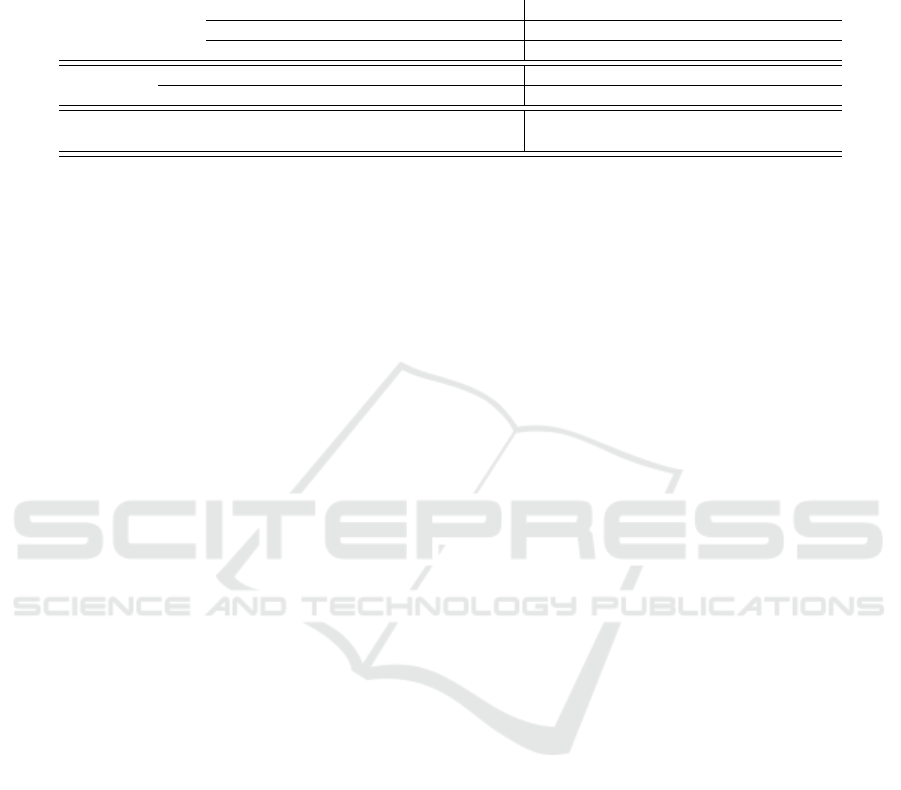
Table 1: Descriptive statistics on the results of the subjective evaluation. The statistics (M: Mean and STD: standard deviation)
are reported for each question asked to the participants (QI and QII) and for each sentence said by the virtual character
(Sentence 1 and 2), considering the gender of the participant (women and men) and the condition (baseline, neutral and
persuasive - Section 6.1).
Women Men
Baseline Neutral Persuasive Baseline Neutral Persuasive
M STD M STD M STD M STD M STD M STD
Sentence 1
QI 1.86 0.06 2.32 0.13 2.37 0.06 1.85 0.07 2.33 0.08 1.98 0.06
QII 1.94 0.56 2.54 0.14 2.40 0.1 1.97 0.08 2.39 0.15 1.91 0.02
Sentence 2
QI 2.26 0.03 2.10 0.03 2.76 0.09 1.79 0.06 2.02 0.14 2.62 0.02
QII 2.33 0.02 2.20 0.04 2.91 0.13 1.86 0.18 2.00 0.20 2.85 0.03
tations, and was created using the open-source tool-
boxes Greta and OpenFace, which only use some non-
verbal cues, there are certain limitations in the choice
of features. We propose on the future work to expand
our analysis and include other multimodal features,
particularly vocal features, to enhance the persuasive
model and to develop an automated artificial agent ca-
pable of expressing persuasive speech.
ACKNOWLEDGMENTS
This research was funded by the French National Re-
search Agency as part of the COPAINS project.
REFERENCES
Ahuja, C., Lee, D. W., Ishii, R., and Morency, L.-P. (2020).
No gestures left behind: Learning relationships be-
tween spoken language and freeform gestures. In
Findings of the association for computational linguis-
tics: EMNLP 2020, pages 1884–1895.
