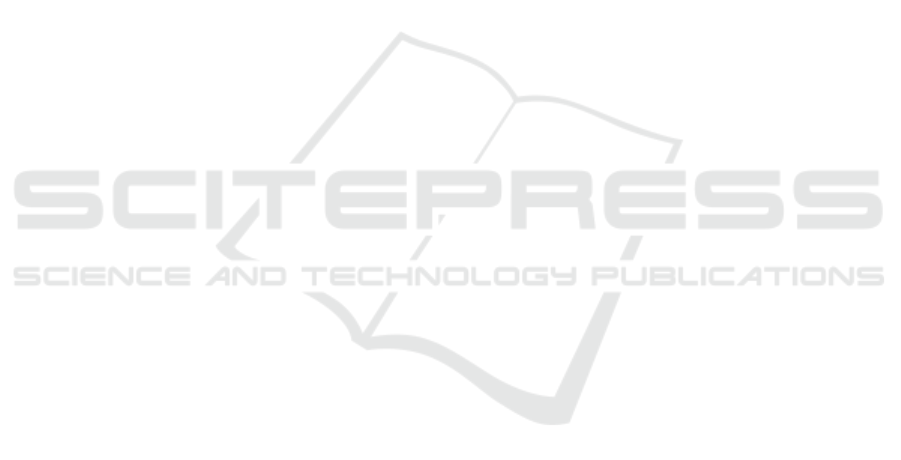
formers for image recognition at scale. In Interna-
tional Conference on Learning Representations.
Duong, H.-T., Le, V.-T., and Hoang, V. T. (2023). Deep
learning-based anomaly detection in video surveil-
lance: A survey. Sensors, 23(11):5024.
Fernando, T., Gammulle, H., Denman, S., Sridharan, S.,
and Fookes, C. (2021). Deep learning for medical
anomaly detection–a survey. ACM Computing Sur-
veys (CSUR), 54(7):1–37.
Haselmann, M., Gruber, D. P., and Tabatabai, P. (2018).
Anomaly detection using deep learning based image
completion. In 2018 17th IEEE international confer-
ence on machine learning and applications (ICMLA),
pages 1237–1242. IEEE.
Hassan, M. and Bhagvati, C. (2012). Structural similarity
measure for color images. International Journal of
Computer Applications, 43(14):7–12.
He, K., Chen, X., Xie, S., Li, Y., Doll
´
ar, P., and Girshick,
R. (2022). Masked autoencoders are scalable vision
learners. In Proceedings of the IEEE/CVF conference
on computer vision and pattern recognition, pages
16000–16009.
He, K., Zhang, X., Ren, S., and Sun, J. (2016). Deep resid-
ual learning for image recognition. In Proceedings of
the IEEE conference on computer vision and pattern
recognition, pages 770–778.
Hou, J., Zhang, Y., Zhong, Q., Xie, D., Pu, S., and Zhou, H.
(2021). Divide-and-assemble: Learning block-wise
memory for unsupervised anomaly detection. In Pro-
ceedings of the IEEE/CVF International Conference
on Computer Vision, pages 8791–8800.
Huang, C., Cao, J., Ye, F., Li, M., Zhang, Y., and Lu, C.
(2019). Inverse-transform autoencoder for anomaly
detection. arXiv preprint arXiv:1911.10676, 2(4).
Kumar, J. S., Anuar, S., and Hassan, N. H. (2022). Trans-
fer learning based performance comparison of the pre-
trained deep neural networks. International Journal of
Advanced Computer Science and Applications, 13(1).
Lee, Y. and Kang, P. (2022). Anovit: Unsuper-
vised anomaly detection and localization with vision
transformer-based encoder-decoder. IEEE Access,
10:46717–46724.
Li, C.-L., Sohn, K., Yoon, J., and Pfister, T. (2021). Cut-
paste: Self-supervised learning for anomaly detection
and localization. In Proceedings of the IEEE/CVF
conference on computer vision and pattern recogni-
tion, pages 9664–9674.
