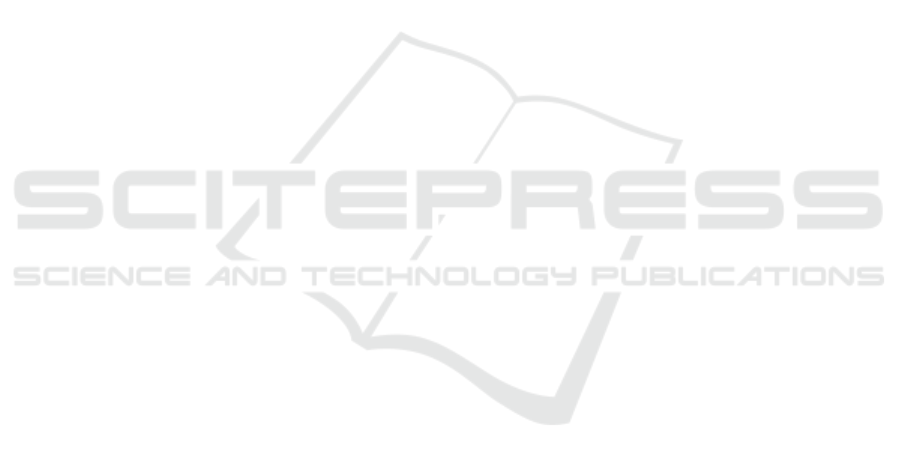
Ershov, E., Savchik, A., Shepelev, D., Bani
´
c, N., Brown,
M. S., Timofte, R., Ko
ˇ
s
ˇ
cevi
´
c, K., Freeman, M.,
Tesalin, V., Bocharov, D., et al. (2022). NTIRE 2022
Challenge on Night Photography Rendering. In Proc.
of the IEEE/CVF Conf. on Cmput. Vis. and Pattern
Recogn., pages 1287–1300.
Ghadiyaram, D. and Bovik, A. C. (2015). Massive On-
line Crowdsourced Study of Subjective and Objective
Picture Quality. IEEE Trans. on Image Processing,
25(1):372–387.
Handley, J. C. (2001). Comparative analysis of bradley-
terry and thurstone-mosteller paired comparison mod-
els for image quality assessment. In PICS, volume 1,
pages 108–112.
Hosu, V., Lin, H., and Saupe, D. (2018). Expertise screen-
ing in crowdsourcing image quality. In 2018 Tenth
international conference on quality of multimedia ex-
perience (QoMEX), pages 1–6. IEEE.
Hosu, V., Lin, H., Sziranyi, T., and Saupe, D. (2020).
Koniq-10k: An ecologically valid database for deep
learning of blind image quality assessment. IEEE
Trans. on Image Processing, 29:4041–4056.
Joglekar, M., Garcia-Molina, H., and Parameswaran, A.
(2013). Evaluating the Crowd with Confidence. In
Proc. of the 19th ACM SIGKDD intern. conf. on
Knowledge discovery and data mining, pages 686–
694.
Keimel, C., Habigt, J., Horch, C., and Diepold, K. (2012).
Qualitycrowd—a framework for crowd-based quality
evaluation. In 2012 Picture coding symposium, pages
245–248. IEEE.
Ko, K., Lee, J.-T., and Kim, C.-S. (2018). PCC-Net: Pair-
wise Aesthetic Comparison Network for Image Aes-
thetic Assessment. In 2018 25th IEEE International
Conf. on Image Processing (ICIP), pages 2491–2495.
IEEE.
Lin, H., Hosu, V., and Saupe, D. (2019). KADID-10k: A
Large-scale Artificially Distorted IQA Database. In
2019 Tenth International Conf. on Quality of Multi-
media Experience (QoMEX), pages 1–3. IEEE.
Lin, H., Hosu, V., and Saupe, D. (2020). DeepFL-IQA:
Weak Supervision for Deep IQA Feature Learning.
arXiv preprint arXiv:2001.08113.
Luce, R. D. (2012). Individual choice behavior: A theoret-
ical analysis. Courier Corporation.
Ma, K., Liu, W., Zhang, K., Duanmu, Z., Wang, Z., and
Zuo, W. (2017). End-to-End Blind Image Quality As-
sessment Using Deep Neural Networks. IEEE Trans.
on Image Processing, 27(3):1202–1213.
