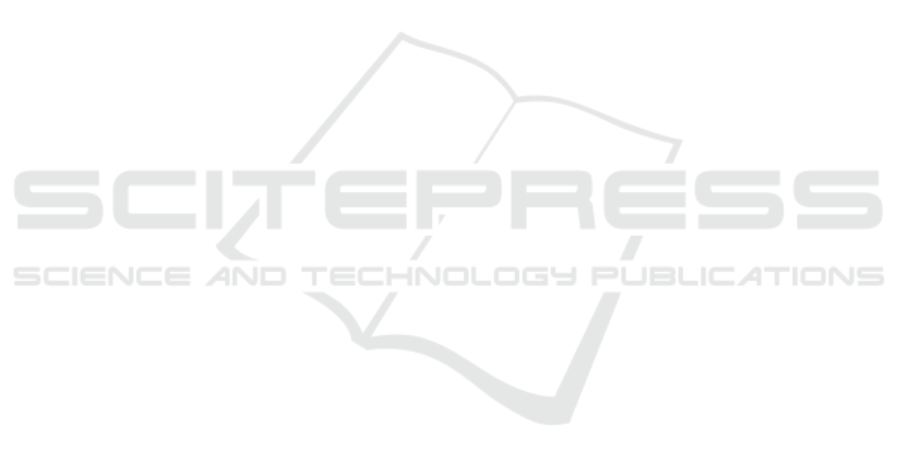
REFERENCES
Armato, S. G. et al. (2011). The lung image database con-
sortium (LIDC) and image database resource initiative
(IDRI): A completed reference database of lung nod-
ules on CT scans. Medical Physics, 38(2):915–931.
Bakas, S. et al. (2018). Identifying the best machine learn-
ing algorithms for brain tumor segmentation, progres-
sion assessment, and overall survival prediction in the
BRATS challenge. ArXiv, abs/1811.02629.
Bradski, G. (2000). The OpenCV Library. Dr. Dobb’s Jour-
nal of Software Tools.
Clark, K. et al. (2013). The Cancer Imaging Archive
(TCIA): Maintaining and operating a public reposi-
tory. Journal of Digital Imaging, 26(6):1045–1057.
Desai, S. et al. (2020). Chest imaging representing a
COVID-19 positive rural u.s. population. Scientific
Data, 7(1).
Diederik, P. et al. (2017). Adam: A method for stochastic
optimization.
Gupta, A. et al. (2016). Synthetic data for text localisation
in natural images. In Proceedings of the IEEE con-
ference on computer vision and pattern recognition,
pages 2315–2324.
Jenjaroenpun, P. et al. (2021). Two SARS-CoV-2 genome
sequences of isolates from rural U.S. patients harbor-
ing the D614G mutation, obtained using nanopore se-
quencing. Microbiology Resource Announcements,
10(1):10.1128/mra.01109–20.
Jodogne, S. (2018). The Orthanc ecosystem for medical
imaging. Journal of Digital Imaging, 31(3):341–352.
Jodogne, S. et al. (2013). Orthanc - a lightweight, restful DI-
COM server for healthcare and medical research. In
2013 IEEE 10th International Symposium on Biomed-
ical Imaging, pages 190–193. IEEE.
Johnson, A. et al. (2020). Deidentification of free-text med-
ical records using pre-trained bidirectional transform-
ers. In Proceedings of the ACM Conference on Health,
Inference, and Learning, CHIL ’20, page 214–221,
New York, NY, USA. Association for Computing Ma-
chinery.
Lehman, C. D. et al. (2007). MRI evaluation of the con-
tralateral breast in women with recently diagnosed
breast cancer. New England Journal of Medicine,
356(13):1295–1303.
Liang, J. et al. (1998). Performance evaluation of docu-
ment layout analysis algorithms on the UW data set.
Proceedings of SPIE - The International Society for
Optical Engineering.
Liao, M. et al. (2017). TextBoxes: A fast text detector with
a single deep neural network. Proceedings of the AAAI
Conference on Artificial Intelligence, 31(1).
Lin, T.-Y. et al. (2014). Microsoft COCO: Common objects
in context. In Computer Vision–ECCV 2014: 13th Eu-
ropean Conference, Zurich, Switzerland, September
6-12, 2014, Proceedings, Part V 13, pages 740–755.
Springer.
Liu, W. et al. (2016). SSD: Single shot multibox detec-
tor. In Computer Vision–ECCV 2016: 14th Euro-
pean Conference, Amsterdam, The Netherlands, Octo-
ber 11–14, 2016, Proceedings, Part I 14, pages 21–37.
Springer.
Lucas, S. et al. (2003). ICDAR 2003 robust reading compe-
titions. In Seventh International Conference on Doc-
ument Analysis and Recognition, 2003. Proceedings.,
pages 682–687.
Machtay, M. et al. (2013). Prediction of survival by
[18F]Fluorodeoxyglucose positron emission tomog-
raphy in patients with locally advanced non–small-
cell lung cancer undergoing definitive chemoradia-
tion therapy: Results of the ACRIN 6668/RTOG 0235
trial. Journal of Clinical Oncology, 31(30):3823–
3830.
Monteiro, E. et al. (2017). A de-identification pipeline for
ultrasound medical images in DICOM format. J. Med.
Syst., 41(5):1–16.
Newhauser, W. et al. (2014). Anonymization of DICOM
electronic medical records for radiation therapy. Com-
puters in biology and medicine, 53:134—140.
Rutherford, M. et al. (2021). A DICOM dataset for eval-
uation of medical image de-identification. Scientific
Data, 8(1).
Vcelak, P. et al. (2019). Identification and classification
of DICOM files with burned-in text content. Interna-
tional Journal of Medical Informatics, 126:128–137.
Vithya, Y. et al. (2020). A review of automatic end-to-end
de-identification: Is high accuracy the only metric?
Applied Artificial Intelligence, 34(3):251–269.
Wang, J. Z. et al. (1997). A textual information detection
and elimination system for secure medical image dis-
tribution. Proceedings : a conference of the American
Medical Informatics Association. AMIA Fall Sympo-
sium, page 896—896.
Wasserthal, J. et al. (2023). TotalSegmentator: Robust seg-
mentation of 104 anatomic structures in CT images.
Radiology: Artificial Intelligence, 5(5).
William, P. et al. (2021). Canadian association of radi-
ologists white paper on de-identification of medical
imaging: Part 1, general principles. Canadian Asso-
ciation of Radiologists Journal, 72(1):13–24. PMID:
33138621.
Wolf, C. et al. (2006). Object count/area graphs for the
evaluation of object detection and segmentation algo-
rithms. International Journal of Document Analysis
and Recognition (IJDAR), 8:280–296.
Ye, M. et al. (2022). DPText-DETR: Towards better scene
text detection with dynamic points in transformer.
Yingxuan, Z. et al. (2010). An automatic system to
detect and extract texts in medical images for de-
identification. In Liu, B. J. and Boonn, W. W., editors,
Medical Imaging 2010: Advanced PACS-based Imag-
ing Informatics and Therapeutic Applications, volume
7628, page 762803. International Society for Optics
and Photonics, SPIE.
Zhou, X. et al. (2017). EAST: an efficient and accurate
scene text detector. In Proceedings of the IEEE con-
ference on Computer Vision and Pattern Recognition,
pages 5551–5560.
BIOIMAGING 2024 - 11th International Conference on Bioimaging
304