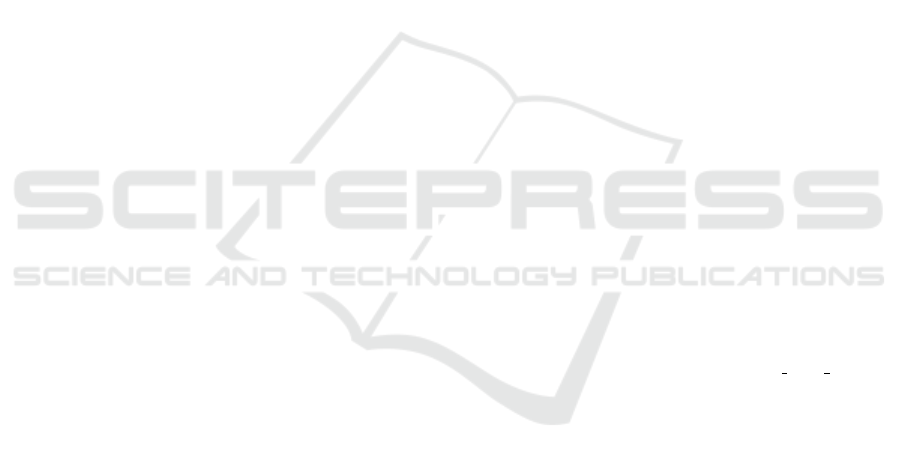
ACKNOWLEDGEMENTS
This work was funded by the Lower Saxony Ministry
of Science and Culture under grant number 11-76251-
12-10/19 ZN3491 within the Lower Saxony “Vorab”
of the Volkswagen Foundation and supported by the
Center for Digital Innovations (ZDIN). We would like
to thank Linda B
¨
uker and Lea Ortmann for their valu-
able input and feedback.
REFERENCES
Ackley, B. J. and Ladwig, G. B. (2010). Nursing diagnosis
handbook-e-book: An evidence-based guide to plan-
ning care. Elsevier Health Sciences.
AI-generated Videos (2023). https://cloud.uol.de/s/bKcKG
y3QKG7LdEm.
Aiken, L. H., Sermeus, W., Van den Heede, K., Sloane,
D. M., Busse, R., McKee, M., Bruyneel, L., Raf-
ferty, A. M., Griffiths, P., Moreno-Casbas, M. T., et al.
(2012). Patient safety, satisfaction, and quality of hos-
pital care: cross sectional surveys of nurses and pa-
tients in 12 countries in europe and the united states.
BMJ, 344:e1717.
Bernhart-Just, A., Lassen, B., and Schwendimann, R.
(2010). Representing the nursing process with nursing
terminologies in electronic medical record systems: a
swiss approach. CIN: Computers, Informatics, Nurs-
ing, 28(6):345–352.
Brinkmann, A., B
¨
ohlen, C. F.-v., Kowalski, C., Lau, S.,
Meyer, O., Diekmann, R., and Hein, A. (2022).
Providing physical relief for nurses by collaborative
robotics. Scientific Reports, 12(1):8644.
Buchanan, C., Howitt, M. L., Wilson, R., Booth, R. G., Ris-
ling, T., and Bamford, M. (2020). Predicted influences
of artificial intelligence on the domains of nursing:
scoping review. JMIR nursing, 3(1):e23939.
Bulechek, G. M., Butcher, H. K., Dochterman, J. M. M.,
and Wagner, C. (2012). Nursing interventions classi-
fication (NIC). Elsevier Health Sciences.
Carroll, W. M. et al. (2020). Emerging technologies for
nurses: Implications for practice. Springer Publishing
Company.
Doenges, M. E. and Moorhouse, M. F. (2012). Application
of nursing process and nursing diagnosis: an interac-
tive text for diagnostic reasoning. FA Davis.
Feldblum, I., German, L., Bilenko, N., Shahar, A., Enten,
R., Greenberg, D., Harman, I., Castel, H., and Sha-
har, D. R. (2009). Nutritional risk and health care use
before and after an acute hospitalization among the el-
derly. Nutrition, 25(4):415–420.
