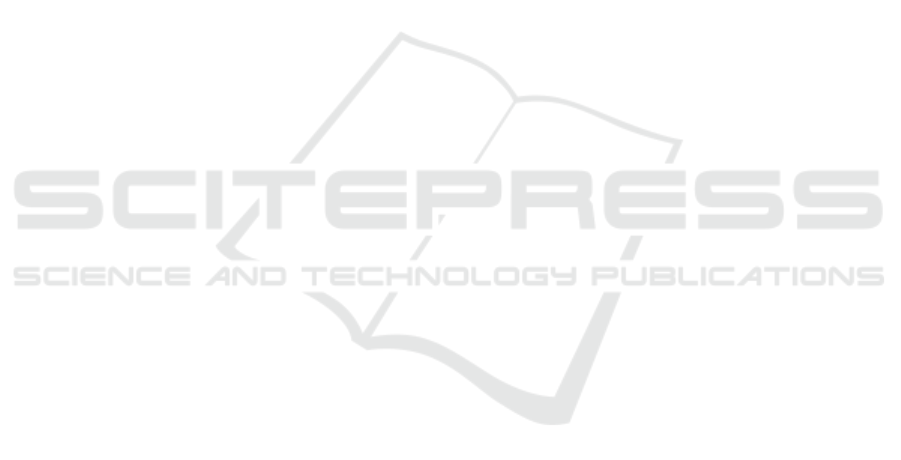
REFERENCES
Agrawal, R., Imieli
´
nski, T., and Swami, A. (1993). Min-
ing association rules between sets of items in large
databases. ACM SIGMOD Record, 22:207–216.
Agrawal, R. and Srikant, R. (1994). Fast algorithms
for mining association rules in large databases. In
Proceedings of the 20th International Conference on
Very Large Data Bases, page 487–499. Morgan Kauf-
mann Publishers Inc.
Baesens, B., Viaene, S., and Vanthienen, J. (2000). Post-
processing of association rules. In KDD ’00: Proceed-
ings of the Sixth ACM SIGKDD International Confer-
ence on Knowledge Discovery and Data Mining. Spe-
cial workshop on post-processing in machine learning
and data mining: interpretation, visualization, integra-
tion, and related topics. Association for Computing
Machinery.
Balasubramani, B. S., Shivaprabhu, V. R., Krishnamurthy,
S., Cruz, I. F., and Malik, T. (2016). Ontology-based
urban data exploration. In Proceedings of the 2nd
ACM SIGSPATIAL Workshop on Smart Cities and
Urban Analytics - UrbanGIS ’16, pages 1–8. ACM
Press.
Bayardo, R. J., Agrawal, R., and Gunopulos, D.
(2000). Constraint-based rule mining in large, dense
databases. Data Mining and Knowledge Discovery,
4:217–240.
Berka, P. (2018). Comprehensive concept description
based on association rules: A meta-learning approach.
Intelligent Data Analysis, 22:325–344.
Castro, G., Salvini, R., Soares, F. A., Nierenberg, A. A.,
Sachs, G. S., Lafer, B., and Dias, R. S. (2018). Ap-
plying Association Rules to Study Bipolar Disorder
and Premenstrual Dysphoric Disorder Comorbidity.
In 2018 IEEE Canadian Conference on Electrical &
Computer Engineering (CCECE), pages 1–4. IEEE.
Cheng, C.-W., Sha, Y., and Wang, M. D. (2016). Inter-
visar: An interactive visualization for association rule
search. In Proceedings of the 7th ACM International
Conference on Bioinformatics, Computational Biol-
ogy, and Health Informatics, pages 175–184. ACM.
de Padua, R., do Carmo, L. P., Rezende, S. O., and de Car-
valho, V. O. (2018). An analysis on community detec-
tion and clustering algorithms on the post-processing
of association rules. In 2018 International Joint Con-
ference on Neural Networks (IJCNN), volume 2018-
July, pages 1–7. IEEE.
Freitas, A. A. (2000). Understanding the crucial differ-
ences between classification and discovery of associ-
ation rules. ACM SIGKDD Explorations Newsletter,
2:65–69.
Hagberg, A. A., Schult, D. A., and Swart, P. J. (2008). Ex-
ploring network structure, dynamics, and function us-
ing networkx. In Varoquaux, G., Vaught, T., and Mill-
man, J., editors, Proceedings of the 7th Python in Sci-
ence Conference, pages 11 – 15, Pasadena, CA USA.
Hahsler, M. (2023). R package arules - min-
ing association rules and frequent itemsets.
https://www.rdocumentation.org/packages/arules/ver
sions/1.7-6. Accessed: 2023-08-05.
Hahsler, M. and Karpienko, R. (2017). Visualizing associa-
tion rules in hierarchical groups. Journal of Business
Economics, 87:317–335.
Han, J., Kamber, M., and Pei, J. (2012). Data Mining: Con-
cepts and Techniques. Elsevier Inc.
Han, J., Pei, J., Yin, Y., and Mao, R. (2004). Min-
ing frequent patterns without candidate generation:
A frequent-pattern tree approach. Data Mining and
Knowledge Discovery, 8:53–87.
Karimi-Majd, A.-M. and Mahootchi, M. (2015). A new
data mining methodology for generating new service
ideas. Information Systems and e-Business Manage-
ment, 13:421–443.
Kwon, J.-H. and Kim, E.-J. (2019). Accident prediction
model using environmental sensors for industrial in-
ternet of things. Sensors and Materials, 31:579.
Matetic, M., Bakaric, M. B., and Sisovic, S. (2015). Asso-
ciation rule mining and visualization of introductory
programming course activities. In Proceedings of the
16th International Conference on Computer Systems
and Technologies - CompSysTech ’15, volume 1008,
pages 374–381. ACM Press.
NetworkX Developers (2022). Net-
workx - network analysis in python.
https://networkx.org/documentation/networkx-2.8.8/.
Accessed: 2023-08-05.
Shinn, M. (2016). Forceatlas2 for python.
https://github.com/mwshinn/forceatlas2-python/.
Accessed: 2023-08-05.
Slyepchenko, A., Frey, B. N., Lafer, B., Nierenberg, A. A.,
Sachs, G. S., and Dias, R. S. (2017). Increased ill-
ness burden in women with comorbid bipolar and pre-
menstrual dysphoric disorder: data from 1 099 women
from STEP-BD study. Acta Psychiatrica Scandinav-
ica, 136(5):473–482.
Wei, L. and Scott, J. (2015). Association rule mining in
the us vaccine adverse event reporting system (vaers).
Pharmacoepidemiology and Drug Safety, 24:922–
933.
Weidner, D., Atzmueller, M., and Seipel, D. (2020).
Finding Maximal Non-redundant Association Rules
in Tennis Data, volume 12057 LNAI, pages 59–78.
Springer.
Zaki, M. (2000). Scalable algorithms for association min-
ing. IEEE Transactions on Knowledge and Data En-
gineering, 12:372–390.
Zhang, C., Xue, X., Zhao, Y., Zhang, X., and Li, T. (2019).
An improved association rule mining-based method
for revealing operational problems of building heat-
ing, ventilation and air conditioning (hvac) systems.
Applied Energy, 253:113492.
Zhang, C., Zhao, Y., Lu, J., Li, T., and Zhang, X. (2021).
Analytic hierarchy process-based fuzzy post mining
method for operation anomaly detection of building
energy systems. Energy and Buildings, 252:111426.
ICPRAM 2024 - 13th International Conference on Pattern Recognition Applications and Methods
130