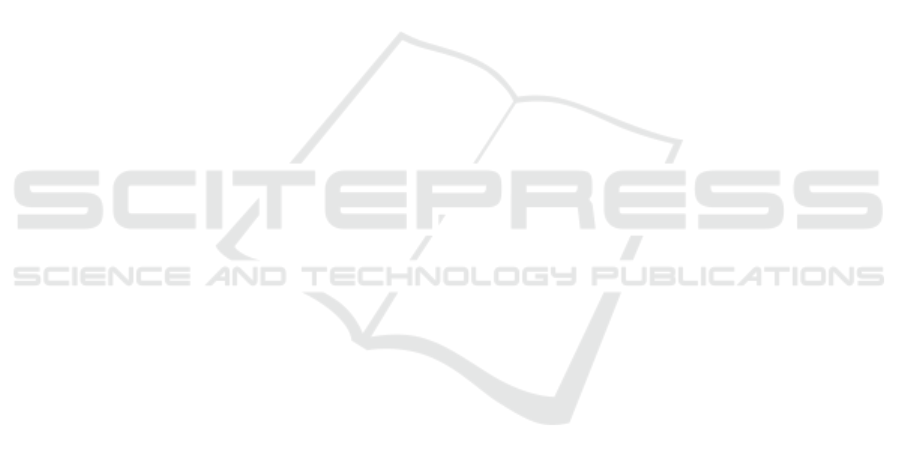
4 CONCLUSIONS
This study addresses the urgent need for timely and
accurate flood inundation mapping in the face of in-
creasing climate-induced challenges. We introduce
a novel dual encoder-decoder architecture that con-
sistently demonstrates superiority over state-of-the-
art models across both Sentinel-1 and Sentinel-2 im-
ages, as evidenced by comprehensive quantitative and
visual analyses. The versatility of this model is
crucial, highlighted through comparative analyses of
each satellite image under different conditions, re-
vealing strengths and limitations in various scenarios.
XAI is leveraged to better understand the
decision-making process of these models. It is shown
that not only is the proposed model the most accurate,
but it has also learned to detect flooded areas more ef-
fectively with greater confidence, showcasing its im-
proved trustworthiness for practical applications.
Despite the success of the proposed model, further
refinement techniques should be incorporated in the
future to enhance segmentation results, such as con-
ditional or Markov random fields. Attention mech-
anisms have also demonstrated superior results in a
range of computer vision tasks, particularly channel
and spatial attention. The incorporation of these tech-
niques can enhance the interpretability of the model
by highlighting the regions that the model paid atten-
tion to, without the need for additional post-hoc XAI
algorithms.
The use of additional data is likely to improve the
results of the work. Deep learning models continue
to im-prove as more data is added, allowing them to
learn more complex feature representations more ef-
fectively. The inclusion of different data types, such
as Digital Elevation Models (DEM) and Light Detec-
tion and Ranging (LiDAR), can provide more detailed
information about the topography of an area. This en-
ables the models to generate more precise flood maps,
as well as more detailed explanations through XAI re-
garding how environmental factors impact flood inun-
dation.
REFERENCES
Bonafilia, D., Tellman, B., Anderson, T., and Issenberg,
E. (2020). Sen1floods11: A georeferenced dataset
to train and test deep learning flood algorithms for
sentinel-1. In Proceedings of the IEEE/CVF Con-
ference on Computer Vision and Pattern Recognition
Workshops, pages 210–211.
Chattopadhay, A., Sarkar, A., Howlader, P., and Balasub-
ramanian, V. N. (2018). Grad-cam++: Generalized
gradient-based visual explanations for deep convolu-
tional networks. In 2018 IEEE winter conference on
applications of computer vision (WACV), pages 839–
847. IEEE.
Chen, L.-C., Zhu, Y., Papandreou, G., Schroff, F., and
Adam, H. (2018). Encoder-decoder with atrous sepa-
rable convolution for semantic image segmentation. In
Proceedings of the European conference on computer
vision (ECCV), pages 801–818.
Draelos, R. L. and Carin, L. (2020). Use hirescam instead
of grad-cam for faithful explanations of convolutional
neural networks. arXiv preprint arXiv:2011.08891.
Fu, R., Hu, Q., Dong, X., Guo, Y., Gao, Y., and Li, B.
(2020). Axiom-based grad-cam: Towards accurate vi-
sualization and explanation of cnns. arXiv preprint
arXiv:2008.02312.
Gebrehiwot, A. and Hashemi-Beni, L. (2020). Automated
indunation mapping: comparison of methods. In
IGARSS 2020-2020 IEEE International Geoscience
and Remote Sensing Symposium, pages 3265–3268.
IEEE.
Ghosh, B., Garg, S., and Motagh, M. (2022). Automatic
flood detection from sentinel-1 data using deep learn-
ing architectures. ISPRS Annals of the Photogramme-
try, Remote Sensing and Spatial Information Sciences,
3:201–208.
He, K., Zhang, X., Ren, S., and Sun, J. (2016). Deep resid-
ual learning for image recognition. In Proceedings of
the IEEE conference on computer vision and pattern
recognition, pages 770–778.
Helleis, M., Wieland, M., Krullikowski, C., Martinis, S.,
and Plank, S. (2022). Sentinel-1-based water and
flood mapping: Benchmarking convolutional neural
networks against an operational rule-based processing
chain. IEEE Journal of Selected Topics in Applied
Earth Observations and Remote Sensing, 15:2023–
2036.
Katiyar, V., Tamkuan, N., and Nagai, M. (2021). Near-
real-time flood mapping using off-the-shelf models
with sar imagery and deep learning. Remote Sensing,
13(12):2334.
Konapala, G., Kumar, S. V., and Ahmad, S. K. (2021). Ex-
ploring sentinel-1 and sentinel-2 diversity for flood in-
undation mapping using deep learning. ISPRS Journal
of Photogrammetry and Remote Sensing, 180:163–
173.
Landuyt, L., Van Wesemael, A., Schumann, G. J.-P.,
Hostache, R., Verhoest, N. E., and Van Coillie, F. M.
(2018). Flood mapping based on synthetic aper-
ture radar: An assessment of established approaches.
IEEE Transactions on Geoscience and Remote Sens-
ing, 57(2):722–739.
Li, Z. and Demir, I. (2023). U-net-based semantic clas-
sification for flood extent extraction using sar im-
agery and gee platform: A case study for 2019 cen-
tral us flooding. Science of The Total Environment,
869:161757.
Loshchilov, I. and Hutter, F. (2017). Sgdr: Stochastic gra-
dient descent with warm restarts.
Loshchilov, I. and Hutter, F. (2019). Decoupled weight de-
cay regularization.
ICAART 2024 - 16th International Conference on Agents and Artificial Intelligence
1034