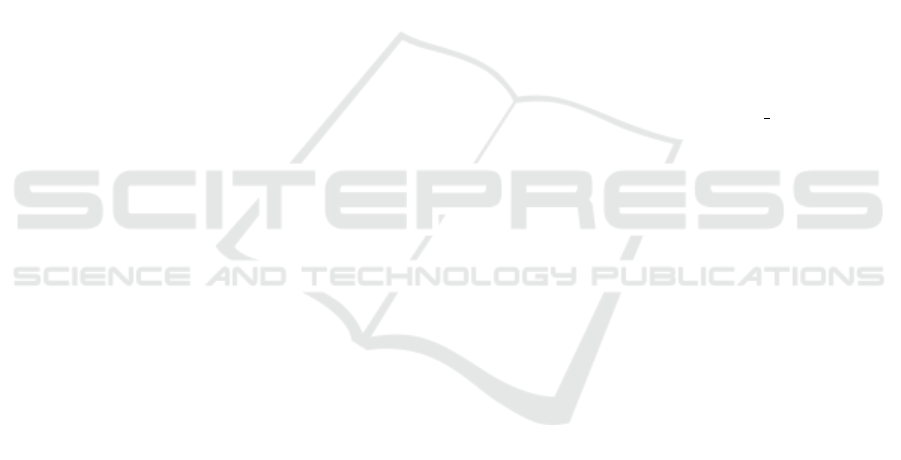
stance, the image with highest d
hauss
(a value of 6.523
mm) is obtained for the last image in Figure 10 and it
is not the image with the highest mean tree ring width
(a value of 11.988 mm). Results show that introduc-
ing a confidence map is relevant.
5 CONCLUSIONS
A new framework is presented by combining a direc-
tional filter to detect tree rings and a confidence map
to perform measurements on detected tree rings. The
method works on untreated cross-section wood im-
ages. Over the experiments, it achieves a RMSE of
0.830 mm for the (Longuetaud et al., 2022) dataset,
slightly better than a deep learning method of 1.482
mm. We also obtained great results for Urudendro
dataset (Marichal et al., 2023b) with a RMSE of
14.626 pixels. Note that combining the confidence
map and deep learning offers also great results.
In future works, we will include automatic pith de-
tection, cross-section segmentation, other tree species
and other deep learning methods. We will also adapt
our method to other applications where orientation
plays an important role such as fingerprint images.
REFERENCES
Aydin, O. U., Taha, A. A., Hilbert, A., Khalil, A. A., Gali-
novic, I., Fiebach, J. B., Frey, D., and Madai, V. I.
(2021). On the usage of average hausdorff distance
for segmentation performance assessment: hidden er-
ror when used for ranking. European radiology exper-
imental, 5:1–7.
Chen, L.-C., Zhu, Y., Papandreou, G., Schroff, F., and
Adam, H. (2018). Encoder-decoder with atrous sep-
arable convolution for semantic image segmentation.
In Proceedings of ECCV, pages 801–818.
Conner, W. S., Schowengerdt, R. A., Munro, M., and
Hughes, M. K. (1998). Design of a computer vi-
sion based tree ring dating system. In IEEE South-
west Symposium on Image Analysis and Interpretation
(Cat. No. 98EX165), pages 256–261.
Decelle, R., Ngo, P., Debled-Rennesson, I., Mothe, F., and
Longuetaud, F. (2021). Digital straight segment filter
for geometric description. In International Confer-
ence on Discrete Geometry and Mathematical Mor-
phology, pages 255–268.
Decelle, R., Ngo, P., Debled-Rennesson, I., Mothe, F., and
Longuetaud, F. (2022). Ant colony optimization for
estimating pith position on images of tree log ends.
Image Processing On Line, 12:558–581.
Decelle, R., Ngo, P., Debled-Rennesson, I., Mothe, F., and
Longuetaud, F. (2023). Light u-net with a new mor-
phological attention gate model application to analyse
wood sections. In ICPRAM 2023.
Fabija
´
nska, A. and Danek, M. (2018). Deepdendro–a tree
rings detector based on a deep convolutional neural
network. Computers and electronics in agriculture,
150:353–363.
Gillert, A., Resente, G., Anadon-Rosell, A., Wilmking, M.,
and von Lukas, U. F. (2023). Iterative next bound-
ary detection for instance segmentation of tree rings
in microscopy images of shrub cross sections. In Pro-
ceedings of IEEE CVFPR, pages 14540–14548.
Gonzalez, R. C. (2009). Digital image processing. Pearson
education india.
He, K., Zhang, X., Ren, S., and Sun, J. (2016). Deep resid-
ual learning for image recognition. In Proceedings of
IEEE CCVPR, pages 770–778.
Kurdthongmee, W. (2020). A comparative study of the ef-
fectiveness of using popular dnn object detection al-
gorithms for pith detection in cross-sectional images
of parawood. Heliyon, 6(2).
Laggoune, H., Guesdon, V., et al. (2005). Tree ring analy-
sis. In IEEE Canadian Conference on Electrical and
Computer Engineering, pages 1574–1577.
Longuetaud, F., Pot, G., Mothe, F., Barthelemy, A., Decelle,
R., Delconte, F., Ge, X., Guillaume, G., Mancini, T.,
Ravoajanahary, T., et al. (2022). Traceability and qual-
ity assessment of douglas fir (pseudotsuga menziesii
(mirb.) franco) logs: the treetrace douglas database.
Annals of Forest Science, 79(1):1–21.
Makela, K., Ophelders, T., Quigley, M., Munch, E., Chit-
wood, D., and Dowtin, A. (2020). Automatic tree
ring detection using jacobi sets. arXiv preprint
arXiv:2010.08691.
Marichal, H., Passarella, D., Lucas, C., Profumo, L., Casar-
avilla, V., Rocha Galli, M. N., Ambite, S., and Ran-
dall, G. (2023a). UruDendro: An Uruguayan Disk
Wood Database For Image Processing.
Marichal, H., Passarella, D., and Randall, G. (2023b). Cs-
trd: a cross sections tree ring detection method. arXiv
preprint arXiv:2305.10809.
Norell, K. and Borgefors, G. (2008). Estimation of pith po-
sition in untreated log ends in sawmill environments.
Computers and Electronics in Agriculture, 63(2):155–
167.
Pol
´
a
ˇ
cek, M., Arizpe, A., H
¨
uther, P., Weidlich, L., Steindl,
S., and Swarts, K. (2023). Automation of tree-ring de-
tection and measurements using deep learning. Meth-
ods in Ecology and Evolution, 14(9):2233–2242.
Russakovsky, O., Deng, J., Su, H., Krause, J., Satheesh,
S., Ma, S., Huang, Z., Karpathy, A., Khosla, A.,
Bernstein, M., Berg, A. C., and Fei-Fei, L. (2015).
ImageNet Large Scale Visual Recognition Challenge.
International Journal of Computer Vision (IJCV),
115(3):211–252.
Venkatesh, M. and Seelamantula, C. S. (2015). Directional
bilateral filters. In Proceeding of IEEE ICASSP, pages
1578–1582.
Wimmer, G., Schraml, R., Hofbauer, H., Petutschnigg, A.,
and Uhl, A. (2021). Two-stage cnn-based wood log
recognition. In International Conference on Compu-
tational Science and Its Applications, pages 115–125.
Directional Filter for Tree Ring Detection
859