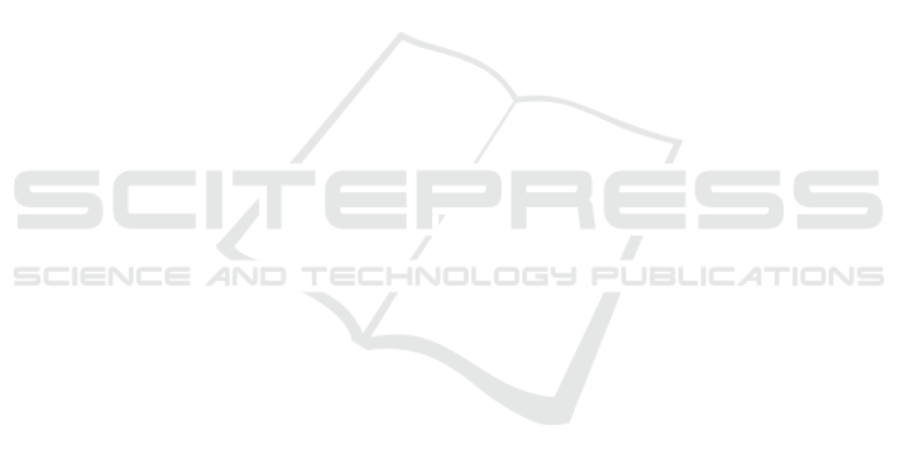
better management of services. We also integrated
the IT1FL model as a decision-making knowledge
representation to detect congestion situations due to
warning events. It enables handling and modeling un-
certainty and makes our system more robust in real-
world scenarios. To prove the validation of our ap-
proach, we elaborate on a scenario of an accident
warning. Our approach outperforms mean travel time
and mean speed. There are also other possible re-
search directions to improve the proposed architec-
ture. Additional scenarios are needed to improve the
evaluation such as considering more data sets and per-
formance metrics (eg. CO2 emission). We can also
include the prediction of unplanned events by using
learning methods. In this case, communication at the
C-ITS sub-system to identify such a service can be
avoided by detecting an event before it even happens.
Besides, extending our proposal by considering the
security aspect is another challenging and crucial re-
search direction.
REFERENCES
Aloui, A., Hachicha, H., and Zagrouba, E. (2021). A mobile
agent-based framework to monitor and manage inter-
operability of c-its services in vehicles. In Agents and
Multi-Agent Systems: Technologies and Applications
2021: Proceedings of 15th KES International Confer-
ence, KES-AMSTA 2021, June 2021, pages 89–101.
Springer.
Banks, J. H. (2002). Introduction to transportation engi-
neering, volume 21. McGraw-Hill New York.
Bedogni, L., Gramaglia, M., Vesco, A., Fiore, M., H
¨
arri, J.,
and Ferrero, F. (2015). The bologna ringway dataset:
Improving road network conversion in sumo and val-
idating urban mobility via navigation services. IEEE
Transactions on Vehicular Technology, 64(12):5464–
5476.
Belbachir, A., El Fallah-Seghrouchni, A., Casals, A., and
Pasin, M. (2019). Smart mobility using multi-agent
system. Procedia Computer Science, 151:447–454.
Bellifemine, F., Bergenti, F., Caire, G., and Poggi, A.
(2005). Jade—a java agent development framework.
Multi-agent programming: Languages, platforms and
applications, pages 125–147.
Dajsuren, Y., Karkhanis, P., Kadiogullary, D., and Fuen-
frocken, M. (2017). C-mobile d3. 1 reference archi-
tecture. Technical report, Technical report.
Davis, S. C. and Boundy, R. G. (2021). Transportation en-
ergy data book: Edition 39. Technical report, Oak
Ridge National Lab.(ORNL), Oak Ridge, TN (United
States).
Devangavi, A. D. and Gupta, R. (2017). Adaptive conges-
tion controlled multipath routing in vanet: A multia-
gent based approach. International Journal of Agent
Technologies and Systems (IJATS), 9(1):43–68.
Gu
´
eriau, M., Armetta, F., Hassas, S., Billot, R., and
El Faouzi, N.-E. (2016). A constructivist approach for
a self-adaptive decision-making system: application
to road traffic control. In 2016 IEEE 28th Interna-
tional Conference on Tools with Artificial Intelligence
(ICTAI), pages 670–677. IEEE.
Hamdani, M., Sahli, N., Jabeur, N., and Khezami, N.
(2022). Agent-based approach for connected vehicles
and smart road signs collaboration. Computing and
Informatics, 41(1):376–396.
Hamidi, H. and Kamankesh, A. (2018). An approach to
intelligent traffic management system using a multi-
agent system. International Journal of Intelligent
Transportation Systems Research, 16:112–124.
Li, S., Edwards, S., Isik, M. O., Zhang, Y., and Blythe,
P. T. (2022). Qualitative examination of cooperative-
intelligent transportation systems in cities to facilitate
large-scale future deployment. Sensors, 22(21):8423.
Naderi, M., Mahdaee, K., and Rahmani, P. (2023). Hi-
erarchical traffic light-aware routing via fuzzy rein-
forcement learning in software-defined vehicular net-
works. Peer-to-Peer Networking and Applications,
16(2):1174–1198.
Perez-Murueta, P., G
´
omez-Espinosa, A., Cardenas, C., and
Gonzalez-Mendoza Jr, M. (2019). Deep learning sys-
tem for vehicular re-routing and congestion avoid-
ance. Applied Sciences, 9(13):2717.
Teixeira, M., d’Orey, P. M., and Kokkinogenis, Z.
(2020). Simulating collective decision-making for
autonomous vehicles coordination enabled by vehic-
ular networks: A computational social choice per-
spective. Simulation Modelling Practice and Theory,
98:101983.
Tim
´
oteo, I. J., Ara
´
ujo, M. R., Rossetti, R. J., and Oliveira,
E. C. (2010). Trasmapi: An api oriented towards
multi-agent systems real-time interaction with multi-
ple traffic simulators. In 13th International IEEE Con-
ference on Intelligent Transportation Systems, pages
1183–1188. IEEE.
Wagner, C. (2013). Juzzy-a java based toolkit for type-2
fuzzy logic. In 2013 IEEE Symposium on Advances in
Type-2 Fuzzy Logic Systems (T2FUZZ), pages 45–52.
IEEE.
Zarari, C., Balbo, F., and Ghedira, K. (2018). Agent execu-
tion platform dedicated to c-its. Procedia Computer
Science, 126:821–830.
Zouari, M., Baklouti, N., Kammoun, M. H., Ayed, M. B.,
Alimi, A. M., and Sanchez-Medina, J. (2021). A
multi-agent system for road traffic decision making
based on hierarchical interval type-2 fuzzy knowledge
representation system. In 2021 IEEE International
Conference on Fuzzy Systems (FUZZ-IEEE), pages 1–
6. IEEE.
Multi-Agent Based Framework for Cooperative Traffic Management in C-ITS System
427