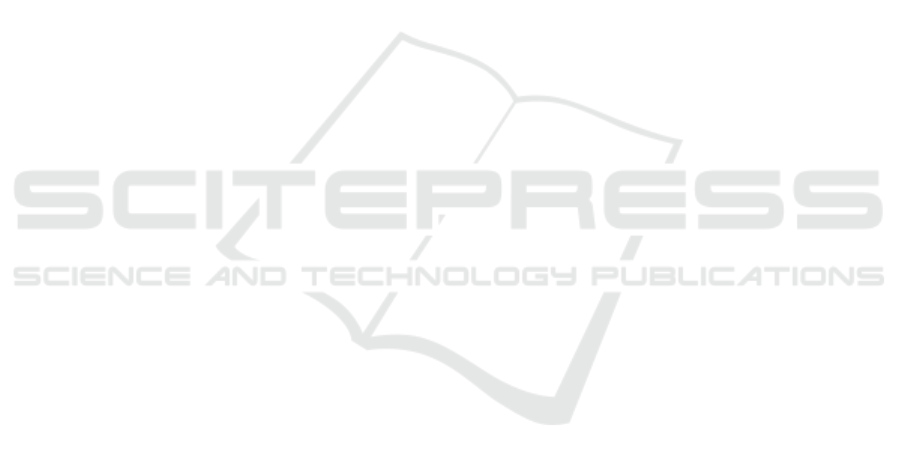
an initiative of the Irish Government’s Department of
Enterprise Trade and Employment (DETE).
REFERENCES
Bayram, F., Ahmed, B. S., and Kassler, A. (2022). From
concept drift to model degradation: An overview on
performance-aware drift detectors. Knowledge-Based
Systems, 245:108632.
Chen, J., Chen, K., Chen, H., Zou, Z., and Shi, Z. (2022). A
degraded reconstruction enhancement-based method
for tiny ship detection in remote sensing images with
a new large-scale dataset. IEEE Transactions on Geo-
science and Remote Sensing, 60:1–14.
Chen, L., Shi, W., and Deng, D. (2021). Improved yolov3
based on attention mechanism for fast and accurate
ship detection in optical remote sensing images. Re-
mote Sensing, 13(4).
Chen, Y. (2018). Airbus ship detection-traditional v . s .
convolutional neural network approach.
Ding, J., Xue, N., Xia, G.-S., Bai, X., Yang, W., Yang,
M. Y., Belongie, S., Luo, J., Datcu, M., Pelillo, M.,
and Zhang, L. (2022). Object detection in aerial im-
ages: A large-scale benchmark and challenges. IEEE
Transactions on Pattern Analysis and Machine Intel-
ligence, 44(11):7778–7796.
Fr
´
ıas-Blanco, I., Campo-
´
Avila, J. d., Ramos-Jim
´
enez, G.,
Morales-Bueno, R., Ortiz-D
´
ıaz, A., and Caballero-
Mota, Y. (2015). Online and non-parametric drift de-
tection methods based on hoeffding’s bounds. IEEE
Transactions on Knowledge and Data Engineering,
27(3):810–823.
Gama, J. a.,
ˇ
Zliobaitundefined, I., Bifet, A., Pechenizkiy,
M., and Bouchachia, A. (2014). A survey on concept
drift adaptation. ACM Comput. Surv., 46(4).
Hashmani, M., Syed, M., Alhussian, H., Rehman, M., and
Budiman, A. (2019). Accuracy performance degra-
dation in image classification models due to concept
drift. International Journal of Advanced Computer
Science and Applications, 10.
Huang, Z., Jiang, X., Wu, F., Fu, Y., Zhang, Y., Fu, T., and
Pei, J. (2023). An improved method for ship target
detection based on yolov4. Applied Sciences, 13(3).
Li, K., Wan, G., Cheng, G., Meng, L., and Han, J. (2020).
Object detection in optical remote sensing images: A
survey and a new benchmark. ISPRS Journal of Pho-
togrammetry and Remote Sensing, 159:296–307.
Liu, Z., Wang, H., Weng, L., and Yang, Y. (2016). Ship
rotated bounding box space for ship extraction from
high-resolution optical satellite images with complex
backgrounds. IEEE Geoscience and Remote Sensing
Letters, 13(8):1074–1078.
Mehmood, H., Khalid, A., Kostakos, P., Gilman, E., and
Pirttikangas, S. (2023). A novel edge architecture and
solution for detecting concept drift in smart environ-
ments. SSRN Electronic Journal.
Raab, C., Heusinger, M., and Schleif, F.-M. (2020). Re-
active soft prototype computing for concept drift
streams. Neurocomputing, 416:340–351.
Wang, J., Yang, W., Guo, H., Zhang, R., and Xia, G.-S.
(2021). Tiny object detection in aerial images. In 2020
25th International Conference on Pattern Recognition
(ICPR), pages 3791–3798.
Webb, G., Lee, L., and Goethals, B. e. a. (2018). Analyzing
concept drift and shift from sample data. Data Min
Knowl Disc, 32:1179–1199.
Wu, Z., Hou, B., Ren, B., Ren, Z., Wang, S., and Jiao, L.
(2021). A deep detection network based on interaction
of instance segmentation and object detection for sar
images. Remote Sensing, 13(13).
Xu, Q., Li, Y., and Shi, Z. (2022). Lmo-yolo: A ship detec-
tion model for low-resolution optical satellite imagery.
IEEE Journal of Selected Topics in Applied Earth Ob-
servations and Remote Sensing, 15:4117–4131.
Yadav, P. K., Thomasson, J. A., Searcy, S. W., Hardin,
R. G., Braga-Neto, U., Popescu, S. C., Martin, D. E.,
Rodriguez, R., Meza, K., Enciso, J., Diaz, J. S.,
and Wang, T. (2022). Assessing the performance of
yolov5 algorithm for detecting volunteer cotton plants
in corn fields at three different growth stages. Artifi-
cial Intelligence in Agriculture, 6:292–303.
Zhang, L., Li, C., and Sun, H. (2022). Object detec-
tion/tracking toward underwater photographs by re-
motely operated vehicles (rovs). Future Generation
Computer Systems, 126:163–168.
Zhang, Y., Yuan, Y., Feng, Y., and Lu, X. (2019). Hier-
archical and robust convolutional neural network for
very high-resolution remote sensing object detection.
IEEE Transactions on Geoscience and Remote Sens-
ing, 57(8):5535–5548.
Zhao, Z.-Q., Zheng, P., Xu, S.-T., and Wu, X. (2019). Ob-
ject detection with deep learning: A review. IEEE
Transactions on Neural Networks and Learning Sys-
tems, 30(11):3212–3232.
VISAPP 2024 - 19th International Conference on Computer Vision Theory and Applications
754