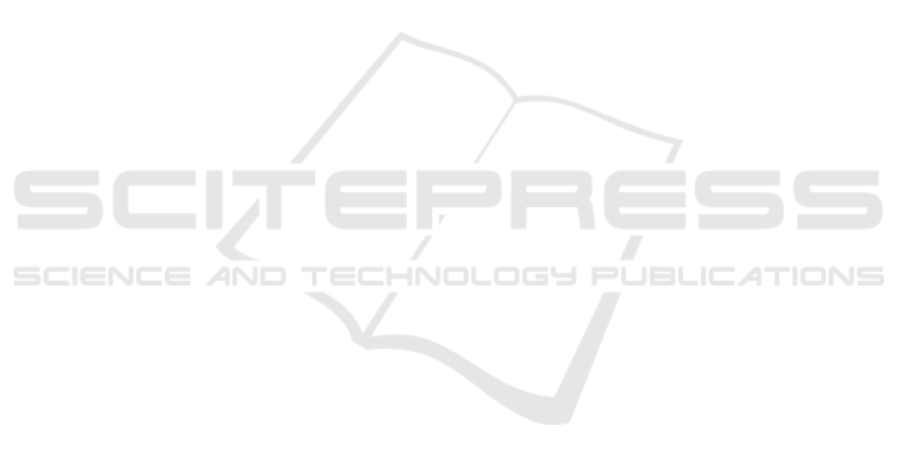
6 CONCLUSION
In conclusion, this paper introduces EAPC, a frame-
work for generating realistic talking faces from audio
and reference image input. We also propose the Dual-
LSTM, which utilizes dual LSTM layers and incor-
porates skip connections from the prior audio frame
to the current audio frame, thereby enhancing the
temporal characteristics of our method. Additionally,
the Dual-LSTM module employs the attention mech-
anism to support emotion control, effectively gener-
ating emotionally animated facial landmark frames.
Qualitative results and our ablation study validate the
effectiveness of our method, leading to the achieve-
ment of competitive results with state-of-the-art. This
research opens up possibilities for more advanced and
natural facial animation generation techniques in var-
ious applications, including video production, virtual
avatars, and virtual reality experiences.
ACKNOWLEDGEMENTS
This research is funded by the University of Science,
VNU-HCM, Vietnam under grant number CNTT
2022-14.
REFERENCES
Cao, H., Cooper, D. G., Keutmann, M. K., Gur, R. C.,
Nenkova, A., and Verma, R. (2014). Crema-d: Crowd-
sourced emotional multimodal actors dataset. IEEE
Transactions on Affective Computing, 5(4):377–390.
Chen, L., Li, Z., Maddox, R. K., Duan, Z., and Xu, C.
(2018). Lip movements generation at a glance.
Chen, L., Maddox, R. K., Duan, Z., and Xu, C. (2019).
Hierarchical cross-modal talking face generation with
dynamic pixel-wise loss. In Proceedings of the
IEEE/CVF conference on computer vision and pattern
recognition, pages 7832–7841.
Chung, J. S., Jamaludin, A., and Zisserman, A. (2017). You
said that? arXiv preprint arXiv:1705.02966.
Eskimez, S. E., Zhang, Y., and Duan, Z. (2021). Speech
driven talking face generation from a single image and
an emotion condition.
Heusel, M., Ramsauer, H., Unterthiner, T., Nessler, B., and
Hochreiter, S. (2018). Gans trained by a two time-
scale update rule converge to a local nash equilibrium.
Isola, P., Zhu, J.-Y., Zhou, T., and Efros, A. A. (2017).
Image-to-image translation with conditional adversar-
ial networks. In Proceedings of the IEEE conference
on computer vision and pattern recognition, pages
1125–1134.
Ji, X., Zhou, H., Wang, K., Wu, Q., Wu, W., Xu, F., and
Cao, X. (2022). Eamm: One-shot emotional talking
face via audio-based emotion-aware motion model.
In ACM SIGGRAPH 2022 Conference Proceedings,
pages 1–10.
Ji, X., Zhou, H., Wang, K., Wu, W., Loy, C. C., Cao, X., and
Xu, F. (2021). Audio-driven emotional video portraits.
In Proceedings of the IEEE/CVF conference on com-
puter vision and pattern recognition, pages 14080–
14089.
Larkin, K. G. (2015). Structural similarity index ssimpli-
fied: Is there really a simpler concept at the heart of
image quality measurement?
Liu, X., Xu, Y., Wu, Q., Zhou, H., Wu, W., and Zhou, B.
(2022). Semantic-aware implicit neural audio-driven
video portrait generation. In European Conference on
Computer Vision, pages 106–125. Springer.
Lugaresi, C., Tang, J., Nash, H., McClanahan, C., Uboweja,
E., Hays, M., Zhang, F., Chang, C.-L., Yong, M. G.,
Lee, J., et al. (2019). Mediapipe: A framework
for building perception pipelines. arXiv preprint
arXiv:1906.08172.
Mittal, G. and Wang, B. (2020). Animating face using dis-
entangled audio representations. In Proceedings of
the IEEE/CVF Winter Conference on Applications of
Computer Vision, pages 3290–3298.
Sinha, S., Biswas, S., Yadav, R., and Bhowmick, B. (2022).
Emotion-controllable generalized talking face genera-
tion.
Song, Y., Zhu, J., Li, D., Wang, A., and Qi, H. (2019). Talk-
ing face generation by conditional recurrent adversar-
ial network. In Proceedings of the Twenty-Eighth In-
ternational Joint Conference on Artificial Intelligence,
IJCAI-19, pages 919–925. International Joint Confer-
ences on Artificial Intelligence Organization.
Song, Y., Zhu, J., Li, D., Wang, X., and Qi, H. (2018). Talk-
ing face generation by conditional recurrent adversar-
ial network. arXiv preprint arXiv:1804.04786.
Suwajanakorn, S., Seitz, S. M., and Kemelmacher-
Shlizerman, I. (2017). Synthesizing obama: Learning
lip sync from audio. ACM Trans. Graph., 36(4).
Thies, J., Elgharib, M., Tewari, A., Theobalt, C., and
Nießner, M. (2020). Neural voice puppetry: Audio-
driven facial reenactment.
Vaswani, A., Shazeer, N., Parmar, N., Uszkoreit, J., Jones,
L., Gomez, A. N., Kaiser, Ł., and Polosukhin, I.
(2017). Attention is all you need. Advances in neural
information processing systems, 30.
Vougioukas, K., Petridis, S., and Pantic, M. (2019). Realis-
tic speech-driven facial animation with gans.
Wang, J., Qian, X., Zhang, M., Tan, R. T., and Li, H.
(2023a). Seeing what you said: Talking face gener-
ation guided by a lip reading expert. In Proceedings
of the IEEE/CVF Conference on Computer Vision and
Pattern Recognition, pages 14653–14662.
Wang, J., Zhao, K., Zhang, S., Zhang, Y., Shen, Y.,
Zhao, D., and Zhou, J. (2023b). Lipformer: High-
fidelity and generalizable talking face generation with
a pre-learned facial codebook. In Proceedings of the
IEEE/CVF Conference on Computer Vision and Pat-
tern Recognition, pages 13844–13853.
EAPC: Emotion and Audio Prior Control Framework for the Emotional and Temporal Talking Face Generation
529