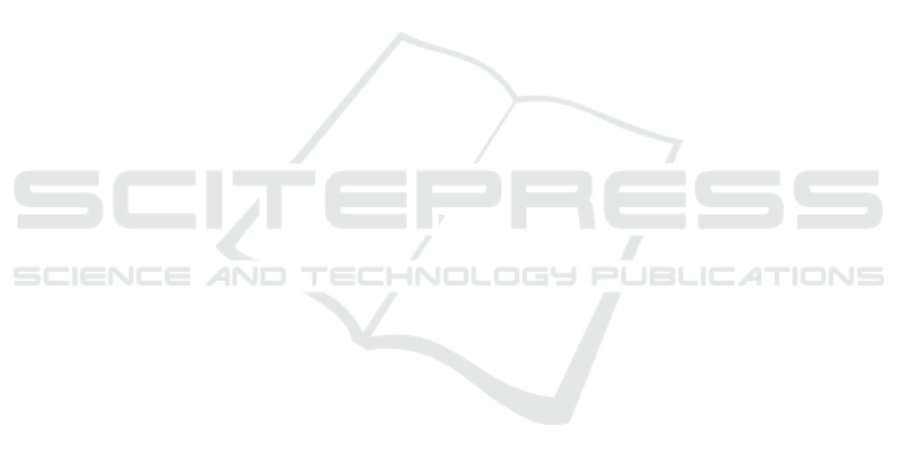
Hildmann, H., Kovacs, E., Saffre, F., and Isakovic, A.
(2019). Nature-inspired drone swarming for real-time
aerial data-collection under dynamic operational con-
straints. Drones, 3(3):71.
Hunjet, R., Fraser, B., Stevens, T., Hodges, L., Mayen,
K., Barca, J. C., Cochrane, M., Cannizzaro, R., and
Palmer, J. L. (2018). Data ferrying with swarming uas
in tactical defence networks. In 2018 IEEE interna-
tional conference on robotics and automation (ICRA),
pages 6381–6388. IEEE.
Jafari, M. and Xu, H. (2018). Biologically-inspired intel-
ligent flocking control for networked multi-uas with
uncertain network imperfections. Drones, 2(4):33.
Jahanshahi, M. R., Shen, W.-M., Mondal, T. G., Abdelbarr,
M., Masri, S. F., and Qidwai, U. A. (2017). Recon-
figurable swarm robots for structural health monitor-
ing: a brief review. International Journal of Intelligent
Robotics and Applications, 1:287–305.
Jurt, M., Milner, E., Sooriyabandara, M., and Hauert, S.
(2022). Collective transport of arbitrarily shaped ob-
jects using robot swarms. Artificial Life and Robotics,
27(2):365–372.
Li, S., Jia, Y., Yang, F., Qin, Q., Gao, H., and Zhou, Y.
(2022). Collaborative decision-making method for
multi-uav based on multiagent reinforcement learn-
ing. IEEE Access, 10:91385–91396.
Liekna, A., Grundspenkis, J., et al. (2014). Towards practi-
cal application of swarm robotics: overview of swarm
tasks. Engineering for rural development, 13:271–
277.
Majd, A., Loni, M., Sahebi, G., and Daneshtalab, M.
(2020). Improving motion safety and efficiency of
intelligent autonomous swarm of drones. Drones,
4(3):48.
Mnih, V., Kavukcuoglu, K., Silver, D., Rusu, A. A., Ve-
ness, J., Bellemare, M. G., Graves, A., Riedmiller, M.,
Fidjeland, A. K., Ostrovski, G., et al. (2015). Human-
level control through deep reinforcement learning. na-
ture, 518(7540):529–533.
Mor
´
en, J. and Balkenius, C. (2000). A computational model
of emotional learning in the amygdala. From animals
to animats, 6:115–124.
Pina, R., De Silva, V., Hook, J., and Kondoz, A. (2022).
Residual q-networks for value function factorizing in
multiagent reinforcement learning. IEEE Transac-
tions on Neural Networks and Learning Systems.
Qu, C., Boubin, J., Gafurov, D., Zhou, J., Aloysius, N.,
Nguyen, H., and Calyam, P. (2022). Uav swarms
in smart agriculture: Experiences and opportunities.
In 2022 IEEE 18th International Conference on e-
Science (e-Science), pages 148–158. IEEE.
Rashid, T., Samvelyan, M., De Witt, C. S., Farquhar, G., Fo-
erster, J., and Whiteson, S. (2020). Monotonic value
function factorisation for deep multi-agent reinforce-
ment learning. The Journal of Machine Learning Re-
search, 21(1):7234–7284.
Revill, M. B. (2016). UAV swarm behavior modeling for
early exposure of failure modes. PhD thesis, Mon-
terey, California: Naval Postgraduate School.
Rodriguez, I., Mogensen, R. S., Schjørring, A., Razza-
ghpour, M., Maldonado, R., Berardinelli, G., Adeo-
gun, R., Christensen, P. H., Mogensen, P., Madsen,
O., et al. (2021). 5g swarm production: Advanced
industrial manufacturing concepts enabled by wire-
less automation. IEEE Communications Magazine,
59(1):48–54.
Sunehag, P., Lever, G., Gruslys, A., Czarnecki, W. M.,
Zambaldi, V., Jaderberg, M., Lanctot, M., Sonnerat,
N., Leibo, J. Z., Tuyls, K., et al. (2017). Value-
decomposition networks for cooperative multi-agent
learning. arXiv preprint arXiv:1706.05296.
Sutton, R. S. and Barto, A. G. (2018). Reinforcement learn-
ing: An introduction. MIT press.
Tan, M. (1993). Multi-agent reinforcement learning: Inde-
pendent vs. cooperative agents. In Proceedings of the
tenth international conference on machine learning,
pages 330–337.
Thumiger, N. and Deghat, M. (2021). A multi-agent deep
reinforcement learning approach for practical decen-
tralized uav collision avoidance. IEEE Control Sys-
tems Letters, 6:2174–2179.
Watkins, C. J. and Dayan, P. (1992). Q-learning. Machine
learning, 8:279–292.
Zhang, X., Bai, Y., and He, K. (2023). On countermea-
sures against cooperative fly of uav swarms. Drones,
7(3):172.
Towards Self-Adaptive Resilient Swarms Using Multi-Agent Reinforcement Learning
417