Ma, K., Wu, Q., Wang, Z., Duanmu, Z., Yong, H., Li, H.,
and Zhang, L. (2016). Group MAd Competition - A
New Methodology to Compare Objective Image Qual-
ity Models. In Proc. of the IEEE Conf. on Cmput. Vis.
and Pattern Recogn., pages 1664–1673.
Madhusudana, P. C., Birkbeck, N., Wang, Y., Adsumilli, B.,
and Bovik, A. C. (2022). Image Quality Assessment
Using Contrastive Learning. IEEE Trans. on Image
Processing, 31:4149–4161.
Mantiuk, R. K., Tomaszewska, A., and Mantiuk, R. (2012).
Comparison of Four Subjective Methods for Image
Quality Assessment. In Computer graphics forum,
volume 31, pages 2478–2491. Wiley Online Library.
Marcus, A., Parameswaran, A., et al. (2015). Crowdsourced
Data Management: Industry and Academic Perspec-
tives. Found. and Trends in Databases, 6(1-2):1–161.
Mohammadi, P., Ebrahimi-Moghadam, A., and Shirani, S.
(2014). Subjective and objective quality assessment
of image: A survey. arXiv preprint arXiv:1406.7799.
Pfister, J., Kobs, K., and Hotho, A. (2021). Self-Supervised
Multi-Task Pretraining Improves Image Aesthetic As-
sessment. In Proc. of the IEEE/CVF Conf. on Cmput.
Vis. and Pattern Recogn., pages 816–825.
Pugach, C., Leder, H., and Graham, D. J. (2017). How sta-
ble are human aesthetic preferences across the lifes-
pan? Frontiers in human neuroscience, 11:289.
Ribeiro, F., Flor
ˆ
encio, D., Zhang, C., and Seltzer, M.
(2011). Crowdmos: An approach for crowdsourcing
mean opinion score studies. In 2011 IEEE interna-
tional conference on acoustics, speech and signal pro-
cessing (ICASSP), pages 2416–2419. IEEE.
Ruikar, J. and Chaudhury, S. (2023). NITS-IQA Database:
A New Image Quality Assessment Database. Sensors,
23(4):2279.
Sheikh, H. R., Sabir, M. F., and Bovik, A. C. (2006). A
Statistical Evaluation of Recent Full Reference Image
Quality Assessment Algorithms. IEEE Trans. on im-
age processing, 15(11):3440–3451.
Shutova, A., Ershov, E., Perevozchikov, G., Ermakov, I.,
Bani
´
c, N., Timofte, R., Collins, R., Efimova, M.,
Terekhin, A., Zini, S., et al. (2023). NTIRE 2022
Challenge on Night Photography Rendering. In Proc.
of the IEEE/CVF Conf. on Cmput. Vis. and Pattern
Recogn., pages 1981–1992.
Siahaan, E., Hanjalic, A., and Redi, J. (2016). A Reli-
able Methodology to Collect Ground Truth Data of
Image Aesthetic Appeal. IEEE Trans. on Multimedia,
18(7):1338–1350.
Talebi, H. and Milanfar, P. (2018). NIMA: Neural Im-
age Assessment. IEEE Trans. on Image Processing,
27(8):3998–4011.
Thurstone, L. L. (1927). A law of comparative judgment.
Psychological review, 34(4):273.
Wang, Z., Bovik, A. C., and Lu, L. (2002). Why is image
quality assessment so difficult? In 2002 IEEE Inter-
national conference on acoustics, speech, and signal
processing, volume 4, pages IV–3313. IEEE.
Ying, Z., Niu, H., Gupta, P., Mahajan, D., Ghadiyaram,
D., and Bovik, A. (2020). From Patches to Pictures
(PaQ-2-PiQ): Mapping the Perceptual Space of Pic-
ture Quality. In Proc. of the IEEE/CVF Conf. on Cm-
put. Vis. and Pattern Recogn., pages 3575–3585.
Zaric, A., Loncaric, M., Tralic, D., Brzica, M., Dumic,
E., and Grgic, S. (2010). Image quality assessment-
comparison of objective measures with results of sub-
jective test. In Proc. ELMAR-2010, pages 113–118.
IEEE.
Zhai, G. and Min, X. (2020). Perceptual image quality as-
sessment: a survey. Science China Information Sci-
ences, 63:1–52.
VISAPP 2024 - 19th International Conference on Computer Vision Theory and Applications
372