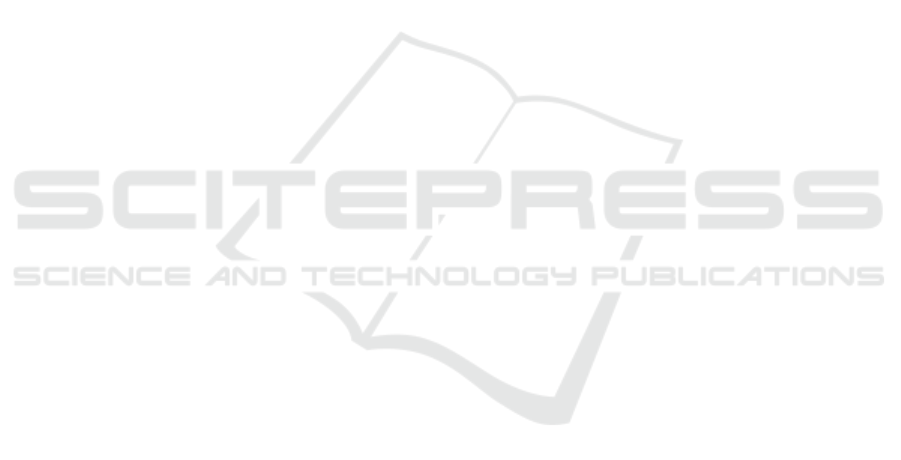
the University of Arizona. Caren Al Anaissy and
Srdjan Vesic were also supported by the project AG-
GREEY ANR22-CE23-0005 from the French Na-
tional Research Agency (ANR).
REFERENCES
Amgoud, L. and Ben-Naim, J. (2018). Evaluation of argu-
ments in weighted bipolar graphs. International Jour-
nal of Approximate Reasoning, 99:39–55.
Amgoud, L. and Prade, H. (2009). Using arguments for
making and explaining decisions. Artificial Intelli-
gence, 173:413–436.
Amgoud, L. and Serrurier, M. (2008). Agents that argue
and explain classifications. Autonomous Agents and
Multi-Agent Systems, 16(2):187–209.
Atkinson, K., Baroni, P., Giacomin, M., Hunter, A.,
Prakken, H., Reed, C., Simari, G. R., Thimm, M., and
Villata, S. (2017). Towards artificial argumentation.
AI Magazine, 38(3):25–36.
Baroni, P., Gabbay, D., Giacomin, M., and van der Torre, L.,
editors (2018). Handbook of Formal Argumentation.
College Publications.
Baroni, P., Romano, M., Toni, F., Aurisicchio, M., and
Bertanza, G. (2015). Automatic evaluation of design
alternatives with quantitative argumentation. Argu-
ment & Computation, 6(1):24–49.
Besnard, P. and Hunter, A. (2008). Elements of Argumenta-
tion. MIT Press.
Bubeck, S., Chandrasekaran, V., Eldan, R., Gehrke, J.,
Horvitz, E., Kamar, E., Lee, P., Lee, Y. T., Li, Y.,
Lundberg, S., et al. (2023). Sparks of artificial gen-
eral intelligence: Early experiments with gpt-4. arXiv
preprint arXiv:2303.12712.
Cabrio, E. and Villata, S. (2013). A natural language bipo-
lar argumentation approach to support users in on-
line debate interactions. Argument & Computation,
4(3):209–230.
Cabrio, E. and Villata, S. (2014). Node: A benchmark of
natural language arguments. In Computational Mod-
els of Argument, pages 449–450. IOS Press.
Cabrio, E., Villata, S., and Gandon, F. (2013). A sup-
port framework for argumentative discussions man-
agement in the web. In The Semantic Web: Seman-
tics and Big Data: 10th International Conference,
ESWC 2013, Montpellier, France, May 26-30, 2013.
Proceedings 10, pages 412–426. Springer.
