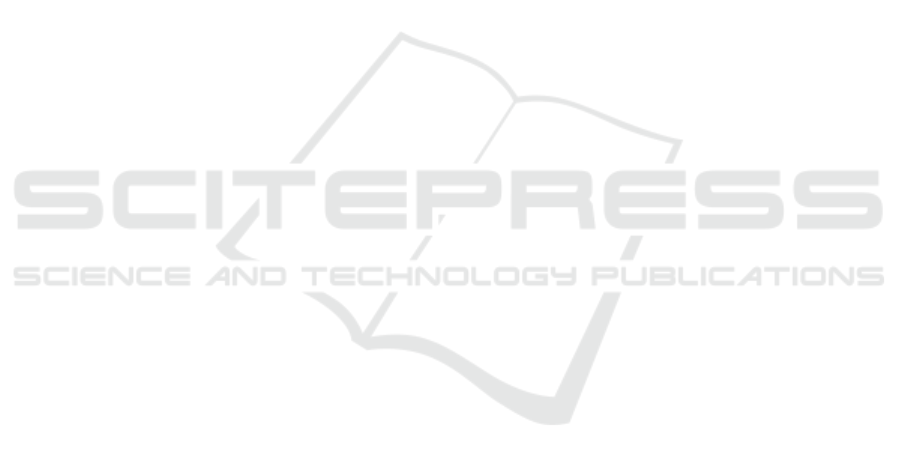
Krishna, K., Khosla, S., Bigham, J. P., and Lipton,
Z. C. (2021). Generating soap notes from doctor-
patient conversations using modular summarization
techniques. In Proceedings of the 59th Annual Meet-
ing of the Association for Computational Linguistics
and the 11th International Joint Conference on Natu-
ral Language Processing, pages 4958–4972.
Lin, C.-Y. (2004). ROUGE: A package for automatic evalu-
ation of summaries. In Text Summarization Branches
Out, pages 74–81, Barcelona, Spain. Association for
Computational Linguistics.
Liu, P., Yuan, W., Fu, J., Jiang, Z., Hayashi, H., and Neubig,
G. (2023a). Pre-train, prompt, and predict: A system-
atic survey of prompting methods in natural language
processing. ACM Computing Surveys, 55(9):1–35.
Liu, Y., Iter, D., Xu, Y., Wang, S., Xu, R., and Zhu,
C. (2023b). Gpteval: Nlg evaluation using gpt-
4 with better human alignment. arXiv preprint
arXiv:2303.16634.
Maas, L., Geurtsen, M., Nouwt, F., Schouten, S.,
Van De Water, R., Van Dulmen, S., Dalpiaz, F.,
Van Deemter, K., and Brinkkemper, S. (2020). The
care2report system: Automated medical reporting as
an integrated solution to reduce administrative burden
in healthcare. In HICSS, pages 1–10.
Mathur, Y., Rangreji, S., Kapoor, R., Palavalli, M., Bertsch,
A., and Gormley, M. (2023). Summqa at mediqa-chat
2023: In-context learning with gpt-4 for medical sum-
marization. In The 61st Annual Meeting Of The Asso-
ciation For Computational Linguistics.
Meijers, M. C., Noordman, J., Spreeuwenberg, P.,
Olde Hartman, T. C., and van Dulmen, S. (2019).
Shared decision-making in general practice: an ob-
servational study comparing 2007 with 2015. Family
practice, 36(3):357–364.
Michalopoulos, G., Williams, K., Singh, G., and Lin, T.
(2022). Medicalsum: A guided clinical abstractive
summarization model for generating medical reports
from patient-doctor conversations. In Findings of the
Association for Computational Linguistics: EMNLP
2022, pages 4741–4749.
Mickey, N. (2023). Explore the benefits of azure openai
service with microsoft learn: Azure blog: Microsoft
azure. Azure. https://tinyurl.com/azure-openai.
Moramarco, F., Korfiatis, A. P., Perera, M., Juric, D., Flann,
J., Reiter, E., Savkov, A., and Belz, A. (2022). Hu-
man evaluation and correlation with automatic metrics
in consultation note generation. In ACL 2022: 60th
Annual Meeting of the Association for Computational
Linguistics, pages 5739–5754. Association for Com-
putational Linguistics.
