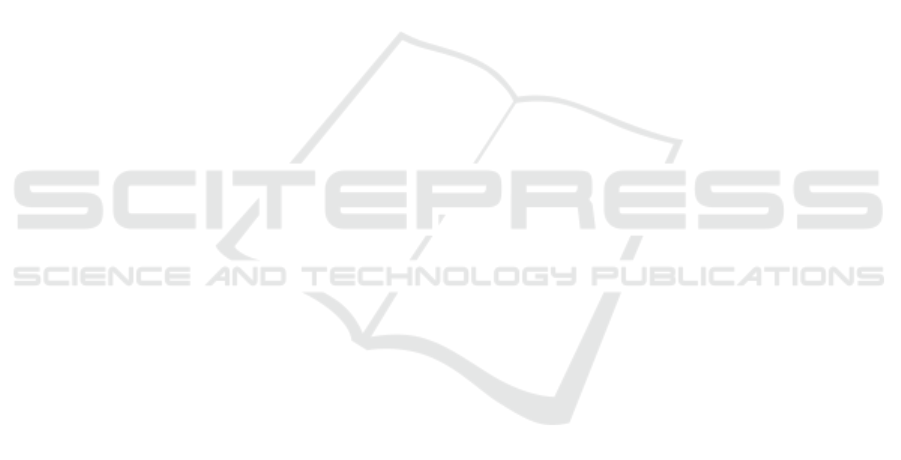
view. Journal of transport geography, 15(5):354–367.
Chwialkowska, A. (2019). How sustainability influencers
drive green lifestyle adoption on social media: The pro-
cess of green lifestyle adoption explained through the
lenses of the minority influence model and social learn-
ing theory. Management of Sustainable Development,
11(1):33–42.
Cialdini, R. B. and Jacobson, R. P. (2021). Influences of so-
cial norms on climate change-related behaviors. Current
Opinion in Behavioral Sciences, 42:1–8.
Clemen, T., Ahmady-Moghaddam, N., Lenfers, U. A.,
Ocker, F., Osterholz, D., Str
¨
obele, J., and Glake, D.
(2021). Multi-agent systems and digital twins for smarter
cities. In ACM SIGSIM-PADS 2021, pages 45–55.
Dauth, W. and Haller, P. (2018). Berufliches Pendeln zwis-
chen Wohn-und Arbeitsort: Klarer Trend zu l
¨
angeren
Pendeldistanzen. Technical report, IAB-Kurzbericht.
Davidsson, P. (2002). Agent based social simulation: A
computer science view. Journal of artificial societies and
social simulation, 5(1).
Dawes, R. M. (1980). Social dilemmas. Annual review of
psychology, 31(1):169–193.
Dittrich, P. and Kron, T. (2002). Complex reflexive agents
as models of social actors. In Proc. of the SICE Workshop
on Artificial Society/Organization/Economy, volume 25,
pages 79–88.
Doran, R. and Larsen, S. (2016). The relative importance
of social and personal norms in explaining intentions to
choose eco-friendly travel options. International Journal
of Tourism Research, 18(2):159–166.
Eriksson, L. and Forward, S. E. (2011). Is the intention to
travel in a pro-environmental manner and the intention
to use the car determined by different factors? Trans-
portation Research Part D: Transport and Environment,
16(5):372–376.
Ettema, D. and Nieuwenhuis, R. (2017). Residential self-
selection and travel behaviour: What are the effects of
attitudes, reasons for location choice and the built envi-
ronment? Journal of Transport Geography, 59:146–155.
Fan, Z., Yang, X., Yuan, W., Jiang, R., Chen, Q., Song, X.,
and Shibasaki, R. (2022). Online trajectory prediction for
metropolitan scale mobility digital twin. In SIGSPATIAL
2022, pages 1–12.
Ferreira, N., Poco, J., Vo, H. T., Freire, J., and Silva, C. T.
(2013). Visual exploration of big spatio-temporal ur-
ban data: A study of new york city taxi trips. IEEE
Transactions on Visualization and Computer Graphics,
19(12):2149–2158.
