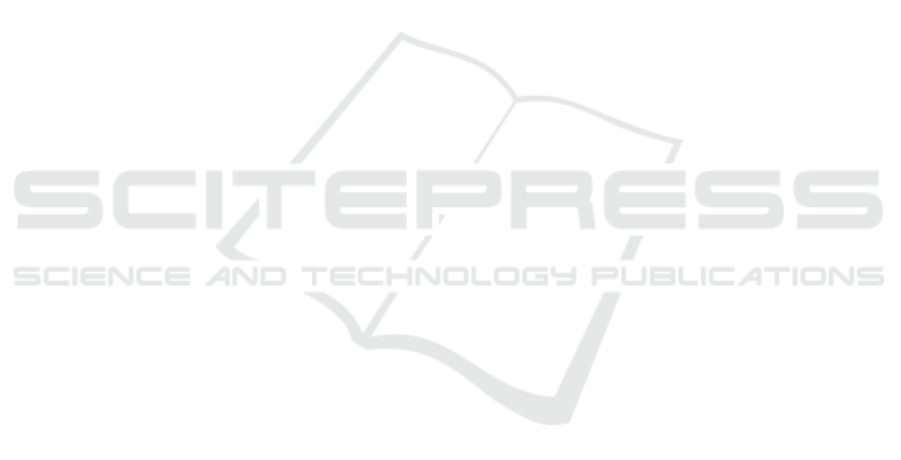
sessment results in the experiments (section 5).
Finding duplicates in all examined datasets does
indicate that such accidental inclusion of duplicates
could be a common occurrence for web-scraped face
image datasets, so that any potential future dataset
construction of this kind should consider implement-
ing a duplicate filter. It may further be sensible to
examine other existing web-scraped datasets, as they
could likewise contain duplicates.
ACKNOWLEDGEMENTS
This research work has been funded by the German
Federal Ministry of Education and Research and the
Hessian Ministry of Higher Education, Research, Sci-
ence and the Arts within their joint support of the
National Research Center for Applied Cybersecurity
ATHENE. This project has received funding from the
European Union’s Horizon 2020 research and inno-
vation programme under grant agreement No 883356.
This text reflects only the author’s views and the Com-
mission is not liable for any use that may be made of
the information contained therein.
REFERENCES
Boutros, F., Fang, M., Klemt, M., Fu, B., and Damer, N.
(2023). CR-FIQA: Face image quality assessment
by learning sample relative classifiability. In Conf.
on Computer Vision and Pattern Recognition (CVPR),
pages 5836–5845. IEEE.
Cheng, Z., Zhu, X., and Gong, S. (2018). Low-resolution
face recognition. In Asian Conf. on Computer Vision
(ACCV).
Deng, J., Guo, J., and Zafeiriou, S. (2019). ArcFace: Ad-
ditive angular margin loss for deep face recognition.
In Conf. on Computer Vision and Pattern Recognition
(CVPR).
Eidinger, E., Enbar, R., and Hassner, T. (2014). Age and
gender estimation of unfiltered faces. IEEE Trans.
on Information Forensics and Security, 9(12):2170–
2179.
Grother, P. and Tabassi, E. (2007). Performance of biomet-
ric quality measures. IEEE Trans. on Pattern Analysis
and Machine Intelligence, 29(4):531–543.
Guo, J., Deng, J., Lattas, A., and Zafeiriou, S. (2022). Sam-
ple and computation redistribution for efficient face
detection. In Intl. Conf. on Learning Representations
(ICLR).
Guo, Y., Zhang, L., Hu, Y., He, X., and Gao, J. (2016). MS-
Celeb-1M: A dataset and benchmark for large-scale
face recognition. Proc. 14th European Conf. on Com-
puter Vision.
Huang, G. B., Ramesh, M., Berg, T., and Learned-Miller,
E. (2007). Labeled faces in the wild: A database for
studying face recognition in unconstrained environ-
ments. Technical report, University of Massachusetts,
Amherst.
ISO/IEC JTC1 SC37 Biometrics (2022). ISO/IEC 2382-
37:2022 Information technology - Vocabulary - Part
37: Biometrics. International Organization for Stan-
dardization.
Jazaery, M. A. and Guo, G. (2019). Automated cleaning of
identity label noise in a large face dataset with quality
control. IET Biometrics, 9(1):25–30.
Jin, C., Jin, R., Chen, K., and Dou, Y. (2018). A com-
munity detection approach to cleaning extremely large
face database. Computational intelligence and neuro-
science.
Meng, Q., Zhao, S., Huang, Z., and Zhou, F. (2021). Mag-
Face: A universal representation for face recognition
and quality assessment. In Conf. on Computer Vi-
sion and Pattern Recognition (CVPR), pages 14225–
14234. IEEE.
O’Connor, J., Aumasson, J., Neves, S., and Wilcox-
O’Hearn, Z. (2021). BLAKE3. https://github.com
/BLAKE3-team/BLAKE3/.
Schlett, T., Rathgeb, C., Tapia, J., and Busch, C. (2023).
Considerations on the evaluation of biometric quality
assessment algorithms. IEEE Trans. on Biometrics,
Behavior, and Identity Science (TBIOM).
Yi, D., Lei, Z., Liao, S., and Li, S. Z. (2014). Learning face
representation from scratch.
Zhang, X., Zhang, L., Wang, X.-J., and Shum, H.-Y. (2012).
Finding celebrities in billions of web images. IEEE
Trans. on Multimedia, 14(4):995–1007.
ICPRAM 2024 - 13th International Conference on Pattern Recognition Applications and Methods
808