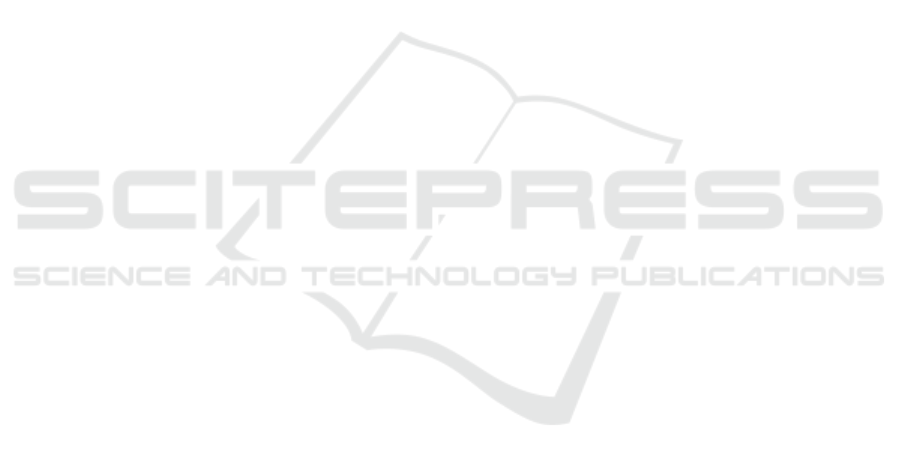
Deng, J., Dong, W., Socher, R., Li, L.-J., Li, K., and Fei-
Fei, L. (2009). Imagenet: A large-scale hierarchical
image database. In 2009 IEEE conference on com-
puter vision and pattern recognition, pages 248–255.
Ieee.
Diba, A., Fayyaz, M., Sharma, V., Arzani, M. M., Youse-
fzadeh, R., Gall, J., and Van Gool, L. (2018). Spatio-
temporal channel correlation networks for action clas-
sification. In Proceedings of the European Conference
on Computer Vision (ECCV), pages 284–299.
Dong, M., Fang, Z., Li, Y., Bi, S., and Chen, J. (2021).
Ar3d: attention residual 3d network for human action
recognition. Sensors, 21(5):1656.
Dosovitskiy, A., Fischer, P., Ilg, E., Hausser, P., Hazirbas,
C., Golkov, V., Van Der Smagt, P., Cremers, D., and
Brox, T. (2015). Flownet: Learning optical flow with
convolutional networks. In Proceedings of the IEEE
international conference on computer vision, pages
2758–2766.
Feichtenhofer, C., Fan, H., Malik, J., and He, K. (2019).
Slowfast networks for video recognition. In Proceed-
ings of the IEEE/CVF international conference on
computer vision, pages 6202–6211.
Goyal, R., Ebrahimi Kahou, S., Michalski, V., Materzyn-
ska, J., Westphal, S., Kim, H., Haenel, V., Fruend, I.,
Yianilos, P., Mueller-Freitag, M., et al. (2017). The”
something something” video database for learning and
evaluating visual common sense. In Proceedings of
the IEEE international conference on computer vi-
sion, pages 5842–5850.
Hara, K., Kataoka, H., and Satoh, Y. (2018). Can spa-
tiotemporal 3d cnns retrace the history of 2d cnns and
imagenet? In Proceedings of the IEEE conference
on Computer Vision and Pattern Recognition, pages
6546–6555.
He, K., Zhang, X., Ren, S., and Sun, J. (2016). Deep resid-
ual learning for image recognition. In Proceedings of
the IEEE conference on computer vision and pattern
recognition, pages 770–778.
Hu, J., Shen, L., and Sun, G. (2018). Squeeze-and-
excitation networks. In Proceedings of the IEEE con-
ference on computer vision and pattern recognition,
pages 7132–7141.
Ioffe, S. and Szegedy, C. (2015). Batch normalization: Ac-
celerating deep network training by reducing internal
covariate shift. In International conference on ma-
chine learning, pages 448–456. pmlr.
