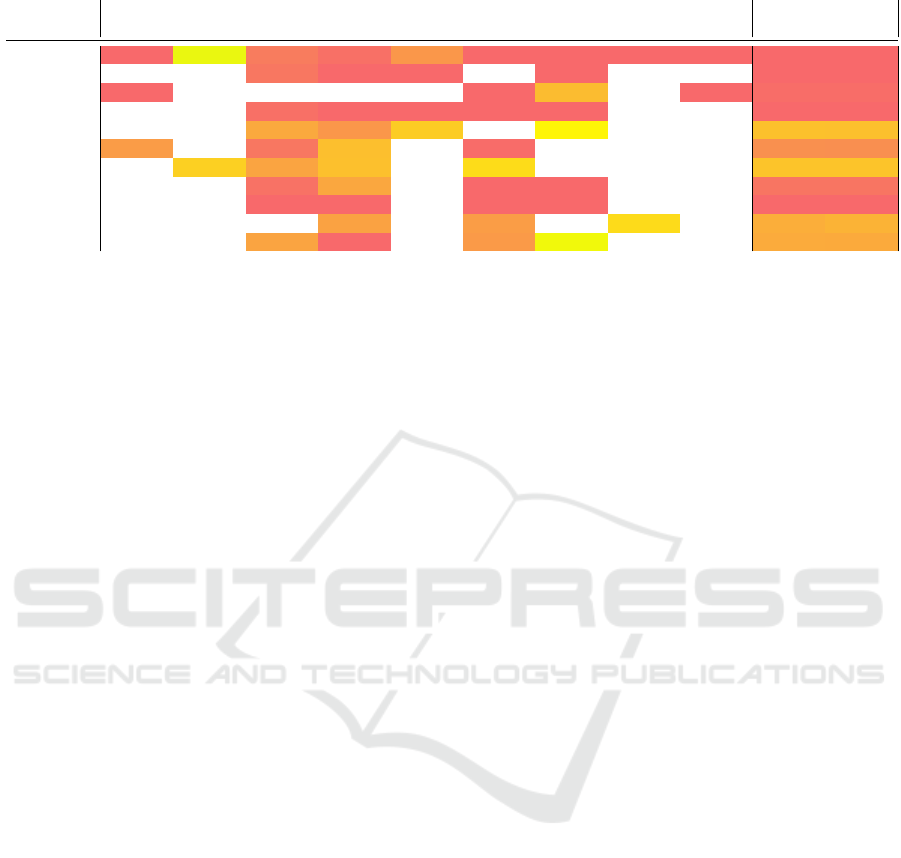
Table 6: AUC of the Handcrafted features model.
Bent Color Comb. Contam. Crack Cut Hole Open Thread Test Test
Avg
all 0.35 0.78 0.53 0.51 0.58 0.48 0.48 0.30 0.13 0.49 0.46
bagel 0.52 0.44 0.48 0.48 0.48 0.48
cable gland 0.47 0.49 0.64 0.43 0.51 0.51
carrot 0.51 0.42 0.46 0.49 0.35 0.45 0.45
cookie 0.61 0.58 0.67 0.73 0.65 0.65
dowel 0.59 0.52 0.64 0.51 0.57 0.56
foam 0.67 0.60 0.65 0.69 0.65 0.65
peach 0.52 0.60 0.48 0.48 0.52 0.52
potato 0.00 0.00 0.00 0.00 0.00 0.00
rope 0.60 0.59 0.69 0.62 0.62
tire 0.60 0.48 0.58 0.77 0.61 0.61
anomaly detection and localization. In Proceedings of
the 17th International Joint Conference on Computer
Vision, Imaging and Computer Graphics Theory and
Applications - Volume 5: VISAPP,, pages 202–213.
INSTICC, SciTePress.
Birnbaum, Z. et al. (2015). Unmanned aerial vehicle secu-
rity using behavioral profiling. In 2015 International
Conference on Unmanned Aircraft Systems (ICUAS),
pages 1310–1319.
Chandola, V., Banerjee, A., and Kumar, V. (2009).
Anomaly detection: A survey. ACM Comput. Surv.,
41(3).
Christiansen, P. et al. (2016). Deepanomaly: Combining
background subtraction and deep learning for detect-
ing obstacles and anomalies in an agricultural field.
Sensors, 16(11):1904.
