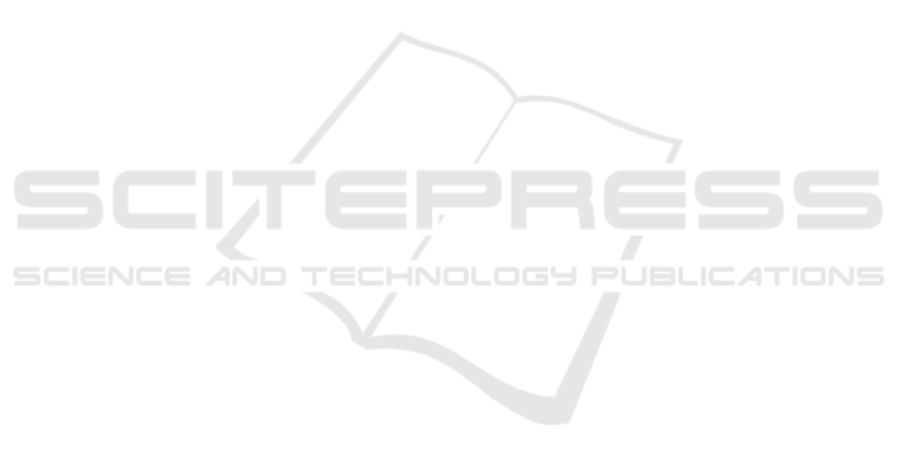
grids 2.0: producing quality-assessed soil information
for the globe. Soil Discuss, 2020:1–37.
Holzman, M. E., Carmona, F., Rivas, R., and Nicl
`
os, R.
(2018). Early assessment of crop yield from re-
motely sensed water stress and solar radiation data. IS-
PRS journal of photogrammetry and remote sensing,
145:297–308.
Ikram, R. M. A., Dai, H.-L., Ewees, A. A., Shiri, J., Kisi,
O., and Zounemat-Kermani, M. (2022). Application
of improved version of multi verse optimizer algo-
rithm for modeling solar radiation. Energy Reports,
8:12063–12080.
Jaiswal, P., Avraham, S., Ilic, K., Kellogg, E. A., McCouch,
S., Pujar, A., Reiser, L., Rhee, S. Y., Sachs, M. M.,
Schaeffer, M., et al. (2005). Plant ontology (po): a
controlled vocabulary of plant structures and growth
stages. Comparative and functional genomics, 6(7-
8):388–397.
Kaewboonma, N., Chansanam, W., and Buranarach, M.
(2020). Ontology-based big data analysis for orchid
smart farming. LIBRES: Library & In-formation Sci-
ence Research Electronic Journal, 29:292–296.
Karani, D. (2018). Introduction to word embedding and
word2vec. Towards Data Science, 1.
Li, D., Kang, L., Cheng, X., Li, D., Ji, L., Wang, K., and
Chen, Y. (2013). An ontology-based knowledge rep-
resentation and implement method for crop cultiva-
tion standard. Mathematical and computer modelling,
58(3-4):466–473.
Li, S., Kang, S., Zhang, L., Zhang, J., Du, T., Tong, L., and
Ding, R. (2016). Evaluation of six potential evapo-
transpiration models for estimating crop potential and
actual evapotranspiration in arid regions. Journal of
Hydrology, 543:450–461.
Malik, P., Sengupta, S., and Jadon, J. S. (2021). Com-
parative analysis of soil properties to predict fertility
and crop yield using machine learning algorithms. In
2021 11th International Conference on Cloud Com-
puting, Data Science & Engineering (Confluence),
pages 1004–1007. IEEE.
Micheli, E., Flint, L., Flint, A., Weiss, S., and Kennedy, M.
(2012). Downscaling future climate projections to the
watershed scale: A north san francisco bay case study.
San Francisco Estuary and Watershed Science, 10(4).
Ngo, Q. H., Kechadi, T., and Le-Khac, N.-A. (2020).
Oak: ontology-based knowledge map model for digi-
tal agriculture. In Future Data and Security Engineer-
ing: 7th International Conference, FDSE 2020, Quy
Nhon, Vietnam, November 25–27, 2020, Proceedings
7, pages 245–259. Springer.
