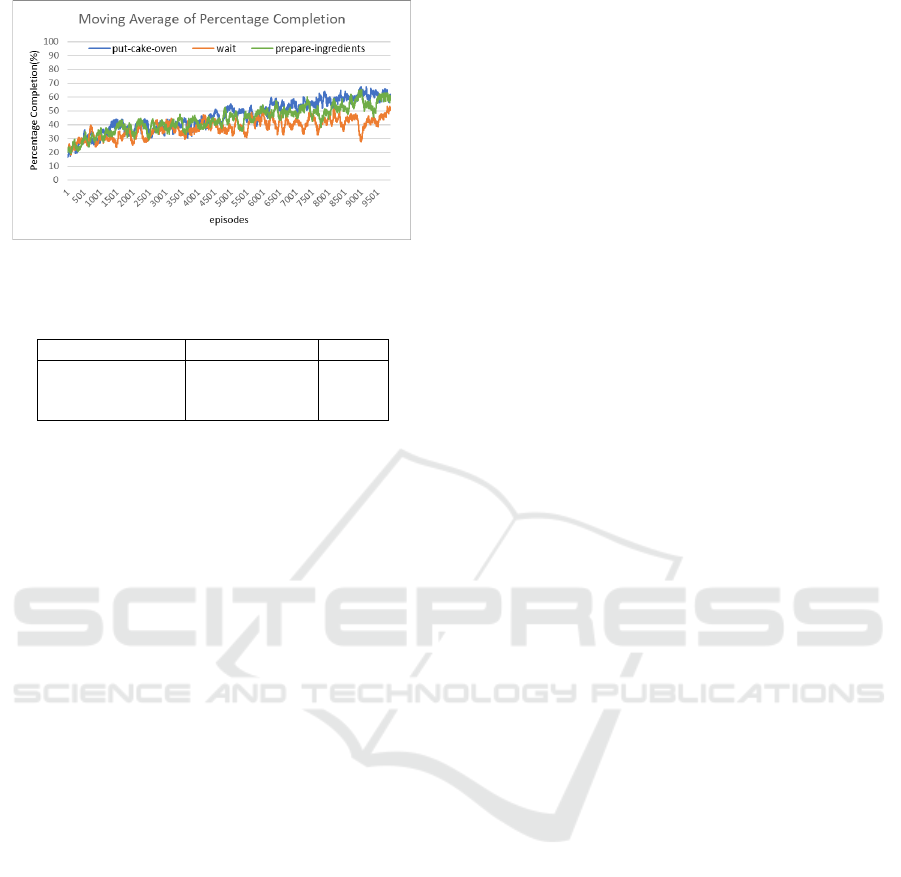
Figure 8: MAPC with various sub-goals for “baking a
cake”.
Table 3: Various sub-goals.
Subgoal Connected nodes Bypass
put-cake-oven 9 2
wait 11 6
prepare-ingredients 9 4
7 CONCLUSION
This paper focused on the generalization and learning
mechanisms of deep reinforcement learning and ana-
lyzed the learned commonsense knowledge. In par-
ticular, we discussed the impact of neural networks
on the acquisition of commonsense knowledge and
the challenges of ScriptWorld in terms of reward de-
sign. The experiment showed that DQN outperforms
Q-learning in ScriptWorld, which indicates that the
neural network generalized the input as event-level
commonsense knowledge. In addition, we found that
the brief method to impart sub-goals sometimes im-
proved the learning accuracy, indicating that the re-
ward design was effective.
One of the future work is to establish the reward
design method for imparting scenario-level common-
sense knowledge. To acquire such commonsense
knowledge, it is necessary to focus not only on the
scenario but also on partial actions within the sce-
nario. In fact, it was found that setting sub-goals is
effective to some extent, but there are still issues to be
addressed regarding the placement of these sub-goals.
The method we are considering is to set a reward for
every node and change the value of the reward accord-
ing to the importance of each node. Another aspect of
this environment is that the success rate of reaching
the goal is low, and the data used for learning often
has a negative component. It could be devised in such
a way that data with a positive element would be heav-
ily reflected in the learning process. In addition, since
Handicap is available in ScriptWorld, we would like
to consider how to utilize it to further improve learn-
ing efficiency.
REFERENCES
Brown, D., Goo, W., Nagarajan, P., and Niekum, S. (2019).
Extrapolating beyond suboptimal demonstrations via
inverse reinforcement learning from observations. In
Proceedings of the 36th International Conference on
Machine Learning, ICML ’19, pages 783–792, Long
Beach, California, USA.
Devlin, S. and Kudenko, D. (2012). Dynamic potential-
based reward shaping. In Proceedings of the 11th
International Conference on Autonomous Agents and
Multiagent Systems - Volume 1, AAMAS ’12, page
433–440.
Hausknecht, M., Ammanabrolu, P., C
ˆ
ot
´
e, M.-A., and Yuan,
X. (2020). Interactive fiction games: A colossal ad-
venture. Proceedings of the AAAI Conference on Ar-
tificial Intelligence, 34(05):7903–7910.
Joshi, A., Ahmad, A., Pandey, U., and Modi, A. (2023).
Scriptworld: Text based environment for learning pro-
cedural knowledge. In Proceedings of the Thirty-
Second International Joint Conference on Artificial
Intelligence, IJCAI-23. Main Track.
Kuderer, M., Gulati, S., and Burgard, W. (2015). Learning
driving styles for autonomous vehicles from demon-
stration. In 2015 IEEE International Conference on
Robotics and Automation (ICRA), pages 2641–2646.
Mannion, P., Devlin, S., Duggan, J., and Howley, E. (2018).
Reward shaping for knowledge-based multi-objective
multi-agent reinforcement learning. The Knowledge
Engineering Review, 33:e23.
Mnih, V., Kavukcuoglu, K., Silver, D., Rusu, A. A., Ve-
ness, J., Bellemare, M. G., Graves, A., Riedmiller,
M., Fidjeland, A. K., Ostrovski, G., Petersen, S.,
Beattie, C., Sadik, A., Antonoglou, I., King, H., Ku-
maran, D., Wierstra, D., Legg, S., and Hassabis, D.
(2015). Human-level control through deep reinforce-
ment learning. Nature, 518(7540):529–533.
Ng, A. Y., Harada, D., and Russell, S. J. (1999). Pol-
icy invariance under reward transformations: Theory
and application to reward shaping. In Proceedings
of the Sixteenth International Conference on Machine
Learning, ICML ’99, page 278–287.
Russell, S. (1998). Learning agents for uncertain en-
vironments (extended abstract). In Proceedings of
the Eleventh Annual Conference on Computational
Learning Theory, COLT’ 98, page 101–103, New
York, NY, USA.
Sutton, R. S. and Barto, A. G. (1998). Introduction to Re-
inforcement Learning. MIT Press, Cambridge, MA,
USA, 1st edition.
Wanzare, L. D. A., Zarcone, A., Thater, S., and Pinkal, M.
(2016). A crowdsourced database of event sequence
descriptions for the acquisition of high-quality script
knowledge. In Proceedings of the Tenth International
Conference on Language Resources and Evaluation
(LREC’16), pages 3494–3501.
Wu, Z., Sun, L., Zhan, W., Yang, C., and Tomizuka, M.
(2020). Efficient sampling-based maximum entropy
inverse reinforcement learning with application to au-
tonomous driving. IEEE Robotics and Automation
Letters, 5(4):5355–5362.
ICAART 2024 - 16th International Conference on Agents and Artificial Intelligence
1220