Li, H. and Li, Y. (2023). Anomaly detection methods based
on gan: a survey. Applied Intelligence, 53(7):8209–
8231.
Liu, J., Song, K., Feng, M., Yan, Y., Tu, Z., and Zhu, L.
(2021). Semi-supervised anomaly detection with dual
prototypes autoencoder for industrial surface inspec-
tion. Optics and Lasers in Engineering, 136:106324.
Liu, J., Xie, G., Wang, J., Li, S., Wang, C., Zheng, F., and
Jin, Y. (2023). Deep industrial image anomaly detec-
tion: A survey. arXiv preprint arXiv:2301.11514, 2.
Mishra, P., Verk, R., Fornasier, D., Piciarelli, C., and
Foresti, G. L. (2021). Vt-adl: A vision transformer
network for image anomaly detection and localization.
In 2021 IEEE 30th International Symposium on In-
dustrial Electronics (ISIE), pages 01–06. IEEE.
Mohammadi, B., Fathy, M., and Sabokrou, M. (2021). Im-
age/video deep anomaly detection: A survey. arXiv
preprint arXiv:2103.01739.
Napoletano, P., Piccoli, F., and Schettini, R. (2018).
Anomaly detection in nanofibrous materials by cnn-
based self-similarity. Sensors, 18(1):209.
Pang, G., Shen, C., Cao, L., and Hengel, A. V. D. (2021).
Deep learning for anomaly detection: A review. ACM
computing surveys (CSUR), 54(2):1–38.
Pirnay, J. and Chai, K. (2022). Inpainting transformer
for anomaly detection. In International Conference
on Image Analysis and Processing, pages 394–406.
Springer.
Rippel, O. and Merhof, D. (2023). Anomaly detection for
automated visual inspection: A review. Bildverar-
beitung in der Automation: Ausgew
¨
ahlte Beitr
¨
age des
Jahreskolloquiums BVAu 2022, pages 1–13.
Schlegl, T., Seeb
¨
ock, P., Waldstein, S. M., Schmidt-Erfurth,
U., and Langs, G. (2017). Unsupervised anomaly de-
tection with generative adversarial networks to guide
marker discovery. In International conference on in-
formation processing in medical imaging, pages 146–
157. Springer.
Schneider, S., Antensteiner, D., Soukup, D., and Scheutz,
M. (2022). Autoencoders-a comparative analysis in
the realm of anomaly detection. In Proceedings of
the IEEE/CVF Conference on Computer Vision and
Pattern Recognition, pages 1986–1992.
Soille, P. and Soille, P. (2004). Erosion and dilation. Mor-
phological Image Analysis: Principles and Applica-
tions, pages 63–103.
Tan, M. and Le, Q. (2019). Efficientnet: Rethinking model
scaling for convolutional neural networks. In Interna-
tional conference on machine learning, pages 6105–
6114. PMLR.
Tang, T.-W., Kuo, W.-H., Lan, J.-H., Ding, C.-F., Hsu, H.,
and Young, H.-T. (2020). Anomaly detection neural
network with dual auto-encoders gan and its industrial
inspection applications. Sensors, 20(12):3336.
Tsai, D.-M. and Jen, P.-H. (2021). Autoencoder-based
anomaly detection for surface defect inspection. Ad-
vanced Engineering Informatics, 48:101272.
Yang, J., Xu, R., Qi, Z., and Shi, Y. (2021). Visual
anomaly detection for images: A survey. arXiv
preprint arXiv:2109.13157.
Zhou, K., Li, J., Xiao, Y., Yang, J., Cheng, J., Liu, W., Luo,
W., Liu, J., and Gao, S. (2021). Memorizing structure-
texture correspondence for image anomaly detection.
IEEE Transactions on Neural Networks and Learning
Systems, 33(6):2335–2349.
VISAPP 2024 - 19th International Conference on Computer Vision Theory and Applications
200