Ambady, N. and Rosenthal, R. (1992). Thin slices of ex-
pressive behavior as predictors of interpersonal con-
sequences: A meta-analysis. Psychological bulletin,
111(2):256.
Burgoon, J. K., Birk, T., and Pfau, M. (1990). Nonverbal
behaviors, persuasion, and credibility. Human com-
munication research, 17(1):140–169.
Cassell, J. (2001). H. vilhjilmsson, and t. BEAT: the Behav-
ior Expression Animation Toolkit.
Chidambaram, V., Chiang, Y.-H., and Mutlu, B. (2012). De-
signing persuasive robots: how robots might persuade
people using vocal and nonverbal cues. In Proceed-
ings of the seventh annual ACM/IEEE international
conference on Human-Robot Interaction, pages 293–
300.
Chiu, C.-C. and Marsella, S. (2014). Gesture generation
with low-dimensional embeddings. In AAMAS.
Dietterich, T. G. (1998). Approximate statistical tests
for comparing supervised classification learning algo-
rithms. Neural computation, 10(7):1895–1923.
Ghazali, A. S., Ham, J., Barakova, E. I., and Markopoulos,
P. (2018). Poker face influence: persuasive robot with
minimal social cues triggers less psychological reac-
tance. In 2018 27th IEEE International Symposium on
Robot and Human Interactive Communication (RO-
MAN), pages 940–946. IEEE.
Habibie, I., Xu, W., Mehta, D., Liu, L., Seidel, H.-P.,
Pons-Moll, G., Elgharib, M., and Theobalt, C. (2021).
Learning speech-driven 3d conversational gestures
from video. In IVA.
Hasegawa, D., Kaneko, N., Shirakawa, S., Sakuta, H., and
Sumi, K. (2018). Evaluation of speech-to-gesture gen-
eration using bi-directional lstm network. In IVA.
Kucherenko, T., Jonell, P., van Waveren, S., Henter,
G. E., Alexandersson, S., Leite, I., and Kjellstr
¨
om, H.
(2020). Gesticulator: A framework for semantically-
aware speech-driven gesture generation. In ICMI.
Lisetti, C., Amini, R., Yasavur, U., and Rishe, N. (2013). I
can help you change! an empathic virtual agent deliv-
ers behavior change health interventions. ACM Trans-
actions on Management Information Systems (TMIS),
4(4):1–28.
Marsella, S., Xu, Y., Lhommet, M., Feng, A., Scherer, S.,
and Shapiro, A. (2013). Virtual character performance
from speech. In SIGGRAPH, pages 25–35.
Miller, G. R. (2013). On being persuaded: Some basic dis-
tinctions. Sage Publications, Inc.
Miller, N., Maruyama, G., Beaber, R. J., and Valone, K.
(1976). Speed of speech and persuasion. Journal of
personality and social psychology, 34(4):615.
Nguyen, H., Masthoff, J., and Edwards, P. (2007). Persua-
sive effects of embodied conversational agent teams.
In International Conference on Human-Computer In-
teraction, pages 176–185. Springer.
Nojavanasghari, B., Gopinath, D., Koushik, J., Baltru
ˇ
saitis,
T., and Morency, L.-P. (2016). Deep multimodal fu-
sion for persuasiveness prediction. In ICMI.
Park, S., Shim, H. S., Chatterjee, M., Sagae, K., and
Morency, L.-P. (2014). Computational analysis of per-
suasiveness in social multimedia: A novel dataset and
multimodal prediction approach. In ICMI.
Park, S., Shim, H. S., Chatterjee, M., Sagae, K., and
Morency, L.-P. (2016). Multimodal analysis and pre-
diction of persuasiveness in online social multimedia.
ACM TiiS, 6(3):1–25.
Petukhova, V., Raju, M., and Bunt, H. (2017). Multimodal
markers of persuasive speech: Designing a virtual de-
bate coach. In INTERSPEECH, pages 142–146.
Tanner, R. and Chartrand, T. (2006). The convincing
chameleon: The impact of mimicry on persuasion.
ACR North American Advances.
ICAART 2024 - 16th International Conference on Agents and Artificial Intelligence
366