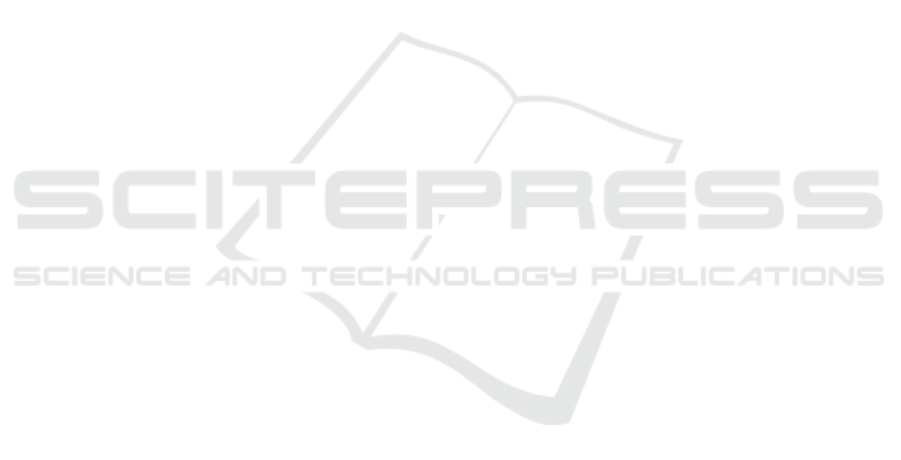
Dosovitskiy, A., Beyer, L., Kolesnikov, A., Weissenborn,
D., Zhai, X., Unterthiner, T., Dehghani, M., Minderer,
M., Heigold, G., Gelly, S., Uszkoreit, J., and Houlsby,
N. (2021). An image is worth 16x16 words: Trans-
formers for image recognition at scale. In 9th Interna-
tional Conference on Learning Representations, ICLR
2021, Virtual Event, Austria, May 3-7, 2021. OpenRe-
view.net.
Droste, R., Jiao, J., and Noble, J. A. (2020). Unified image
and video saliency modeling. In Computer Vision–
ECCV 2020: 16th European Conference, Glasgow,
UK, August 23–28, 2020, Proceedings, Part V 16,
pages 419–435. Springer.
Fang, Y., Zhang, C., Min, X., Huang, H., Yi, Y., Zhai, G.,
and Lin, C.-W. (2020). Devsnet: deep video saliency
network using short-term and long-term cues. Pattern
Recognition, 103:107294.
Jain, S., Yarlagadda, P., Jyoti, S., Karthik, S., Subramanian,
R., and Gandhi, V. (2021). Vinet: Pushing the limits of
visual modality for audio-visual saliency prediction.
In 2021 IEEE/RSJ International Conference on Intel-
ligent Robots and Systems (IROS), pages 3520–3527.
IEEE.
Kay, W., Carreira, J., Simonyan, K., Zhang, B., Hillier, C.,
Vijayanarasimhan, S., Viola, F., Green, T., Back, T.,
Natsev, P., Suleyman, M., and Zisserman, A. (2017).
The kinetics human action video dataset. CoRR,
abs/1705.06950.
Kingma, D. P. and Ba, J. (2014). Adam: A
method for stochastic optimization. arXiv preprint
arXiv:1412.6980.
Kopuklu, O., Kose, N., Gunduz, A., and Rigoll, G. (2019).
Resource efficient 3d convolutional neural networks.
In Proceedings of the IEEE/CVF international con-
ference on computer vision workshops, pages 0–0.
Lai, Q., Wang, W., Sun, H., and Shen, J. (2019). Video
saliency prediction using spatiotemporal residual at-
tentive networks. IEEE Transactions on Image Pro-
cessing, 29:1113–1126.
Li, K., Wang, Y., Zhang, J., Gao, P., Song, G., Liu, Y., Li,
H., and Qiao, Y. (2023). Uniformer: Unifying convo-
lution and self-attention for visual recognition. IEEE
Transactions on Pattern Analysis and Machine Intel-
ligence.