Chu, Y.-M., Liu, C., Hsieh, T.-I., Chen, H.-T., and Liu, T.-L.
(2023). Shape-guided dual-memory learning for 3D
anomaly detection. In Krause, A., Brunskill, E., Cho,
K., Engelhardt, B., Sabato, S., and Scarlett, J., editors,
Proceedings of the 40th International Conference on
Machine Learning, volume 202 of Proceedings of Ma-
chine Learning Research, pages 6185–6194. PMLR.
Dinh, L., Sohl-Dickstein, J., and Bengio, S. (2016). Density
estimation using real nvp.
Dong, R., Qi, Z., Zhang, L., Zhang, J., Sun, J., Ge, Z., Yi,
L., and Ma, K. (2023). Autoencoders as cross-modal
teachers: Can pretrained 2d image transformers help
3d representation learning? In The Eleventh Interna-
tional Conference on Learning Representations.
Dosovitskiy, A., Beyer, L., Kolesnikov, A., Weissenborn,
D., Zhai, X., Unterthiner, T., Dehghani, M., Min-
derer, M., Heigold, G., Gelly, S., Uszkoreit, J., and
Houlsby, N. (2021). An Image is Worth 16x16
Words: Transformers for Image Recognition at Scale.
arXiv:2010.11929 [cs].
Floris, A., Frittoli, L., Carrera, D., and Boracchi, G. (2022).
Composite layers for deep anomaly detection on 3d
point clouds.
Frittoli, L. (2022). ADVANCED LEARNING METHODS
FOR ANOMALY DETECTION IN MULTIVARIATE
DATASTREAMS AND POINT CLOUDS. PhD thesis,
Politecnico Milano.
Haselmann, M., Gruber, D. P., and Tabatabai, P. (2018).
Anomaly detection using deep learning based im-
age completion. In 2018 17th IEEE International
Conference on Machine Learning and Applications
(ICMLA), pages 1237–1242.
Horwitz, E. and Hoshen, Y. (2023). Back to the Feature:
Classical 3D Features Are (Almost) All You Need for
3D Anomaly Detection. In IEEE/CVF Conference
on Computer Vision and Pattern Recognition, pages
2967–2976.
Khalastchi, E., Kalech, M., Kaminka, G. A., and Lin, R.
(2015). Online data-driven anomaly detection in au-
tonomous robots. Knowledge and Information Sys-
tems, 43(3):657–688.
Kingma, D. P. and Ba, J. (2014). Adam: A
method for stochastic optimization. arXiv preprint
arXiv:1412.6980.
Mantegazza, D., Giusti, A., Gambardella, L. M., and Guzzi,
J. (2022). An outlier exposure approach to im-
prove visual anomaly detection performance for mo-
bile robots. IEEE Robotics and Automation Letters,
pages 1–8.
Mantegazza, D., Xhyra, A., Giusti, A., and Guzzi, J. (2023).
Active Anomaly Detection for Autonomous Robots:
A Benchmark. In Iida, F., Maiolino, P., Abdulali, A.,
and Wang, M., editors, Towards Autonomous Robotic
Systems, Lecture Notes in Computer Science, pages
315–327, Cham. Springer Nature Switzerland.
Masuda, M., Hachiuma, R., Fujii, R., Saito, H., and
Sekikawa, Y. (2021). Toward unsupervised 3d point
cloud anomaly detection using variational autoen-
coder. In 2021 IEEE International Conference on Im-
age Processing (ICIP), pages 3118–3122.
Radford, A., Kim, J. W., Hallacy, C., Ramesh, A., Goh, G.,
Agarwal, S., Sastry, G., Askell, A., Mishkin, P., Clark,
J., Krueger, G., and Sutskever, I. (2021). Learning
Transferable Visual Models From Natural Language
Supervision. arXiv:2103.00020 [cs].
Rudolph, M., Wehrbein, T., Rosenhahn, B., and Wandt,
B. (2023). Asymmetric Student-Teacher Networks
for Industrial Anomaly Detection. In Proceedings of
the IEEE/CVF Winter Conference on Applications of
Computer Vision (WACV), pages 2592–2602.
Ruff, L. et al. (2021). A unifying review of deep and shal-
low anomaly detection. Proceedings of the IEEE,
109(5):756–795.
Schlegl, T. et al. (2017). Unsupervised anomaly detec-
Detecting Anomalous 3D Point Clouds Using Pre-Trained Feature Extractors
379