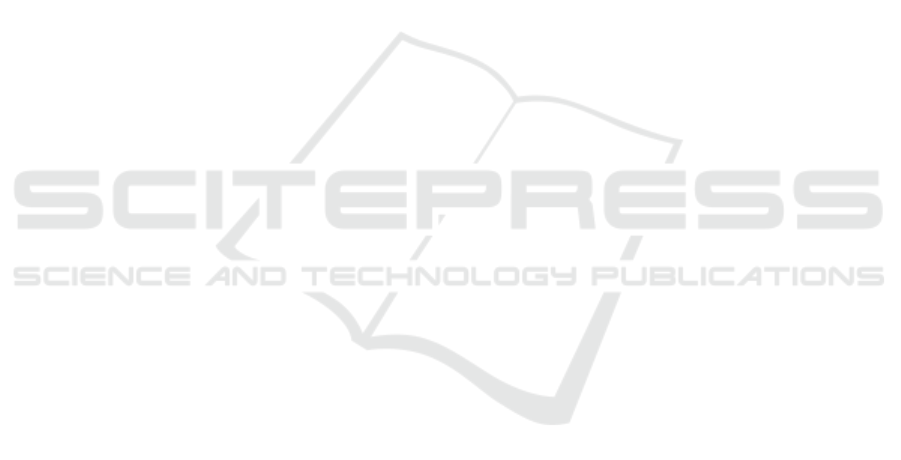
C., editors, Proceedings of the Information Systems
Development: Artificial Intelligence for Information
Systems Development and Operations, Cluj-Napoca,
Romania: Babes
,
-Bolyai University.
Celikyilmaz, A., Clark, E., and Gao, J. (2020). Evaluation
of text generation: A survey. CoRR, abs/2006.14799.
arXiv.
Cer, D., Yang, Y., Kong, S.-y., Hua, N., Limtiaco, N., John,
R. S., Constant, N., Guajardo-Cespedes, M., Yuan,
S., Tar, C., et al. (2018). Universal sentence encoder.
arXiv preprint arXiv:1803.11175.
Fabbri, A. R., Kry
´
sci
´
nski, W., McCann, B., Xiong, C.,
Socher, R., and Radev, D. (2020). Summeval: Re-
evaluating summarization evaluation. Transactions of
the Association for Computational Linguistics, 9:391–
409.
Forgues, G., Pineau, J., Larchev
ˆ
eque, J.-M., and Trem-
blay, R. (2014). Bootstrapping dialog systems with
word embeddings. In Nips, modern machine learning
and natural language processing workshop, volume 2,
page 168.
Gardner, R. L., Cooper, E., Haskell, J., Harris, D. A.,
Poplau, S., Kroth, P. J., and Linzer, M. (2018). Physi-
cian stress and burnout: the impact of health infor-
mation technology. Journal of the American Medical
Informatics Association, 26(2):106–114.
Goodrich, B., Rao, V., Liu, P. J., and Saleh, M. (2019). As-
sessing the factual accuracy of generated text. Pro-
ceedings of the ACM SIGKDD International Con-
ference on Knowledge Discovery and Data Mining,
pages 166–175.
Hauer, A., Waukau, H., and Welch, P. (2018). Physician
burnout in wisconsin: An alarming trend affecting
physician wellness. Wmj, 117(5):194–200.
Heuer, A. J. (2022). More evidence that the healthcare ad-
ministrative burden is real, widespread and has serious
consequences comment on” perceived burden due to
registrations for quality monitoring and improvement
in hospitals: A mixed methods study”. International
Journal of Health Policy and Management, 11(4):536.
Heun, L., Brandau, D. T., Chi, X., Wang, P., and Kangas, J.
(1998). Validation of computer-mediated open-ended
standardized patient assessments. International Jour-
nal of Medical Informatics, 50(1):235–241.
Houwen, J., Lucassen, P. L., Stappers, H. W., Assendelft,
W. J., van Dulmen, S., and Olde Hartman, T. C.
(2017). Improving gp communication in consultations
on medically unexplained symptoms: a qualitative in-
terview study with patients in primary care. British
Journal of General Practice, 67(663):e716–e723.