Linardos, P., Mohedano, E., Nieto, J. J., O’Connor, N. E.,
Giro-i Nieto, X., and McGuinness, K. (2019). Simple
vs complex temporal recurrences for video saliency
prediction. arXiv preprint arXiv:1907.01869.
Liu, Z., Ning, J., Cao, Y., Wei, Y., Zhang, Z., Lin, S., and
Hu, H. (2022). Video swin transformer. In Proceed-
ings of the IEEE/CVF conference on computer vision
and pattern recognition, pages 3202–3211.
Lyudvichenko, V., Erofeev, M., Gitman, Y., and Vatolin, D.
(2017). A semiautomatic saliency model and its appli-
cation to video compression. In 2017 13th IEEE In-
ternational Conference on Intelligent Computer Com-
munication and Processing (ICCP), pages 403–410.
IEEE.
Ma, C., Sun, H., Rao, Y., Zhou, J., and Lu, J. (2022). Video
saliency forecasting transformer. IEEE Trans. Circuits
Syst. Video Technol., 32(10):6850–6862.
Mathe, S. and Sminchisescu, C. (2014). Actions in the eye:
Dynamic gaze datasets and learnt saliency models for
visual recognition. IEEE transactions on pattern anal-
ysis and machine intelligence, 37(7):1408–1424.
Min, K. and Corso, J. J. (2019). Tased-net: Temporally-
aggregating spatial encoder-decoder network for
video saliency detection. In Proceedings of the
IEEE/CVF International Conference on Computer Vi-
sion, pages 2394–2403.
Wang, W., Shen, J., Xie, J., Cheng, M.-M., Ling, H., and
Borji, A. (2019). Revisiting video saliency prediction
in the deep learning era. IEEE transactions on pattern
analysis and machine intelligence, 43(1):220–237.
Wang, Z., Liu, Z., Li, G., Wang, Y., Zhang, T., Xu, L., and
Wang, J. (2021). Spatio-temporal self-attention net-
work for video saliency prediction. IEEE Transac-
tions on Multimedia.
Wu, X., Wu, Z., Zhang, J., Ju, L., and Wang, S. (2020).
Salsac: A video saliency prediction model with shuf-
fled attentions and correlation-based convlstm. In The
Thirty-Fourth AAAI Conference on Artificial Intelli-
gence, AAAI 2020, The Thirty-Second Innovative Ap-
plications of Artificial Intelligence Conference, IAAI
2020, The Tenth AAAI Symposium on Educational
Advances in Artificial Intelligence, EAAI 2020, New
York, NY, USA, February 7-12, 2020, pages 12410–
12417. AAAI Press.
Xie, S., Sun, C., Huang, J., Tu, Z., and Murphy, K. (2018).
Rethinking spatiotemporal feature learning: Speed-
accuracy trade-offs in video classification. In Pro-
ceedings of the European conference on computer vi-
sion (ECCV), pages 305–321.
Xiong, J., Zhang, P., Li, C., Huang, W., Zha, Y., and You, T.
(2023). Unist: Towards unifying saliency transformer
for video saliency prediction and detection. CoRR,
abs/2309.08220.
Zhang, K., Chen, Z., and Liu, S. (2020). A spatial-temporal
recurrent neural network for video saliency prediction.
IEEE Transactions on Image Processing, 30:572–587.
Zhang, Y., Zhang, T., Wu, C., and Tao, R. (2023). Multi-
scale spatiotemporal feature fusion network for video
saliency prediction. IEEE Transactions on Multime-
dia.
Zhou, X., Wu, S., Shi, R., Zheng, B., Wang, S., Yin,
H., Zhang, J., and Yan, C. (2023). Transformer-
based multi-scale feature integration network for
video saliency prediction. IEEE Transactions on Cir-
cuits and Systems for Video Technology.
Transformer-Based Video Saliency Prediction with High Temporal Dimension Decoding
623