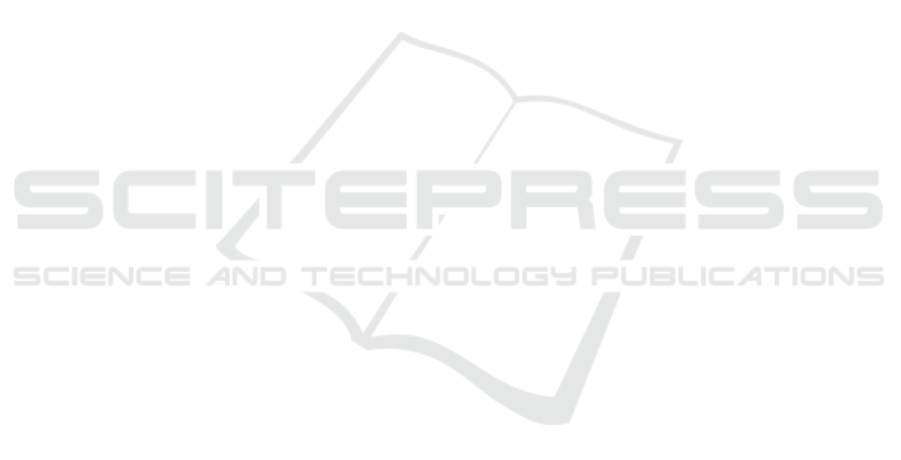
tems: Contributions Presented at the 18th UK Work-
shop on Computational Intelligence, September 5-7,
2018, Nottingham, UK, pages 191–202. Springer.
Jain, A., Patel, H., Nagalapatti, L., Gupta, N., Mehta, S.,
Guttula, S., Mujumdar, S., Afzal, S., Sharma Mittal,
R., and Munigala, V. (2020). Overview and impor-
tance of data quality for machine learning tasks. In
Proceedings of the 26th ACM SIGKDD international
conference on knowledge discovery & data mining,
pages 3561–3562.
Kingma, D. P. and Ba, J. (2017). Adam: A method for
stochastic optimization.
Kowsari, K., Heidarysafa, M., Brown, D. E., Meimandi,
K. J., and Barnes, L. E. (2018). Rmdl: Random mul-
timodel deep learning for classification. In Proceed-
ings of the 2nd international conference on informa-
tion system and data mining, pages 19–28.
Kwon, J., Kim, J., Park, H., and Choi, I. K. (2021). Asam:
Adaptive sharpness-aware minimization for scale-
invariant learning of deep neural networks. In Interna-
tional Conference on Machine Learning, pages 5905–
5914. PMLR.
Lei, X., Pan, H., and Huang, X. (2019). A dilated cnn model
for image classification. IEEE Access, 7:124087–
124095.
Li, Q., Cai, W., Wang, X., Zhou, Y., Feng, D. D., and Chen,
M. (2014). Medical image classification with convo-
lutional neural network. In 2014 13th international
conference on control automation robotics & vision
(ICARCV), pages 844–848. IEEE.
Liang, P., Shi, W., and Zhang, X. (2017). Remote sens-
ing image classification based on stacked denoising
autoencoder. Remote Sensing, 10(1):16.
Liu, Y., Wang, X., Zhang, Z., and Deng, F. (2023). A review
of deep leaning in image classification for mineral ex-
ploration. Minerals Engineering, 204:108433.
Louizos, C., Reisser, M., Soriaga, J., and Welling, M.
(2021). An expectation-maximization perspective on
federated learning. arXiv preprint arXiv:2111.10192.
Mazzia, V., Salvetti, F., and Chiaberge, M. (2021).
Efficient-capsnet: Capsule network with self-attention
routing. Scientific reports, 11(1):14634.
Mehdy, M., Ng, P., Shair, E., Saleh, N., Gomes, C., et al.
(2017). Artificial neural networks in image processing
for early detection of breast cancer. Computational
and mathematical methods in medicine, 2017.
