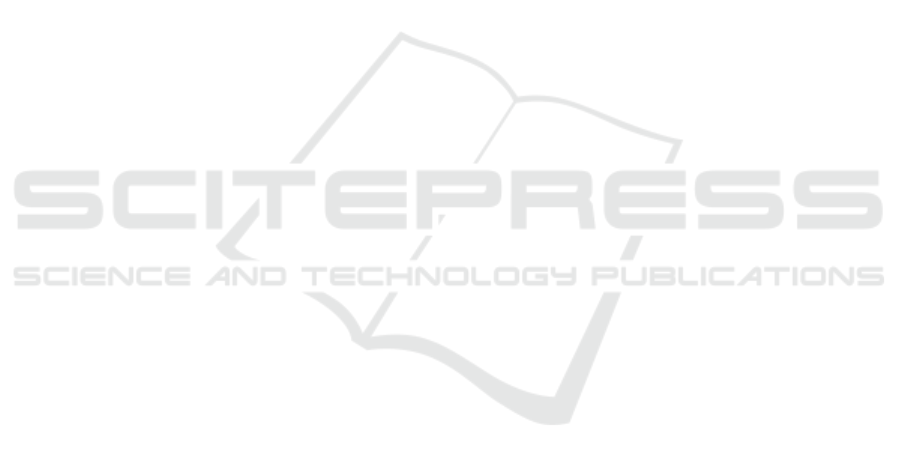
6 CONCLUSION AND FUTURE
WORK
Through a systematic experimental setup, covering
diverse scenarios, we identified key factors influenc-
ing the accuracy of aerial image-based relative object
position estimation, including plane misalignment,
3D structure uncertainties and calibration errors. We
developed mathematical models, and used them in a
unique simulator that allowed us to conclude the fol-
lowings.
Our main contribution is the evaluated projection
error of 3D-modelled traffic participants. We showed
that the distortion originating from this factor is min-
imal, when the object is below the camera (nadir) and
increases as the object moves away from the camera.
We also demonstrated that, the initial angular mis-
alignment of the marker and camera causes a signif-
icant error. Thus, a precise calibration is crucial for
aerial image-based positioning, especially for long-
range applications.
Setting the distortion and bias parameters to val-
ues that correspond to practically relevant use cases,
we found that in near range, the error of aerial image-
based relative object position estimation is less than
10 cm. Thus, under the aforementioned circum-
stances, the novel approach can achieve a precision
comparable to other, more complex, radar or LiDAR-
based methods. To reach a better precision, or a sim-
ilar precision for a wider range, one can use, for in-
stance, complex camera systems.
Future Work. In this stage, the simulator is ready
to build up a simple 3D world-model and simulate
basic error factors. Further developments can lead
to a more realistic simulated environment, including
topography; different weather conditions (rain/fog);
random noise. More realistic camera models can be
implemented, considering resolution, field of view,
and gimbal stabilization biases. Finally, more so-
phisticated error metrics can be developed for further
analysis.
ACKNOWLEDGEMENTS
Project no. C2286690 has been implemented with the
support provided by the ministry of culture and in-
novation of Hungary from the national research, de-
velopment and innovation found, financed under the
KDP-2023.
Furthermore, I would like to express my gratitude
to M
´
at
´
e Fugerth, Marcell Nagy, Anna V
´
amos and to
my company Robert Bosch Kft. for supporting my
project.
REFERENCES
Babinec, A. and Apeltauer, J. (2016). On accuracy of posi-
tion estimation from aerial imagery captured by low-
flying uavs. International Journal of Transportation
Science and Technology, 5(3):152–166. Unmanned
Aerial Vehicles and Remote Sensing.
Collins, R. T. (1992). Projective reconstruction of approxi-
mately planar scenes. volume 1838, page 174 – 185.
Dille, M., Grocholsky, B., and Nuske, S. (2011). Per-
sistent visual tracking and accurate geo-location of
moving ground targets by small air vehicles. In In-
fotech@Aerospace 2011, Reston, Virigina. American
Institute of Aeronautics and Astronautics.
Dosovitskiy, A., Ros, G., Codevilla, F., L
´
opez, A. M., and
Koltun, V. (2017). CARLA: an open urban driving
simulator. In 1st Annual Conference on Robot Learn-
ing, CoRL 2017, Mountain View, California, USA,
November 13-15, 2017, Proceedings, volume 78 of
Proceedings of Machine Learning Research, pages 1–
16.
Hartley, R. and Zisserman, A. (2003). Multiple View Geom-
etry in Computer Vision. Cambridge University Press,
New York, NY, USA, 2 edition.
Krajewski, R., Bock, J., Kloeker, L., and Eckstein, L.
(2018). The highd dataset: A drone dataset of natural-
istic vehicle trajectories on german highways for val-
idation of highly automated driving systems. In 2018
21st International Conference on Intelligent Trans-
portation Systems (ITSC), pages 2118–2125.
Kucharczyk, M., Hugenholtz, C. H., and Zou, X. (2018).
Uav–lidar accuracy in vegetated terrain. Journal of
Unmanned Vehicle Systems, 6(4):212–234.
Nguyen, K., Fookes, C., Sridharan, S., Tian, Y., Liu, F., Liu,
X., and Ross, A. (2022). The state of aerial surveil-
lance: A survey. arXiv preprint arXiv:2201.03080.
Nieuwenhuisen, M., Droeschel, D., Beul, M., and Behnke,
S. (2016). Autonomous navigation for micro aerial
vehicles in complex GNSS-denied environments. J.
Intell. Robot. Syst., 84(1-4):199–216.
Nuske, S. T., Dille, M., Grocholsky, B., and Singh, S.
(2010). Representing substantial heading uncertainty
for accurate geolocation by small UAVs. In AIAA
Guidance, Navigation, and Control Conference, Re-
ston, Virigina. American Institute of Aeronautics and
Astronautics.
Organisciak, D., Poyser, M., Alsehaim, A., Hu, S., Isaac-
Medina, B., Breckon, T., and Shum, H. (2022). UAV-
ReID: A benchmark on unmanned aerial vehicle re-
identification in video imagery. In Proceedings of the
17th International Joint Conference on Computer Vi-
sion, Imaging and Computer Graphics Theory and
Applications. SCITEPRESS - Science and Technol-
ogy Publications.
Patoliya, J., Mewada, H., Hassaballah, M., Khan, M. A.,
and Kadry, S. (2022). A robust autonomous naviga-
tion and mapping system based on GPS and LiDAR
data for unconstraint environment. Earth Sci. Inform.,
15(4):2703–2715.
Wang, P. (2021). Research on comparison of LiDAR and
camera in autonomous driving. J. Phys. Conf. Ser.,
2093(1):012032.
Error Analysis of Aerial Image-Based Relative Object Position Estimation
663