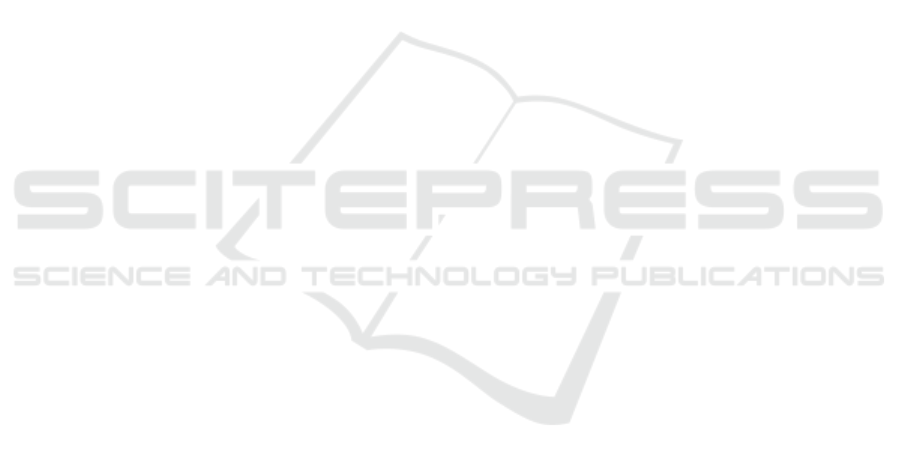
Chen, L., Wei, H., and Ferryman, J. (2013). A survey of
human motion analysis using depth imagery. Pattern
Recognition Letters, 34(15):1995–2006.
Dong, X., Garratt, M. A., Anavatti, S. G., and Abbass, H. A.
(2022). Mobilexnet: An efficient convolutional neu-
ral network for monocular depth estimation. IEEE
Transactions on Intelligent Transportation Systems,
23(11):20134–20147.
Eigen, D., Puhrsch, C., and Fergus, R. (2014). Depth map
prediction from a single image using a multi-scale
deep network. Advances in neural information pro-
cessing systems, 27.
Fu, H., Gong, M., Wang, C., Batmanghelich, K., and
Tao, D. (2018). Deep ordinal regression network
for monocular depth estimation. In Proceedings of
the IEEE conference on computer vision and pattern
recognition, pages 2002–2011.
Furukawa, R., Sagawa, R., and Kawasaki, H. (2017). Depth
estimation using structured light flow–analysis of pro-
jected pattern flow on an object’s surface. In Proceed-
ings of the IEEE International conference on com-
puter vision, pages 4640–4648.
Garg, R., Bg, V. K., Carneiro, G., and Reid, I. (2016). Unsu-
pervised cnn for single view depth estimation: Geom-
etry to the rescue. In Computer Vision–ECCV 2016:
14th European Conference, Amsterdam, The Nether-
lands, October 11-14, 2016, Proceedings, Part VIII
14, pages 740–756. Springer.
Godard, C., Mac Aodha, O., and Brostow, G. J. (2017).
Unsupervised monocular depth estimation with left-
right consistency. In Proceedings of the IEEE con-
ference on computer vision and pattern recognition,
pages 270–279.
Hao, Z., Li, Y., You, S., and Lu, F. (2018). Detail preserving
depth estimation from a single image using attention
guided networks. In 2018 International Conference
on 3D Vision (3DV), pages 304–313. IEEE.
Hirschmuller, H. and Scharstein, D. (2008). Evaluation of
stereo matching costs on images with radiometric dif-
ferences. IEEE transactions on pattern analysis and
machine intelligence, 31(9):1582–1599.
Laina, I., Rupprecht, C., Belagiannis, V., Tombari, F., and
Navab, N. (2016). Deeper depth prediction with fully
convolutional residual networks. In 2016 Fourth inter-
national conference on 3D vision (3DV), pages 239–
248. IEEE.
Li, B., Dai, Y., and He, M. (2018). Monocular depth es-
timation with hierarchical fusion of dilated cnns and
soft-weighted-sum inference. Pattern Recognition,
83:328–339.
Masoumian, A., Rashwan, H. A., Cristiano, J., Asif, M. S.,
and Puig, D. (2022). Monocular depth estimation us-
ing deep learning: A review. Sensors, 22(14):5353.
Mauri, A., Khemmar, R., Decoux, B., Benmoumen, T.,
Haddad, M., and Boutteau, R. (2021). A compara-
tive study of deep learning-based depth estimation ap-
proaches: Application to smart mobility. In 2021 8th
International Conference on Smart Computing and
Communications (ICSCC), pages 80–84. IEEE.
Murez, Z., Van As, T., Bartolozzi, J., Sinha, A., Badri-
narayanan, V., and Rabinovich, A. (2020). Atlas: End-
to-end 3d scene reconstruction from posed images. In
Computer Vision–ECCV 2020: 16th European Con-
ference, Glasgow, UK, August 23–28, 2020, Proceed-
ings, Part VII 16, pages 414–431. Springer.
Patil, V., Sakaridis, C., Liniger, A., and Van Gool, L. (2022).
P3depth: Monocular depth estimation with a piece-
wise planarity prior. In Proceedings of the IEEE/CVF
Conference on Computer Vision and Pattern Recogni-
tion, pages 1610–1621.
Paul, S., Jhamb, B., Mishra, D., and Kumar, M. S. (2022).
Edge loss functions for deep-learning depth-map. Ma-
chine Learning with Applications, 7:100218.
Sikder, A. K., Petracca, G., Aksu, H., Jaeger, T., and Ulu-
agac, A. S. (2021). A survey on sensor-based threats
and attacks to smart devices and applications. IEEE
Communications Surveys & Tutorials, 23(2):1125–
1159.
Silberman, N., Hoiem, D., Kohli, P., and Fergus, R. (2012).
Indoor segmentation and support inference from rgbd
images. In Computer Vision–ECCV 2012: 12th Euro-
pean Conference on Computer Vision, Florence, Italy,
October 7-13, 2012, Proceedings, Part V 12, pages
746–760. Springer.
Tang, S., Tan, F., Cheng, K., Li, Z., Zhu, S., and Tan, P.
(2019). A neural network for detailed human depth
estimation from a single image. In Proceedings of the
IEEE/CVF International Conference on Computer Vi-
sion, pages 7750–7759.
Torralba, A. and Oliva, A. (2002). Depth estimation from
image structure. IEEE Transactions on pattern analy-
sis and machine intelligence, 24(9):1226–1238.
Wang, Z., Bovik, A. C., Sheikh, H. R., and Simoncelli, E. P.
(2004). Image quality assessment: from error visi-
bility to structural similarity. IEEE transactions on
image processing, 13(4):600–612.
Yan, S., Yang, J., Leonardis, A., and K
¨
am
¨
ar
¨
ainen, J. (2021).
Depth-only object tracking. In British Machine Vision
Conference.
Yang, Y., Wang, Y., Zhu, C., Zhu, M., Sun, H., and Yan, T.
(2021). Mixed-scale unet based on dense atrous pyra-
mid for monocular depth estimation. IEEE Access,
9:114070–114084.
Yue, H., Zhang, J., Wu, X., Wang, J., and Chen, W. (2020).
Edge enhancement in monocular depth prediction. In
2020 15th IEEE Conference on Industrial Electronics
and Applications (ICIEA), pages 1594–1599. IEEE.
Zhao, C., Sun, Q., Zhang, C., Tang, Y., and Qian, F. (2020).
Monocular depth estimation based on deep learning:
An overview. Science China Technological Sciences,
63(9):1612–1627.
Depth Estimation Using Weighted-Loss and Transfer Learning
787