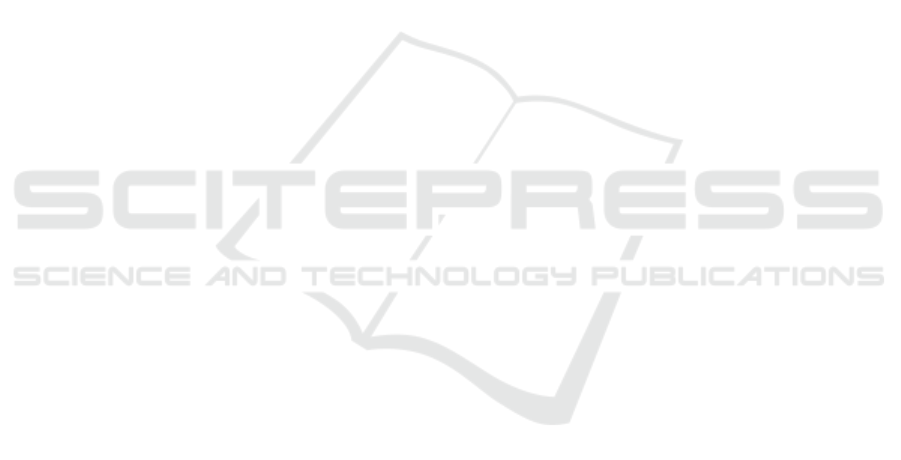
works. In Conference on Computer Vision and Pattern
Recognition, pages 16102–16112.
Chan, E. R., Monteiro, M., Kellnhofer, P., Wu, J., and Wet-
zstein, G. (2021). Pi-gan: Periodic implicit generative
adversarial networks for 3d-aware image synthesis. In
Conference on Computer Vision and Pattern Recogni-
tion, pages 5799–5809.
Choi, Y., Uh, Y., Yoo, J., and Ha, J. (2020). Stargan v2: Di-
verse image synthesis for multiple domains. In Con-
ference on Computer Vision and Pattern Recognition,
pages 8185–8194.
Deng, J., Guo, J., Xue, N., and Zafeiriou, S. (2019). Ar-
cface: Additive angular margin loss for deep face
recognition. In Proceedings of the IEEE/CVF Con-
ference on Computer Vision and Pattern Recognition
(CVPR).
Deng, Y., Wang, B., and yeung Shum, H. (2022a). Learning
detailed radiance manifolds for high-fidelity and 3d-
consistent portrait synthesis from monocular image.
2023 IEEE/CVF Conference on Computer Vision and
Pattern Recognition (CVPR), pages 4423–4433.
Deng, Y., Yang, J., Xiang, J., and Tong, X. (2022b).
GRAM: generative radiance manifolds for 3d-aware
image generation. In Conference on Computer Vision
and Pattern Recognition, pages 10663–10673.
Gadelha, M., Maji, S., and Wang, R. (2017). 3d shape in-
duction from 2d views of multiple objects. In Interna-
tional Conference on 3D Vision, pages 402–411.
Goodfellow, I. J., Pouget-Abadie, J., Mirza, M., Xu, B.,
Warde-Farley, D., Ozair, S., Courville, A. C., and Ben-
gio, Y. (2014). Generative adversarial nets. In Con-
ference on Neural Information Processing Systems,
pages 2672–2680.
Gu, J., Liu, L., Wang, P., and Theobalt, C. (2022). Stylenerf:
A style-based 3d aware generator for high-resolution
image synthesis. In International Conference on
Learning Representations.
Heusel, M., Ramsauer, H., Unterthiner, T., Nessler, B., and
Hochreiter, S. (2017). Gans trained by a two time-
scale update rule converge to a local nash equilibrium.
In Advances in Neural Information Processing Sys-
tems, pages 6626–6637.
Kajiya, J. T. (1986). The rendering equation. In Pro-
ceedings of the 13th annual conference on Computer
graphics and interactive techniques, pages 143–150.
Kaneko, T. (2022). Ar-nerf: Unsupervised learning of depth
and defocus effects from natural images with aper-
ture rendering neural radiance fields. In Conference
on Computer Vision and Pattern Recognition, pages
18387–18397.
