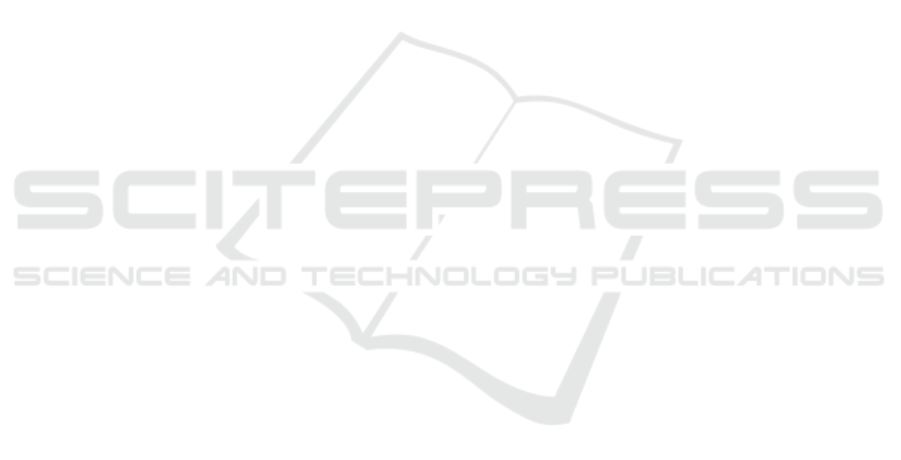
AV-PEA’s flexibility allows its adoption on any visual
transformer supported by the CLS token.
ACKNOWLEDGEMENTS
This work is supported by the Academy of Finland in
project 345791. We acknowledge the LUMI super-
computer, owned by the EuroHPC Joint Undertaking,
hosted by CSC and the LUMI consortium.
REFERENCES
Brown, T., Mann, B., Ryder, N., Subbiah, M., Kaplan, J. D.,
Dhariwal, P., Neelakantan, A., Shyam, P., Sastry, G.,
Askell, A., et al. (2020). Language models are few-
shot learners. NeurIPS, 33:1877–1901.
Bugliarello, E., Cotterell, R., Okazaki, N., and Elliott,
D. (2021). Multimodal pretraining unmasked: A
meta-analysis and a unified framework of vision-and-
language BERTs. Transactions of the Association for
Computational Linguistics, 9:978–994.
Chen, C.-F. R., Fan, Q., and Panda, R. (2021). Crossvit:
Cross-attention multi-scale vision transformer for im-
age classification. In ICCV, pages 357–366.
Chen, S., He, X., Guo, L., Zhu, X., Wang, W., Tang, J.,
and Liu, J. (2023). Valor: Vision-audio-language
omni-perception pretraining model and dataset. arXiv
preprint arXiv:2304.08345.
Deng, J., Dong, W., Socher, R., Li, L.-J., Li, K., and Fei-
Fei, L. (2009). Imagenet: A large-scale hierarchical
image database. In CVPR, pages 248–255.
Dosovitskiy, A., Beyer, L., Kolesnikov, A., Weissenborn,
D., Zhai, X., Unterthiner, T., Dehghani, M., Minderer,
M., Heigold, G., Gelly, S., Uszkoreit, J., and Houlsby,
N. (2021). An image is worth 16x16 words: Trans-
formers for image recognition at scale. In ICLR.
Gemmeke, J. F., Ellis, D. P., Freedman, D., Jansen, A.,
Lawrence, W., Moore, R. C., Plakal, M., and Rit-
ter, M. (2017). Audio set: An ontology and human-
labeled dataset for audio events. In ICASSP, pages
776–780.
Gong, Y., Chung, Y.-A., and Glass, J. (2021a). Ast: Audio
spectrogram transformer. In Interspeech, pages 571–
575.
Gong, Y., Chung, Y.-A., and Glass, J. (2021b). Psla: Im-
proving audio tagging with pretraining, sampling, la-
beling, and aggregation. IEEE/ACM Transactions on
Audio, Speech, and Language Processing, 29:3292–
3306.
Guzhov, A., Raue, F., Hees, J., and Dengel, A. (2022). Au-
dioclip: Extending clip to image, text and audio. In
ICASSP, pages 976–980.
Houlsby, N., Giurgiu, A., Jastrzebski, S., Morrone, B.,
De Laroussilhe, Q., Gesmundo, A., Attariyan, M., and
Gelly, S. (2019). Parameter-efficient transfer learning
for nlp. In ICML, pages 2790–2799.
Li, G., Wei, Y., Tian, Y., Xu, C., Wen, J.-R., and Hu,
D. (2022). Learning to answer questions in dy-
namic audio-visual scenarios. In CVPR, pages 19108–
19118.
Lin, Y.-B., Lei, J., Bansal, M., and Bertasius, G. (2022).
Eclipse: Efficient long-range video retrieval using
sight and sound. In ECCV, pages 413–430.
Lin, Y.-B., Sung, Y.-L., Lei, J., Bansal, M., and Bertasius,
G. (2023). Vision transformers are parameter-efficient
audio-visual learners. In CVPR, pages 2299–2309.
Nagrani, A., Yang, S., Arnab, A., Jansen, A., Schmid, C.,
and Sun, C. (2021). Attention bottlenecks for multi-
modal fusion. NeurIPS, 34:14200–14213.
Pan, J., Lin, Z., Zhu, X., Shao, J., and Li, H. (2022). St-
adapter: Parameter-efficient image-to-video transfer
learning. In NeurIPS, pages 26462–26477.
Radford, A., Kim, J. W., Hallacy, C., Ramesh, A., Goh, G.,
Agarwal, S., Sastry, G., Askell, A., Mishkin, P., Clark,
J., et al. (2021). Learning transferable visual models
from natural language supervision. In ICML, pages
8748–8763.
Rao, V., Khalil, M. I., Li, H., Dai, P., and Lu, J. (2022).
Dual perspective network for audio-visual event local-
ization. In ECCV, pages 689–704.
Schwartz, I., Schwing, A. G., and Hazan, T. (2019). A sim-
ple baseline for audio-visual scene-aware dialog. In
CVPR, pages 12548–12558.
Sung, Y.-L., Cho, J., and Bansal, M. (2022). Vl-adapter:
Parameter-efficient transfer learning for vision-and-
language tasks. In CVPR, pages 5227–5237.
Tian, Y., Shi, J., Li, B., Duan, Z., and Xu, C. (2018). Audio-
visual event localization in unconstrained videos. In
ECCV, pages 247–263.
Wang, W., Bao, H., Dong, L., Bjorck, J., Peng, Z., Liu, Q.,
Aggarwal, K., Mohammed, O. K., Singhal, S., Som,
S., and Wei, F. (2023). Image as a foreign language:
BEiT pretraining for vision and vision-language tasks.
In CVPR.
Xia, Y. and Zhao, Z. (2022). Cross-modal background sup-
pression for audio-visual event localization. In CVPR,
pages 19989–19998.
Yang, T., Zhu, Y., Xie, Y., Zhang, A., Chen, C., and Li,
M. (2023). AIM: Adapting image models for efficient
video action recognition. In ICLR.
Yun, H., Yu, Y., Yang, W., Lee, K., and Kim, G. (2021).
Pano-avqa: Grounded audio-visual question answer-
ing on 360deg videos. In ICCV, pages 2031–2041.
AV-PEA: Parameter-Efficient Adapter for Audio-Visual Multimodal Learning
737