Miranda, E., Aryuni, M., and Irwansyah, E. (2016). A sur-
vey of medical image classification techniques. In
2016 international conference on information man-
agement and technology (ICIMTech), pages 56–61.
IEEE.
Mirsadeghi, M., Shalchian, M., Kheradpisheh, S. R., and
Masquelier, T. (2023). Spike time displacement-based
error backpropagation in convolutional spiking neural
networks. Neural Computing and Applications, pages
1–16.
Najafabadi, M. M., Villanustre, F., Khoshgoftaar, T. M.,
Seliya, N., Wald, R., and Muharemagic, E. (2015).
Deep learning applications and challenges in big data
analytics. Journal of big data, 2(1):1–21.
Pereira, I. G., Guerin, J. M., Silva J
´
unior, A. G., Gar-
cia, G. S., Piscitelli, P., Miani, A., Distante, C., and
Gonc¸alves, L. M. G. (2020). Forecasting covid-19
dynamics in brazil: a data driven approach. Interna-
tional Journal of Environmental Research and Public
Health, 17(14):5115.
Pham, H. and Le, Q. (2021). Autodropout: Learning
dropout patterns to regularize deep networks. In Pro-
ceedings of the AAAI Conference on Artificial Intelli-
gence, volume 35.11, pages 9351–9359.
Ram
´
e, A., Sun, R., and Cord, M. (2021). Mixmo: Mixing
multiple inputs for multiple outputs via deep subnet-
works. In Proceedings of the IEEE/CVF International
Conference on Computer Vision, pages 823–833.
Sharma, D. and Jindal, G. (2011). Identifying lung cancer
using image processing techniques. In International
Conference on Computational Techniques and Arti-
ficial Intelligence (ICCTAI), volume 17, pages 872–
880. Citeseer.
Shi, M., Zhou, Y., Ye, Q., and Lv, J. (2022). Personalized
federated learning with hidden information on person-
alized prior. arXiv preprint arXiv:2211.10684.
Tanveer, M. S., Khan, M. U. K., and Kyung, C.-M. (2021).
Fine-tuning darts for image classification. In 2020
25th International Conference on Pattern Recognition
(ICPR), pages 4789–4796. IEEE.
Tseng, C.-H., Liu, H.-C., Lee, S.-J., and Zeng, X. (2022).
Perturbed gradients updating within unit space for
deep learning. In 2022 International Joint Conference
on Neural Networks (IJCNN), pages 01–08. IEEE.
Turay, T. and Vladimirova, T. (2022). Toward performing
image classification and object detection with convo-
lutional neural networks in autonomous driving sys-
tems: A survey. IEEE Access, 10:14076–14119.
Xiao, H., Rasul, K., and Vollgraf, R. (2017).
Fashion-mnist: a novel image dataset for bench-
marking machine learning algorithms. cite
arxiv:1708.07747Comment: Dataset is freely avail-
able at https://github.com/zalandoresearch/fashion-
mnist Benchmark is available at http://fashion-
mnist.s3-website.eu-central-1.amazonaws.com/.
Yang, Z., Wu, X., Huang, P., Zhang, F., Wan, M., and Lai, Z.
(2022). Orthogonal autoencoder regression for image
classification. Information Sciences, 618:400–416.
Zhang, M., Li, W., and Du, Q. (2018). Diverse region-based
cnn for hyperspectral image classification. IEEE
Transactions on Image Processing, 27(6):2623–2634.
Zhao, J., Mathieu, M., Goroshin, R., and Lecun, Y. (2015).
Stacked what-where auto-encoders. arXiv preprint
arXiv:1506.02351.
Zhou, S., Xue, Z., and Du, P. (2019). Semisupervised
stacked autoencoder with cotraining for hyperspec-
tral image classification. IEEE Transactions on Geo-
science and Remote Sensing, 57(6):3813–3826.
VISAPP 2024 - 19th International Conference on Computer Vision Theory and Applications
710