Granco, G., Heier Stamm, J. L., Bergtold, J. S., Daniels,
M. D., Sanderson, M. R., Sheshukov, A. Y., Mather,
M. E., Caldas, M. M., Ramsey, S. M., Lehrter II, R. J.,
Haukos, D. A., Gao, J., Chatterjee, S., Nifong, J. C., and
Aistrup, J. A. (2019). Evaluating environmental change
and behavioral decision-making for sustainability policy
using an agent-based model: A case study for the smoky
hill river watershed, kansas. Science of The Total Envi-
ronment, 695:133769.
Grieves, M. (2014). Digital Twin: Manufacturing Excel-
lence through Virtual Factory Replication. Technical re-
port, Michael W. Grieves, LLC.
Helbing, D. (2012). Social self-organization: Agent-based
simulations and experiments to study emergent social be-
havior. Springer.
Le Fur, J., Sall, M., and Dembele, J.-M. (2023). A Digital
Twin Simulator Approach as a Support to Develop an
Integrated Observatory of the Epidemic Risk in a Rural
Community in Senegal. In SIMULTECH 2023, pages
134–142. SCITEPRESS.
Leiserowitz, A., Carman, J., Buttermore, N., Neyens, L.,
Rosenthal, S., Marlon, J., Schneider, J., and Mulcahy, K.
(2022). International public opinion on climate change
2022.
Lorig, F., Lebherz, D. S., Berndt, J. O., and Timm, I. J.
(2017). Hypothesis-driven experiment design in com-
puter simulation studies. In WSC 2017, pages 1360–
1371. IEEE.
Major, P., Li, G., Hildre, H. P., and Zhang, H. (2021). The
use of a data-driven digital twin of a smart city: A case
study of
˚
alesund, norway. IEEE Instrumentation & Mea-
surement Magazine, 24(7):39–49.
Major, P., Torres, R., Amundsen, A., Stadsnes, P., et al.
(2022). On the use of graphical digital twins for urban
planning of mobility projects: a case study from a new
district in
˚
alesund, norway. In ECMS 2022.
Masson-Delmotte, V., Zhai, P., P
¨
ortner, H.-O., Roberts, D.,
Skea, J., Shukla, P. R., et al. (2022). Global Warming of
1.5 C: IPCC special report on impacts of global warming
of 1.5 C above pre-industrial levels in context of strength-
ening response to climate change, sustainable develop-
ment, and efforts to eradicate poverty. Cambridge Uni-
versity Press.
Mavrokapnidis, D., Mohammadi, N., and Taylor, J. (2021).
Community dynamics in smart city digital twins: A com-
puter vision-based approach for monitoring and forecast-
ing collective urban hazard exposure. In HICSS 2021.
Meta, I., Serra-Burriel, F., Carrasco-Jim
´
enez, J. C., Cucchi-
etti, F. M., Div
´
ı-Cuesta, C., Garc
´
ıa Calatrava, C., Garc
´
ıa,
D., Graells-Garrido, E., Navarro, G., L
`
azaro, Q., et al.
(2021). The camp nou stadium as a testbed for city phys-
iology: a modular framework for urban digital twins.
Complexity, 2021:1–15.
Mohammadi, N. and Taylor, J. (2019). Devising a Game
Theoretic Approach to Enable Smart City Digital Twin
Analytics. In HICCS 2019.
Mohammadi, N. and Taylor, J. (2020). Knowledge discov-
ery in smart city digital twins. In HICSS 2020.
National Academies of Sciences, E., Medicine, et al.
(2020). Climate change: evidence and causes up-
date 2020. The National Academies Press, Washington,
17226:25733.
Pan, X., Mohammadi, N., and Taylor, J. E. (2022). Smart
city digital twins for public safety: A deep learning
and simulation based method for dynamic sensing and
decision-making. In WSC 2022, pages 808–818.
Shukla, P. R., Skea, J., Calvo Buendia, E., Masson-
Delmotte, V., P
¨
ortner, H. O., Roberts, D., Zhai, P., Slade,
ICAART 2024 - 16th International Conference on Agents and Artificial Intelligence
394