Craandijk, D. and Bex, F. (2020). Deep learning for abstract
argumentation semantics. In Bessiere, C., editor, Pro-
ceedings of the Twenty-Ninth International Joint Con-
ference on Artificial Intelligence, IJCAI 2020, pages
1667–1673. ijcai.org.
Dung, P. M. (1995). On the Acceptability of Arguments and
its Fundamental Role in Non-Monotonic Reasoning,
Logic Programming and n-Person Games. Artificial
Intelligence, 77:321–357.
Gori, M., Monfardini, G., and Scarselli, F. (2005). A new
model for learning in graph domains. In Proceedings.
2005 IEEE international joint conference on neural
networks, volume 2, pages 729–734.
Kendall, M. G. (1938). A new measure of rank correlation.
Biometrika, 30(1/2):81–93.
Kipf, T. N. and Welling, M. (2016). Semi-supervised clas-
sification with graph convolutional networks. arXiv
preprint arXiv:1609.02907.
Kuhlmann, I. and Thimm, M. (2019). Using graph convo-
lutional networks for approximate reasoning with ab-
stract argumentation frameworks: A feasibility study.
In Scalable Uncertainty Management: 13th Interna-
tional Conference, SUM 2019, Compi
`
egne, France,
December 16–18, 2019, Proceedings, pages 24–37.
Springer.
LeCun, Y., Bottou, L., Bengio, Y., and Haffner, P. (1998).
Gradient-based learning applied to document recogni-
tion. Proceedings of the IEEE, 86(11):2278–2324.
Mossakowski, T. and Neuhaus, F. (2016). Bipolar
weighted argumentation graphs. arXiv preprint
arXiv:1611.08572.
Mossakowski, T. and Neuhaus, F. (2018). Modular seman-
tics and characteristics for bipolar weighted argumen-
tation graphs. arXiv preprint arXiv:1807.06685.
OpenAI (2023). Gpt-4 technical report.
Potyka, N. (2018). Continuous dynamical systems for
weighted bipolar argumentation. In KR, pages 148–
157.
Potyka, N. (2019). Extending modular semantics for bipolar
weighted argumentation. In KI 2019: Advances in Ar-
tificial Intelligence: 42nd German Conference on AI,
Kassel, Germany, September 23–26, 2019, Proceed-
ings 42, pages 273–276. Springer.
Potyka, N. (2021). Interpreting neural networks as quanti-
tative argumentation frameworks. In Proceedings of
the AAAI Conference on Artificial Intelligence, vol-
ume 35, pages 6463–6470.
Rago, A., Toni, F., Aurisicchio, M., Baroni, P., et al. (2016).
Discontinuity-free decision support with quantitative
argumentation debates. KR, 16:63–73.
Rahwan, I. and Simari, G. R., editors (2009). Argumenta-
tion in Artificial Intelligence. Springer.
Sainz, O., Campos, J. A., Garc
´
ıa-Ferrero, I., Etxaniz, J.,
and Agirre, E. (2023). Did chatgpt cheat on your test?
Last accessed: 18th July, 2023.
Scarselli, F., Gori, M., Tsoi, A. C., Hagenbuchner, M.,
and Monfardini, G. (2008). The graph neural net-
work model. IEEE transactions on neural networks,
20(1):61–80.
Smith, L. N. and Topin, N. (2019). Super-convergence:
Very fast training of neural networks using large learn-
ing rates. In Artificial intelligence and machine learn-
ing for multi-domain operations applications, volume
11006, pages 369–386. SPIE.
Veli
ˇ
ckovi
´
c, P., Cucurull, G., Casanova, A., Romero, A., Lio,
P., and Bengio, Y. (2017). Graph attention networks.
arXiv preprint arXiv:1710.10903.
Zhang, C., Zhang, C., Li, C., Qiao, Y., Zheng, S., Dam,
S. K., Zhang, M., Kim, J. U., Kim, S. T., Choi, J.,
et al. (2023). One small step for generative ai, one
giant leap for agi: A complete survey on chatgpt in
aigc era. arXiv preprint arXiv:2304.06488.
On Learning Bipolar Gradual Argumentation Semantics with Neural Networks
499