Ngo, Q. H., Le-Khac, N.-A., and Kechadi, T. (2018). Ontol-
ogy based approach for precision agriculture. In Multi-
disciplinary Trends in Artificial Intelligence: 12th In-
ternational Conference, MIWAI 2018, Hanoi, Viet-
nam, November 18–20, 2018, Proceedings 12, pages
175–186. Springer.
Onishi, T. and Shiina, H. (2020). Distributed representation
computation using cbow model and skip–gram model.
In 2020 9th International Congress on Advanced Ap-
plied Informatics (IIAI-AAI), pages 845–846. IEEE.
Poudel, S. and Shaw, R. (2016). The relationships between
climate variability and crop yield in a mountainous en-
vironment: A case study in lamjung district, nepal.
Climate, 4(1):13.
Rajbhandari, S. and Keizer, J. (2012). The agrovoc concept
scheme–a walkthrough. Journal of Integrative Agri-
culture, 11(5):694–699.
Ristoski, P., Rosati, J., Di Noia, T., De Leone, R., and Paul-
heim, H. (2019). Rdf2vec: Rdf graph embeddings and
their applications. Semantic Web, 10(4):721–752.
Schr
¨
oder, M., Lockhoff, M., Shi, L., August, T., Bennartz,
R., Borbas, E., Brogniez, H., Calbet, X., Crewell, S.,
Eikenberg, S., et al. (2017). Gewex water vapor as-
sessment (g-vap), wcrp report 16/2017, world climate
research programme (wcrp): Geneva, switzerland, 216
pp.
Sharma, N., Thakur, M., Suryakumar, P., Mukherjee, P.,
Raza, A., Prakash, C. S., and Anand, A. (2022).
‘breathing out’under heat stress—respiratory control
of crop yield under high temperature. Agronomy,
12(4):806.
Terraclimate (2020). https://www.climatologylab.org. Ac-
cessed on September 23, 2023.
Wang, J., Vanga, S. K., Saxena, R., Orsat, V., and Raghavan,
V. (2018). Effect of climate change on the yield of
cereal crops: A review. Climate, 6(2):41.
Wang, Z., Yang, Y., Zhang, C., Guo, H., and Hou, Y. (2022).
Historical and future palmer drought severity index
with improved hydrological modeling. Journal of Hy-
drology, 610:127941.
Wei, Y.-y., Wang, R.-j., Hu, Y.-m., and Xue, W. (2012).
From web resources to agricultural ontology: a
method for semi-automatic construction. Journal of
Integrative Agriculture, 11(5):775–783.
Xie, N., Wang, W., and Yang, Y. (2008). Ontology-based
agricultural knowledge acquisition and application. In
Computer And Computing Technologies In Agricul-
ture, Volume I: First IFIP TC 12 International Con-
ference on Computer and Computing Technologies in
Agriculture (CCTA 2007), Wuyishan, China, August
18-20, 2007 1, pages 349–357. Springer.
Yuan, W., Zheng, Y., Piao, S., Ciais, P., Lombardozzi, D.,
Wang, Y., Ryu, Y., Chen, G., Dong, W., Hu, Z., et al.
(2019). Increased atmospheric vapor pressure deficit
reduces global vegetation growth. Science advances,
5(8):eaax1396.
Zhang, Y., Wu, C., Yeh, P. J.-F., Li, J., Hu, B. X., Feng,
P., and Jun, C. (2022). Evaluation and comparison of
precipitation estimates and hydrologic utility of chirps,
trmm 3b42 v7 and persiann-cdr products in various cli-
mate regimes. Atmospheric Research, 265:105881.
Zheng, Y.-L., He, Q.-Y., Ping, Q., and Ze, L. (2012). Con-
struction of the ontology-based agricultural knowl-
edge management system. Journal of Integrative Agri-
culture, 11(5):700–709.
A Quantitative Assessment Framework for Modelling and Evaluation Using Representation Learning in Smart Agriculture Ontology
1051