Mridha, M. F., Lima, A. A., Nur, K., Das, S. C., Hasan,
M., and Kabir, M. M. (2021). A survey of automatic
text summarization: Progress, process and challenges.
IEEE Access, 9:156043–156070.
Nair, V., Schumacher, E., and Kannan, A. (2023). Generat-
ing medically-accurate summaries of patient-provider
dialogue: A multi-stage approach using large lan-
guage models. arXiv preprint arXiv:2305.05982.
Overhage, J. M. and McCallie Jr, D. (2020). Physician time
spent using the electronic health record during outpa-
tient encounters: a descriptive study. Annals of inter-
nal medicine, 172(3):169–174.
Radford, A., Wu, J., Child, R., Luan, D., Amodei, D.,
Sutskever, I., et al. (2019). Language models are un-
supervised multitask learners. OpenAI blog, 1(8):9.
Reynolds, L. and McDonell, K. (2021). Prompt program-
ming for large language models: Beyond the few-shot
paradigm. In Extended Abstracts of the 2021 CHI
Conference on Human Factors in Computing Systems,
pages 1–7.
Robinson, R. (2023). How to write an effective gpt-3 or
gpt-4 prompt. Zapier. https://tinyurl.com/gpt-prompt.
Savkov, A., Moramarco, F., Korfiatis, A. P., Perera, M.,
Belz, A., and Reiter, E. (2022). Consultation check-
lists: Standardising the human evaluation of medical
note generation. In Proceedings of the 2022 Confer-
ence on Empirical Methods in Natural Language Pro-
cessing: Industry Track, pages 111–120.
Schenker, J. D. and Rumrill Jr, P. D. (2004). Causal-
comparative research designs. Journal of vocational
rehabilitation, 21(3):117–121.
Tam, A. (2023). What are zero-shot prompting and
few-shot prompting. Machine Learning Mastery.
https://tinyurl.com/machine-learning-mastery.
Tangsali, R., Vyawahare, A. J., Mandke, A. V., Litake,
O. R., and Kadam, D. D. (2022). Abstractive ap-
proaches to multidocument summarization of medical
literature reviews. In Proceedings of the Third Work-
shop on Scholarly Document Processing, pages 199–
203.
van Buchem, M. M., Boosman, H., Bauer, M. P., Kant,
I. M., Cammel, S. A., and Steyerberg, E. W. (2021).
The digital scribe in clinical practice: a scoping re-
view and research agenda. NPJ digital medicine,
4(1):57.
Wang, J., Shi, E., Yu, S., Wu, Z., Ma, C., Dai, H., Yang,
Q., Kang, Y., Wu, J., Hu, H., et al. (2023). Prompt
engineering for healthcare: Methodologies and appli-
cations. arXiv preprint arXiv:2304.14670.
White, J., Fu, Q., Hays, S., Sandborn, M., Olea, C., Gilbert,
H., Elnashar, A., Spencer-Smith, J., and Schmidt,
D. C. (2023). A prompt pattern catalog to enhance
prompt engineering with chatgpt. arXiv preprint
arXiv:2302.11382.
Widyassari, A. P., Affandy, A., Noersasongko, E., Fanani,
A. Z., Syukur, A., and Basuki, R. S. (2019). Litera-
ture review of automatic text summarization: research
trend, dataset and method. In 2019 International Con-
ference on Information and Communications Technol-
ogy (ICOIACT), pages 491–496. IEEE.
Yadav, D., Desai, J., and Yadav, A. K. (2022). Automatic
text summarization methods: A comprehensive re-
view. arXiv preprint arXiv:2204.01849.
Ye, X. and Durrett, G. (2022). The unreliability of expla-
nations in few-shot prompting for textual reasoning.
Advances in neural information processing systems,
35:30378–30392.
Zhao, Z., Wallace, E., Feng, S., Klein, D., and Singh, S.
(2021). Calibrate before use: Improving few-shot per-
formance of language models. In International Con-
ference on Machine Learning, pages 12697–12706.
PMLR.
Enhancing Summarization Performance Through Transformer-Based Prompt Engineering in Automated Medical Reporting
165