Hellmers, S. (2021). Technikgest
¨
utztes klinisches Assess-
ment der k
¨
orperlichen Funktionalit
¨
at f
¨
ur
¨
altere Men-
schen. Verlag Dr. Hut.
Herdman, T. H., Kamitsuru, S., and Lopes, C. (2021).
NANDA International Nursing Diagnoses: Defini-
tions and Classification 2021-2023. New York:
Thieme, 12th edition.
H
¨
ohmann, U., Lautenschl
¨
ager, M., and Schwarz, L. (2016).
Belastungen im pflegeberuf: Bedingungsfaktoren, fol-
gen und desiderate. Pflege-Report, pages 73–89.
Hojdelewicz, B. M. (2021). Der Pflegeprozess:
Prozesshafte Pflegebeziehung. Wien: facultas.
Lancet Regional Health-Europe, T. (2023). Embracing gen-
erative ai in health care. The Lancet Regional Health-
Europe, 30.
Lugaresi, C., Tang, J., Nash, H., McClanahan, C., Uboweja,
E., Hays, M., Zhang, F., Chang, C.-L., Yong, M., Lee,
J., et al. (2019). Mediapipe: A framework for per-
ceiving and processing reality. In Third workshop on
computer vision for AR/VR at IEEE computer vision
and pattern recognition (CVPR), volume 2019.
Lunney, M. (2006). Helping nurses use nanda, noc, and
nic: Novice to expert. JONA: The Journal of Nursing
Administration, 36(3):118–125.
Martinez-Ortigosa, A., Martinez-Granados, A., Gil-
Hern
´
andez, E., Rodriguez-Arrastia, M., Ropero-
Padilla, C., Roman, P., et al. (2023). Applications of
artificial intelligence in nursing care: A systematic re-
view. Journal of Nursing Management, 2023.
Moorhead, S., Swanson, E., and Johnson, M. (2023). Nurs-
ing Outcomes Classification (NOC)-e-book: Mea-
surement of health outcomes. Elsevier Health Sci-
ences.
Poppe, R. (2010). A survey on vision-based human action
recognition. Image and vision computing, 28(6):976–
990.
RunwayML (2023). https://runwayml.com. accessed:
2023-11-15.
Sensmeier, J. (2017). Harnessing the power of artificial in-
telligence. Nursing management, 48(11):14–19.
Statistisches Bundesamt (Destatis) (2023). Pflegevoraus-
berechnung: 1,8 millionen mehr pflegebed
¨
urftige bis
zum jahr 2055 zu erwarten. Pressemitteilung Nr. 124
vom 30. M
¨
arz 2023, https://www.destatis.de/DE/Press
e/Pressemitteilungen/2023/03/PD23\ 124\ 12.html.
Volkert, D., Beck, A. M., Cederholm, T., Cruz-Jentoft, A.,
Hooper, L., Kiesswetter, E., Maggio, M., Raynaud-
Simon, A., Sieber, C., Sobotka, L., et al. (2022). Es-
pen practical guideline: Clinical nutrition and hydra-
tion in geriatrics. Clinical Nutrition, 41(4):958–989.
Wilkinson, J. M. (2014). Pearson nursing diagnosis hand-
book with NIC interventions and NOC outcomes.
Pearson.
W
¨
uller, H., Behrens, J., Garthaus, M., Marquard, S., and
Remmers, H. (2019). A scoping review of augmented
reality in nursing. BMC nursing, 18(1):1–11.
Zerth, J., Jaensch, P., and M
¨
uller, S. (2021). Technik,
pflegeinnovation und implementierungsbedingungen.
In Jacobs, K., Kuhlmey, A., Greß, S., Klauber, J., and
Schwinger, A., editors, Pflege-Report 2021: Sicher-
stellung der Pflege: Bedarfslagen und Angebotsstruk-
turen, pages 157–172, Berlin, Heidelberg. Springer
Berlin Heidelberg.
A Concept for Daily Assessments During Nutrition Intake: Integrating Technology in the Nursing Process
619