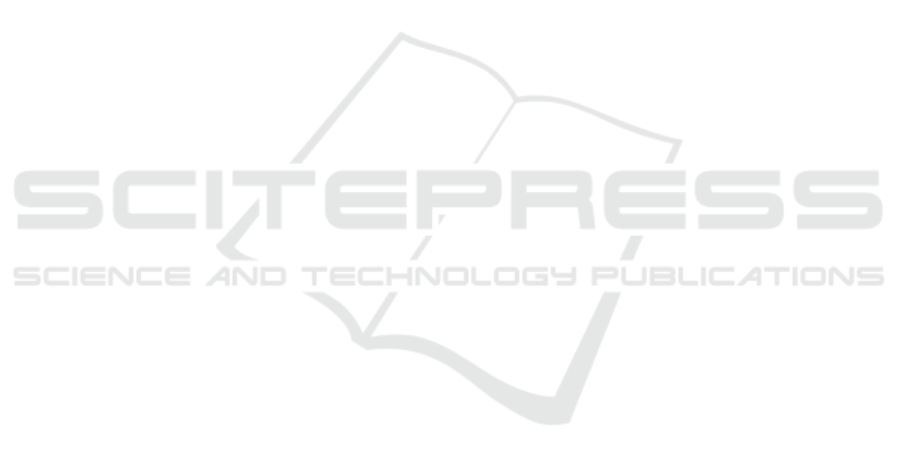
timestamp for digital multimedia via optical sensing
and signal processing. IEEE Transactions on Infor-
mation Forensics and Security, 8(9):1417–1432.
Grigoras, C. (2005). Digital audio recording analysis–the
electric network frequency criterion. International
Journal of Speech Language and the Law, 12(1):63–
76.
Grigoras, C. (2007). Applications of ENF criterion in
forensic audio, video, computer and telecommunica-
tion analysis. Forensic Science International, 167(2-
3):136–145.
Hajj-Ahmad, A. (2016). ENF power frequency data for lo-
cation forensics. https://dx.doi.org/10.21227/H2159S.
Signal Processing Cup.
Hajj-Ahmad, A., Berkovich, A., and Wu, M. (2016). Ex-
ploiting power signatures for camera forensics. IEEE
Signal Processing Letters, 23(5):713–717.
Hajj-Ahmad, A., Garg, R., and Wu, M. (2013). ENF based
location classification of sensor recordings. In Pro-
ceedings of the 2013 IEEE International Workshop on
Information Forensics and Security, pages 138–143.
IEEE.
Hajj-Ahmad, A., Garg, R., and Wu, M. (2015). ENF-based
region-of-recording identification for media signals.
IEEE Transactions on Information Forensics and Se-
curity, 10(6):1125–1136.
Hua, G., Goh, J., and Thing, V. L. (2014). A dynamic
matching algorithm for audio timestamp identification
using the ENF criterion. IEEE Transactions on Infor-
mation Forensics and Security, 9(7):1045–1055.
Jiang, S., Shi, C., and Li, H. (2019). Acoustic scene classi-
fication using ensembles of convolutional neural net-
works and spectrogram decompositions. In Mandel,
M., Salamon, J., and Ellis, D. P. W., editors, Proceed-
ings of the 2019 Challenge on Detection and Classi-
fication of Acoustic Scenes and Events, pages 45–49.
New York University, NY, USA.
Kim, S. and Yoon, J. W. (2020). Location tracking tech-
nique for regional ENF classification using ARIMA.
In Proceedings of the 2020 International Conference
on Information and Communication Technology Con-
vergence, pages 1321–1324. IEEE.
Kingma, D. P. and Ba, J. (2014). Adam: A
method for stochastic optimization. arXiv preprint
arXiv:1412.6980.
Le, L., Kabir, A. N. M., Ji, C., Basodi, S., and Pan, Y.
(2019). Using transfer learning, svm, and ensem-
ble classification to classify baby cries based on their
spectrogram images. In Proceedings of the 2019 IEEE
16th International Conference on Mobile Ad Hoc and
Sensor Systems Workshops, pages 106–110. IEEE.
