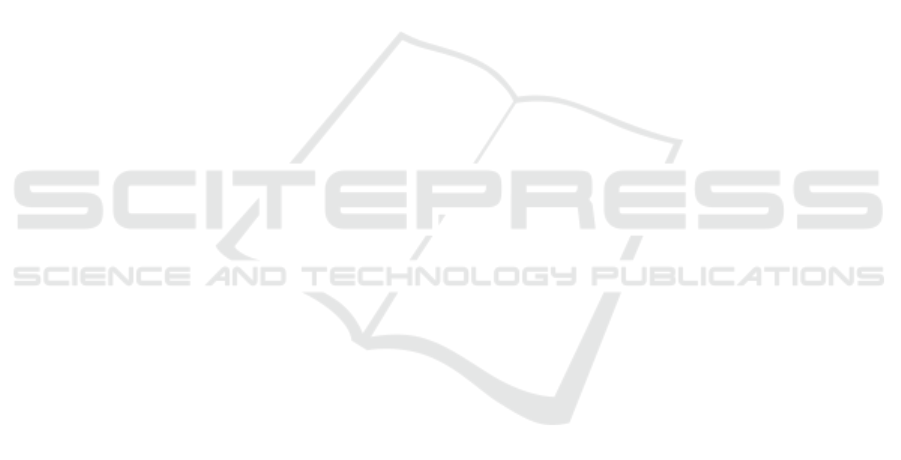
5 CONCLUSION
Our study indicates that both spatial and tempo-
ral down-sampling generally lead to reduced perfor-
mance, though spatial down-sampling shows some
dataset-dependent improvements. Additionally, the
effectiveness of buffer size varies with the duration of
action and there is no optimal global value for that.
For pre-training, we did not observe improvements
from conventional self-supervision methods construc-
tion contexts. Finally, our results highlight the poten-
tial of frame-based approaches for future investiga-
tion of action recognition.
ACKNOWLEDGEMENTS
This research is part of the BoB project, an ICON
project cofunded by Flanders Innovation & En-
trepreneurship (VLAIO) and imec, with project no.
HBC.2021.0658. The data of the construction site
was provided by Willemen Groep, a Belgian con-
struction group, with support from AICON inc.
REFERENCES
Carreira, J. and Zisserman, A. (2017). Quo vadis, action
recognition? a new model and the kinetics dataset. In
CVPR, pages 6299–6308.
Chen, L., Ma, N., Wang, P., Li, J., Wang, P., Pang, G., and
Shi, X. (2020). Survey of pedestrian action recog-
nition techniques for autonomous driving. Tsinghua
Science and Technology, 25(4):458–470.
Fathi, A. and Mori, G. (2008). Action recognition by learn-
ing mid-level motion features. In CVPR, pages 1–8.
Feichtenhofer, C., Fan, H., Malik, J., and He, K. (2019).
Slowfast networks for video recognition. In ICCV.
Feichtenhofer, C., Pinz, A., and Wildes, R. P. (2017).
Spatiotemporal multiplier networks for video action
recognition. In CVPR.
Girshick, R., Donahue, J., Darrell, T., and Malik, J. (2014).
Rich feature hierarchies for accurate object detection
and semantic segmentation. In CVPR, pages 580–587.
Huang, Z., Wan, C., Probst, T., and Van Gool, L. (2017).
Deep learning on lie groups for skeleton-based action
recognition. In CVPR, pages 6099–6108.
Ishioka, H., Weng, X., Man, Y., and Kitani, K. Single cam-
era worker detection, tracking and action recognition
in con-struction site. In ISARC.
Jhuang, H., Gall, J., Zuffi, S., Schmid, C., and Black, M. J.
(2013). Towards understanding action recognition. In
ICCV, pages 3192–3199.
Kar, A. and Prabhakaran, B. (2017). A convnet-based archi-
tecture for semantic labeling of 3d lidar point clouds.
In IROS. IEEE.
K
¨
op
¨
ukl
¨
u, O., Wei, X., and Rigoll, G. (2019). You only
watch once: A unified cnn architecture for real-time
spatiotemporal action localization. arXiv:1911.06644.
Li, Z. and Li, D. (2022). Action recognition of construction
workers under occlusion. Journal of Building Engi-
neering, 45:103352.
Liu, W., Anguelov, D., Erhan, D., Szegedy, C., Reed, S.,
Fu, C.-Y., and Berg, A. C. (2016). Ssd: Single shot
multibox detector. In ECCV.
Noor, N. and Park, I. K. (2023). A lightweight skeleton-
based 3d-cnn for real-time fall detection and action
recognition. In IEEE/CVF, pages 2179–2188.
Qiu, Z., Yao, T., and Mei, T. (2017). Learning spatio-
temporal representation with pseudo-3d residual net-
works. In ICCV, pages 5533–5541.
Ramezani, M. and Yaghmaee, F. (2016). A review on hu-
man action analysis in videos for retrieval applica-
tions. Artificial Intelligence Review, 46:485–514.
Redmon, J., Divvala, S., Girshick, R., and Farhadi, A.
(2016). You only look once: Unified, real-time ob-
ject detection. In CVPR.
Reis, D., Kupec, J., Hong, J., and Daoudi, A.
(2023). Real-time flying object detection with yolov8.
arXiv:2305.09972.
Roberts, D. and Golparvar-Fard, M. (2019). End-to-end
vision-based detection, tracking and activity analysis
of earthmoving equipment filmed at ground level. Au-
tomation in Construction, 105:102811.
Rodomagoulakis, I., Kardaris, N., Pitsikalis, V., Mavroudi,
E., Katsamanis, A., Tsiami, A., and Maragos, P.
(2016). Multimodal human action recognition in as-
sistive human-robot interaction. In ICASSP.
Si, C., Chen, W., Wang, W., Wang, L., and Tan, T. (2019).
Skeleton-based action recognition with spatial reason-
ing and temporal stack learning. In CVPR.
Simonyan, K. and Zisserman, A. (2014). Two-stream con-
volutional networks for action recognition in videos.
NIPS, 27.
Tran, D., Bourdev, L., Fergus, R., Torresani, L., and Paluri,
M. (2015). Learning spatiotemporal features with 3d
convolutional networks. In ICCV, pages 4489–4497.
Tran, D., Wang, H., Torresani, L., Ray, J., LeCun, Y., and
Paluri, M. (2018). A closer look at spatiotemporal
convolutions for action recognition. In CVPR.
Wang, H. and Schmid, C. (2013). Action recognition with
improved trajectories. In PICCV, pages 3551–3558.
Wang, Z., Liu, Y., Duan, S., and Pan, H. (2023). An ef-
ficient detection of non-standard miner behavior us-
ing improved yolov8. Computers and Electrical En-
gineering, 112:109021.
Weinzaepfel, P., Harchaoui, Z., and Schmid, C. (2015).
Learning to track for spatio-temporal action localiza-
tion. In ICCV, pages 3164–3172.
Yan, S., Xiong, Y., and Lin, D. (2018). Spatial temporal
graph convolutional networks for skeleton-based ac-
tion recognition. In AAAI.
Zhang, D., He, L., Tu, Z., Zhang, S., Han, F., and Yang, B.
(2020). Learning motion representation for real-time
spatio-temporal action localization. Pattern Recogni-
tion, 103:107312.
VISAPP 2024 - 19th International Conference on Computer Vision Theory and Applications
600