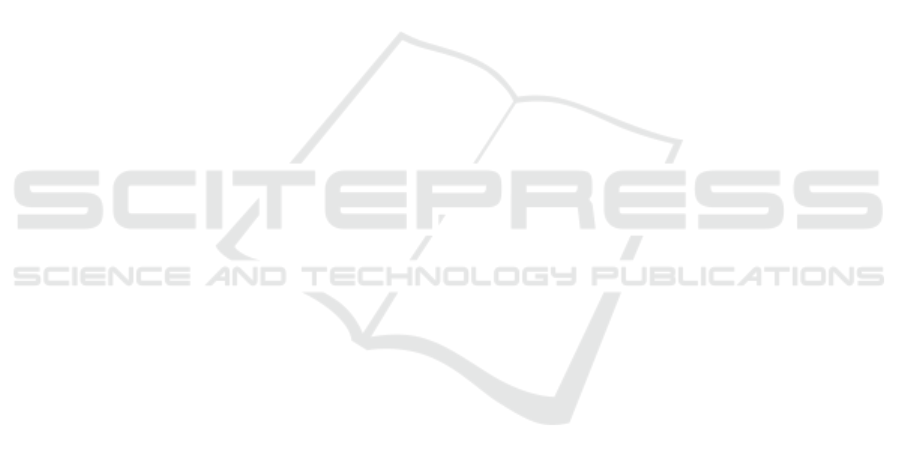
kimgs. We also plan to experiment with different im-
age patch resolutions and WSI zoom levels.
The following research will analyse the Ki67 in-
dex captured in StyleGAN latent space and the rela-
tionship between H&E and Ki67 staining. We expect
that the Ki67 information improves the structure of
latent space.
In our future research, we will train a model for re-
verse mapping to StyleGAN latent space. The model
will estimate a latent vector and Ki67 index of real
H&E image. Mapping real H&E images to latent
space will enable different experiments with Style-
GAN model, e.g., to generate counterfactual exam-
ples as an explanation of Ki67 index prediction. We
can modify an input image’s Ki67 index or latent
vector and investigate StyleGAN’s outputs. Another
experiment can analyse linear interpolation in latent
space, similarly to (Daroach et al., 2021). These ex-
periments can potentially unveil concealed associa-
tions between H&E and Ki67 tissue staining, expand-
ing scientific knowledge.
ACKNOWLEDGEMENTS
This research was supported by the Ministry of Ed-
ucation, Science, Research and Sport of the Slovak
Republic under the contract No. VEGA 1/0369/22.
Gratitude is extended to K. Tobi
´
a
ˇ
sov
´
a for her assess-
ment of generated images and to L. Plank and K.
Tobi
´
a
ˇ
sov
´
a for their collaboration in the preparation of
the dataset.
REFERENCES
Brock, A., Donahue, J., and Simonyan, K. (2018). Large
scale gan training for high fidelity natural image syn-
thesis. arXiv preprint arXiv:1809.11096.
Daroach, G. B., Yoder, J. A., Iczkowski, K. A., and LaVi-
olette, P. S. (2021). High-resolution controllable pro-
static histology synthesis using stylegan. BIOIMAG-
ING, 11.
Goodfellow, I., Pouget-Abadie, J., Mirza, M., Xu, B.,
Warde-Farley, D., Ozair, S., Courville, A., and Ben-
gio, Y. (2014). Generative adversarial nets. In Ghahra-
mani, Z., Welling, M., Cortes, C., Lawrence, N., and
Weinberger, K., editors, Advances in Neural Infor-
mation Processing Systems, volume 27. Curran Asso-
ciates, Inc.
Gurcan, M. N., Boucheron, L. E., Can, A., Madabhushi, A.,
Rajpoot, N. M., and Yener, B. (2009). Histopatho-
logical image analysis: A review. IEEE reviews in
biomedical engineering, 2:147–171.
Heusel, M., Ramsauer, H., Unterthiner, T., Nessler, B., and
Hochreiter, S. (2017). Gans trained by a two time-
scale update rule converge to a local nash equilibrium.
Advances in neural information processing systems,
30.
Karras, T., Aittala, M., Laine, S., H
¨
ark
¨
onen, E., Hellsten, J.,
Lehtinen, J., and Aila, T. (2021). Alias-free generative
adversarial networks. In Proc. NeurIPS.
Karras, T., Laine, S., and Aila, T. (2019). A style-based
generator architecture for generative adversarial net-
works. In Proceedings of the IEEE/CVF conference
on computer vision and pattern recognition, pages
4401–4410.
Karras, T., Laine, S., Aittala, M., Hellsten, J., Lehtinen,
J., and Aila, T. (2020). Analyzing and improving
the image quality of stylegan. In Proceedings of the
IEEE/CVF conference on computer vision and pattern
recognition, pages 8110–8119.
Mirza, M. and Osindero, S. (2014). Conditional generative
adversarial nets. arXiv preprint arXiv:1411.1784.
NVlabs (april 2023). Alias-free generative adversarial net-
works (stylegan3) - official pytorch implementation of
the neurips 2021 paper.
Petr
´
ıkov
´
a, D., Cimr
´
ak, I., Tobi
´
a
ˇ
sov
´
a, K., and Plank, L.
(2023). Semi-automated workflow for computer-
generated scoring of Ki67 positive cells from he
stained slides. In BIOINFORMATICS, pages 292–
300.
Quiros, A. C., Murray-Smith, R., and Yuan, K. (2019).
Pathologygan: Learning deep representations of can-
cer tissue. arXiv preprint arXiv:1907.02644.
Regulation, G. D. P. (2020). Art. 22 gdpr. automated indi-
vidual decision-making, including profiling. Intersoft
Consulting, https://gdpr-info. eu/art-22-gdpr.
Schutte, K., Moindrot, O., H
´
erent, P., Schiratti, J.-B., and
J
´
egou, S. (2021). Using stylegan for visual inter-
pretability of deep learning models on medical im-
ages. arXiv preprint arXiv:2101.07563.
Wittekind, D. (2003). Traditional staining for routine diag-
nostic pathology including the role of tannic acid. 1.
value and limitations of the hematoxylin-eosin stain.
Biotechnic & histochemistry, 78(5):261–270.
BIOINFORMATICS 2024 - 15th International Conference on Bioinformatics Models, Methods and Algorithms
518