Kiros, R., Zhu, Y., Salakhutdinov, R. R., Zemel, R., Ur-
tasun, R., Torralba, A., and Fidler, S. (2015). Skip-
thought vectors. Advances in neural information pro-
cessing systems, 28.
Kry
´
sci
´
nski, W., Keskar, N. S., McCann, B., Xiong, C., and
Socher, R. (2019). Neural text summarization: A criti-
cal evaluation. In Proceedings of the 2019 Conference
on Empirical Methods in Natural Language Process-
ing and the 9th International Joint Conference on Nat-
ural Language Processing (EMNLP-IJCNLP), pages
540–551.
Kusner, M., Sun, Y., Kolkin, N., and Weinberger, K. (2015).
From word embeddings to document distances. In
International conference on machine learning, pages
957–966. PMLR.
Kwint, E., Zoet, A., Labunets, K., and Brinkkemper, S.
(2023). How different elements of audio affect the
word error rate of transcripts in automated medical re-
porting. Proceedings of BIOSTEC, 5:179–187.
Lavander, P., Meril
¨
ainen, M., and Turkki, L. (2016). Work-
ing time use and division of labour among nurses and
health-care workers in hospitals–a systematic review.
Journal of Nursing Management, 24(8):1027–1040.
Levenshtein, V. I. et al. (1966). Binary codes capable of cor-
recting deletions, insertions, and reversals. In Soviet
physics doklady, volume 10, pages 707–710. Soviet
Union.
Lin, C.-Y. (2004). Rouge: A package for automatic evalu-
ation of summaries. In Text summarization branches
out, pages 74–81.
Maas, L., Geurtsen, M., Nouwt, F., Schouten, S.,
Van De Water, R., Van Dulmen, S., Dalpiaz, F.,
Van Deemter, K., and Brinkkemper, S. (2020). The
care2report system: Automated medical reporting as
an integrated solution to reduce administrative burden
in healthcare. In HICSS, pages 1–10.
Maroengsit, W., Piyakulpinyo, T., Phonyiam, K.,
Pongnumkul, S., Chaovalit, P., and Theeramunkong,
T. (2019). A survey on evaluation methods for chat-
bots. In Proceedings of the 2019 7th International
conference on information and education technology,
pages 111–119.
Meijers, M. C., Noordman, J., Spreeuwenberg, P.,
Olde Hartman, T. C., and van Dulmen, S. (2019).
Shared decision-making in general practice: an ob-
servational study comparing 2007 with 2015. Family
practice, 36(3):357–364.
Molenaar, S., Maas, L., Burriel, V., Dalpiaz, F., and
Brinkkemper, S. (2020). Medical dialogue summa-
rization for automated reporting in healthcare. In
Dupuy-Chessa, S. and Proper, H. A., editors, Ad-
vanced Information Systems Engineering Workshops,
pages 76–88, Cham. Springer International Publish-
ing.
Moramarco, F., Korfiatis, A. P., Perera, M., Juric, D., Flann,
J., Reiter, E., Savkov, A., and Belz, A. (2022). Hu-
man evaluation and correlation with automatic metrics
in consultation note generation. In ACL 2022: 60th
Annual Meeting of the Association for Computational
Linguistics, pages 5739–5754. Association for Com-
putational Linguistics.
Morris, A. C., Maier, V., and Green, P. (2004). From wer
and ril to mer and wil: improved evaluation measures
for connected speech recognition. In Eighth Interna-
tional Conference on Spoken Language Processing.
Moy, A. J., Schwartz, J. M., Chen, R., Sadri, S., Lucas, E.,
Cato, K. D., and Rossetti, S. C. (2021). Measurement
of clinical documentation burden among physicians
and nurses using electronic health records: a scoping
review. Journal of the American Medical Informatics
Association, 28(5):998–1008.
Ng, J. P. and Abrecht, V. (2015). Better summarization eval-
uation with word embeddings for rouge. In Proceed-
Comparative Experimentation of Accuracy Metrics in Automated Medical Reporting: The Case of Otitis Consultations
593