Mienye, I. D. and Sun, Y. (2022). A survey of ensem-
ble learning: Concepts, algorithms, applications, and
prospects. IEEE Access, 10:99129–99149.
Nanni, L., Costa, Y. M. G., Aguiar, R. L., Mangolin,
R. B., Brahnam, S., and Silla, C. N. (2020). Ensem-
ble of convolutional neural networks to improve ani-
mal audio classification. EURASIP Journal on Audio,
Speech, and Music Processing, 2020(1):1–14.
Ngharamike, E., Ang, K. L.-M., Seng, J. K. P., and Wang,
M. (2023a). ENF based digital multimedia forensics:
Survey, application, challenges and future work. IEEE
Access, 11:101241–101272.
Ngharamike, E., Ang, L.-M., Seng, K. P., and Wang, M.
(2023b). Exploiting the rolling shutter read-out time
for ENF-based camera identification. Applied Sci-
ences, 13(8):5039.
Ohib, R., Arnob, S. Y., Arefin, R., Amin, M., and Reza, T.
(2017). ENF based grid classification system: Identi-
fying the region of origin of digital recordings. Crite-
rion, 3(4):5.
Pedregosa, F., Varoquaux, G., Gramfort, A., Michel, V.,
Thirion, B., Grisel, O., Blondel, M., Prettenhofer, P.,
Weiss, R., Dubourg, V., et al. (2011). Scikit-learn:
Machine learning in Python. The Journal of Machine
Learning Research, 12:2825–2830.
Ristea, N. C. and Ionescu, R. T. (2021). Self-paced ensem-
ble learning for speech and audio classification. arXiv
preprint arXiv:2103.11988.
ˇ
Sari
´
c,
ˇ
Z.,
ˇ
Zuni
´
c, A., Zrni
´
c, T., Kne
ˇ
zevi
´
c, M., Despo-
tovi
´
c, D., and Deli
´
c, T. (2016). Improving location
of recording classification using electric network fre-
quency (ENF) analysis. In Proceedings of the 2016
IEEE International Symposium on Intelligent Systems
and Informatics, pages 51–56. IEEE.
Sarkar, M., Chowdhury, D., Shahnaz, C., and Fattah, S. A.
(2019). Application of electrical network frequency
of digital recordings for location-stamp verification.
Applied Sciences, 9(15):3135.
Triantafyllopoulos, A., Foliadis, A., Roustas, G., Krillis,
I., Athanasiou, F., Papaioannou, M., and Skodras, A.
(2016). Exploring power signatures for location foren-
sics of media recordings. Technical report, University
of Patras, Greece. Signal Processing Cup.
Tsai, S.-J., Zhang, L., Phadke, A. G., Liu, Y., Ingram,
M. R., Bell, S. C., Grant, I. S., Bradshaw, D. T.,
Lubkeman, D., and Tang, L. (2007). Frequency sen-
sitivity and electromechanical propagation simulation
study in large power systems. IEEE Transactions on
Circuits and Systems I: Regular Papers, 54(8):1819–
1828.
Vatansever, S., Dirik, A. E., and Memon, N. (2022). ENF
based robust media time-stamping. IEEE Signal Pro-
cessing Letters, 29:1963–1967.
Wu, M., Hajj-Ahmad, A., Kirchner, M., Ren, Y., Zhang, C.,
and Campisi, P. (2016). Location signatures that you
don’t see: Highlights from the IEEE signal processing
cup 2016 student competition. IEEE Signal Process-
ing Magazine, 33(5):149–156.
Yao, W., Zhao, J., Till, M. J., You, S., Liu, Y., Cui, Y.,
and Liu, Y. (2017). Source location identification of
distribution-level electric network frequency signals at
multiple geographic scales. IEEE Access, 5:11166–
11175.
Zhou, H., Duanmu, H., Li, J., Ma, Y., Shi, J., Tan, Z., Wang,
X., Xiang, L., Yin, H., and Li, W. (2016). Geographic
location estimation from ENF signals with high ac-
curacy. Technical report, University of Science and
Technology of China. Signal Processing Cup.
On Spectrogram Analysis in a Multiple Classifier Fusion Framework for Power Grid Classification Using Electric Network Frequency
99