Ji, S., Xu, W., Yang, M., and Yu, K. (2012a). 3d convolu-
tional neural networks for human action recognition.
IEEE transactions on pattern analysis and machine
intelligence, 35(1):221–231.
Ji, S., Xu, W., Yang, M., and Yu, K. (2012b). 3d convolu-
tional neural networks for human action recognition.
IEEE transactions on pattern analysis and machine
intelligence, 35(1):221–231.
Jiang, B., Wang, M., Gan, W., Wu, W., and Yan, J. (2019).
Stm: Spatiotemporal and motion encoding for action
recognition. In Proceedings of the IEEE/CVF Interna-
tional Conference on Computer Vision, pages 2000–
2009.
Kay, W., Carreira, J., Simonyan, K., Zhang, B., Hillier, C.,
Vijayanarasimhan, S., Viola, F., Green, T., Back, T.,
Natsev, P., et al. (2017). The kinetics human action
video dataset. arXiv preprint arXiv:1705.06950.
Kuehne, H., Jhuang, H., Garrote, E., Poggio, T., and Serre,
T. (2011). Hmdb: a large video database for human
motion recognition. In 2011 International conference
on computer vision, pages 2556–2563. IEEE.
Kwon, H., Kim, M., Kwak, S., and Cho, M. (2020). Mo-
tionsqueeze: Neural motion feature learning for video
understanding. In Computer Vision–ECCV 2020:
16th European Conference, Glasgow, UK, August 23–
28, 2020, Proceedings, Part XVI 16, pages 345–362.
Springer.
Li, Y., Ji, B., Shi, X., Zhang, J., Kang, B., and Wang, L.
(2020). Tea: Temporal excitation and aggregation for
action recognition. In Proceedings of the IEEE/CVF
conference on computer vision and pattern recogni-
tion, pages 909–918.
Lin, J., Gan, C., and Han, S. (2019). Tsm: Temporal shift
module for efficient video understanding. In Pro-
ceedings of the IEEE/CVF international conference
on computer vision, pages 7083–7093.
Piergiovanni, A. and Ryoo, M. S. (2019). Representation
flow for action recognition. In Proceedings of the
IEEE/CVF conference on computer vision and pattern
recognition, pages 9945–9953.
Qiu, Z., Yao, T., and Mei, T. (2017). Learning spatio-
temporal representation with pseudo-3d residual net-
works. In proceedings of the IEEE International Con-
ference on Computer Vision, pages 5533–5541.
Simonyan, K. and Zisserman, A. (2014a). Two-stream con-
volutional networks for action recognition in videos.
Advances in neural information processing systems,
27.
Simonyan, K. and Zisserman, A. (2014b). Very deep con-
volutional networks for large-scale image recognition.
arXiv preprint arXiv:1409.1556.
Soomro, K., Zamir, A. R., and Shah, M. (2012). Ucf101:
A dataset of 101 human actions classes from videos in
the wild. arXiv preprint arXiv:1212.0402.
Sun, S., Kuang, Z., Sheng, L., Ouyang, W., and Zhang, W.
(2018). Optical flow guided feature: A fast and robust
motion representation for video action recognition. In
Proceedings of the IEEE conference on computer vi-
sion and pattern recognition, pages 1390–1399.
Tran, D., Bourdev, L., Fergus, R., Torresani, L., and Paluri,
M. (2015). Learning spatiotemporal features with 3d
convolutional networks. In Proceedings of the IEEE
international conference on computer vision, pages
4489–4497.
Tran, D., Ray, J., Shou, Z., Chang, S.-F., and Paluri, M.
(2017). Convnet architecture search for spatiotempo-
ral feature learning. arXiv preprint arXiv:1708.05038.
Tran, D., Wang, H., Torresani, L., Ray, J., LeCun, Y., and
Paluri, M. (2018). A closer look at spatiotemporal
convolutions for action recognition. In Proceedings of
the IEEE conference on Computer Vision and Pattern
Recognition, pages 6450–6459.
ICAART 2024 - 16th International Conference on Agents and Artificial Intelligence
1012