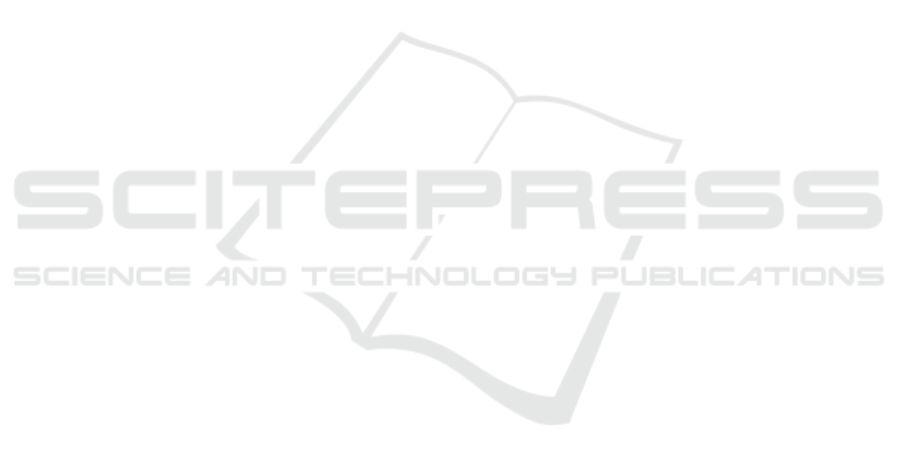
als to serious ocular conditions and visual impairment
later in life (Haarman et al., 2020). Over the past few
decades, the prevalence of myopia has increased on
a large scale, especially in East and Southeast Asian
countries, where the prevalence of myopia in young
adults is 80-90%, and an accompanying high preva-
lence of high myopia in young adults (10-20%) (Mor-
gan et al., 2012; Morgan et al., 2018). Several studies
have shown connections of myopia with parental my-
opia, longer education, more near work time , and less
time spent outdoors (Huang et al., 2015; P
¨
arssinen
and Kauppinen, 2022). In addition to the above fac-
tors, numerous other factors can influence the onset
and progression of myopia. Due to those factors, there
is great individual variation in the development of my-
opia and its progression. For the prevention of myopia
and slowing its progression, it is of great interest to
have prediction tools to know in advance which chil-
dren are at risk of developing myopia. The methods
developed in this manuscript aim to improve the pre-
dictability of the development of myopia.
Application to Myopia Diagnosis: In myopia
management, these technologies may have a crucial
role in the development of screening tools that ac-
curately predict the onset and progression of my-
opia in children. AI algorithms can analyze vast
datasets, identifying patterns that precede myopia de-
velopment. For instance, machine learning models
have been trained to predict myopia based on bio-
metric data, environmental factors, and genetic data.
These models are increasingly being utilized to alert
healthcare providers and parents for early signs of
myopia, enabling timely intervention.
Challenges in Implementation: Despite their po-
tential, the deployment of AI/ML/NLP in myopia di-
agnosis is not without challenges. Issues such as
data privacy, the need for large and diverse datasets
for model training, and the integration of these tech-
nologies into existing healthcare infrastructures are
ongoing concerns. Moreover, ensuring these ad-
vanced tools are accessible across various socio-
economic backgrounds remains a hurdle to achieving
widespread benefit.
1.2 Selected Initiatives
United States. In response to the myopia surge
among children, the United States has launched AI-
powered initiatives that intertwine research with prac-
tical applications. The National Eye Institute (NEI)
has catalyzed this movement by funding research into
AI models capable of predicting myopia progression,
with recent studies demonstrating a 30% improve-
ment in early detection accuracy. School-based pro-
grams have seen an infusion of AI, particularly dur-
ing the COVID-19 pandemic, where increased screen
time has been linked to a marked rise in myopia cases
(Kuehn, 2021; Ma et al., 2022). These programs
benefit from collaborations such as the one between
the American Ophthalmological Society and tech gi-
ants, aiming to create standardized screening proto-
cols across various states.
China, Singapore, Japan, and South Korea. The
Far Eastern countries have established a collabora-
tive network, the East Asian Ophthalmology Alliance
(EAOA), to facilitate the exchange of AI research and
technologies. Groundbreaking studies, like China’s
AI-based analysis of retinal images, have reported an
80% accuracy in predicting myopia, showcasing the
power of collaborative data sharing and algorithm de-
velopment. For example the (Foo et al., 2023). Sin-
gapore’s national program, integrating genetic data,
has seen a 20% increase in predictive precision post-
COVID-19, addressing the lifestyle changes that have
potentially accelerated myopia rates in children. This
collective effort underscores the potential for an inter-
national standard in myopia risk assessment.
Europe. Europe’s integration of AI in myopia de-
tection is evolving, with the European Vision In-
stitute leading multi-country studies that emphasize
early biomarker identification. Despite challenges
with data fragmentation, recent EU directives have
sought to unify health data standards, promoting re-
search like the Pan-European Myopia Study (PEMS),
which reported a 15% increase in myopia detection
since the pandemic began. The GDPR (General Data
Protection Regulation), while stringent, is adapting to
foster secure data sharing for AI applications, with
the recent establishment of the European Health Data
Space aiming to facilitate this shift.
1.3 Machine Learning Models
Trustworthiness as an Imperative in
Healthcare Applications
Trustworthiness in machine learning models tran-
scends a mere desirable quality, becoming imperative
in sensitive applications like healthcare. Users, partic-
ularly medical professionals, must be confident in the
models’ predictive capabilities to make crucial clin-
ical decisions. Trustworthiness is a composite mea-
sure, including but not limited to, effectiveness, ro-
bustness, fairness, interpretability, reliability, trans-
Predicting Children’s Myopia Risk: A Monte Carlo Approach to Compare the Performance of Machine Learning Models
1093