Karras, T., Aittala, M., Laine, S., H
¨
ark
¨
onen, E., Hellsten, J.,
Lehtinen, J., and Aila, T. (2021a). Alias-free gener-
ative adversarial networks. In Conference on Neural
Information Processing Systems, pages 852–863.
Karras, T., Laine, S., and Aila, T. (2021b). A style-
based generator architecture for generative adversar-
ial networks. IEEE Trans. Pattern Anal. Mach. Intell.,
43(12):4217–4228.
Karras, T., Laine, S., Aittala, M., Hellsten, J., Lehtinen, J.,
and Aila, T. (2020). Analyzing and improving the im-
age quality of stylegan. In Conference on Computer
Vision and Pattern Recognition, pages 8107–8116.
Kim, G. and Chun, S. Y. (2022). Datid-3d: Diversity-
preserved domain adaptation using text-to-image dif-
fusion for 3d generative model. 2023 IEEE/CVF Con-
ference on Computer Vision and Pattern Recognition
(CVPR), pages 14203–14213.
Kumar, A., Bhunia, A. K., Narayan, S., Cholakkal, H., An-
wer, R. M., Khan, S. S., Yang, M., and Khan, F. S.
(2023). Generative multiplane neural radiance for 3d-
aware image generation. ArXiv, abs/2304.01172.
Kwak, J., Li, Y., Yoon, D., Kim, D., Han, D. K., and Ko, H.
(2022). Injecting 3d perception of controllable nerf-
gan into stylegan for editable portrait image synthesis.
In European Conference on Computer Vision, volume
13677 of Lecture Notes in Computer Science, pages
236–253. Springer.
Laine, S., Hellsten, J., Karras, T., Seol, Y., Lehtinen, J.,
and Aila, T. (2020). Modular primitives for high-
performance differentiable rendering. ACM Trans.
Graph., 39(6):194:1–194:14.
Liu, F. and Liu, X. (2022). 2d gans meet unsupervised
single-view 3d reconstruction. In European Confer-
ence on Computer Vision, volume 13661, pages 497–
514. Springer.
Max, N. (1995). Optical models for direct volume render-
ing. IEEE Transactions on Visualization and Com-
puter Graphics, 1(2):99–108.
Meetz, K., Meinzer, H., Baur, H., Engelmann, U., and
Scheppelmann, D. (1991). The heidelberg ray tracing
model. IEEE Computer Graphics and Applications,
11(06):34–43.
Mildenhall, B., Srinivasan, P. P., Tancik, M., Barron, J. T.,
Ramamoorthi, R., and Ng, R. (2022). Nerf: represent-
ing scenes as neural radiance fields for view synthesis.
Commun. ACM, 65(1):99–106.
Niemeyer, M. and Geiger, A. (2021a). CAMPARI: camera-
aware decomposed generative neural radiance fields.
In International Conference on 3D Vision, pages 951–
961.
Niemeyer, M. and Geiger, A. (2021b). GIRAFFE: repre-
senting scenes as compositional generative neural fea-
ture fields. In Conference on Computer Vision and
Pattern Recognition, pages 11453–11464.
Or-El, R., Luo, X., Shan, M., Shechtman, E., Park, J. J., and
Kemelmacher-Shlizerman, I. (2022). Stylesdf: High-
resolution 3d-consistent image and geometry genera-
tion. In Conference on Computer Vision and Pattern
Recognition, pages 13493–13503.
Poole, B., Jain, A., Barron, J. T., and Mildenhall, B. (2022).
Dreamfusion: Text-to-3d using 2d diffusion. ArXiv,
abs/2209.14988.
Rushmeier, H. E. and Torrance, K. E. (1987). The zonal
method for calculating light intensities in the presence
of a participating medium. ACM SIGGRAPH Com-
puter Graphics, 21(4):293–302.
Schwarz, K., Sauer, A., Niemeyer, M., Liao, Y., and Geiger,
A. (2022). Voxgraf: Fast 3d-aware image synthesis
with sparse voxel grids. In Conference on Neural In-
formation Processing Systems.
Alias-Free GAN for 3D-Aware Image Generation
231