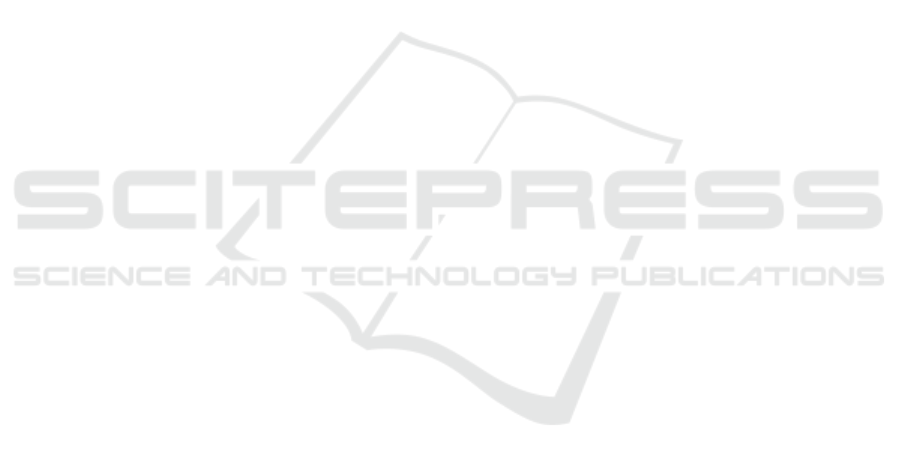
REFERENCES
Ahmed, S. M. and Mstafa, R. J. (2022). Identifying severity
grading of knee osteoarthritis from x-ray images us-
ing an efficient mixture of deep learning and machine
learning models. Diagnostics, 12(12):2939.
Ambellan, F., Tack, A., Ehlke, M., and Zachow, S. (2019).
Automated segmentation of knee bone and cartilage
combining statistical shape knowledge and convolu-
tional neural networks: Data from the osteoarthritis
initiative. Medical Image Analysis, 52:109–118.
Antony, J., McGuinness, K., Moran, K., and O’Connor,
N. E. (2017). Automatic detection of knee joints
and quantification of knee osteoarthritis severity using
convolutional neural networks. International Confer-
ence on Machine Learning and Data Mining.
Chen, P., Gao, L., Shi, X., Allen, K., and Yang, L. (2019).
Fully automatic knee osteoarthritis severity grading
using deep neural networks with a novel ordinal loss.
Computerized Medical Imaging and Graphics, 75:84–
92.
Goodfellow, I., Bengio, Y., and Courville, A. (2016). Deep
learning. MIT press.
Guida, C., Zhang, M., and Shan, J. (2021). Knee os-
teoarthritis classification using 3d cnn and mri. Ap-
plied Sciences, 11(11):5196.
Harada, Y., Osamu Tokuda, K. F., Shiraishi, G., Motomura,
T., Kimura, M., and Matsunaga, N. (2011). Relation-
ship between cartilage volume using mri and kellgren-
lawrence radiographic score in knee osteoarthritis
with and without meniscal tears. American Journal
of Roentgenology.
Haugen, I. K. and Bøyesen, P. (2011). Imaging modalities
in hand osteoarthritis-status and perspectives of con-
ventional radiography, magnetic resonance imaging,
and ultrasonography. Arthritis research & therapy,
13(6):1–8.
Hu, J., Shen, L., and Sun, G. (2018). Squeeze-and-
excitation networks. In Proceedings of the IEEE con-
ference on computer vision and pattern recognition,
pages 7132–7141.
Kellgren, J. H. and Lawrence, J. (1957). Radiological as-
sessment of osteo-arthrosis. Annals of the rheumatic
diseases, 16(4):494.
Kimura, W., Sakuma, T., and Kato, S. (2022). Segmentation
of cartilage regions in knee osteoarthritis mr images
using transunet. International Conference on Knowl-
edge Information and Creativity Support Systems.
Kinds, M. B., Marijnissen, A. C., Bijlsma, J. W., Boers, M.,
Lafeber, F. P., and Welsing, P. M. (2013). Quantita-
tive radiographic features of early knee osteoarthritis:
development over 5 years and relationship with symp-
toms in the check cohort. The Journal of rheumatol-
ogy, 40(1):58–65.
Kohn, M. D., Sassoon, A. A., and Fernando, N. D. (2016).
Classifications in brief: Kellgren-lawrence classifica-
tion of osteoarthritis. Clinical Orthopaedics and Re-
lated Research, 474:1886–1893.
Mohammed, A. S., Hasanaath, A. A., Latif, G., and Bashar,
A. (2023). Knee osteoarthritis detection and severity
classification using residual neural networks on pre-
processed x-ray images. Diagnostics, 13(8):1380.
Nevitt, M., Felson, D., and Lester, G. (2006). The os-
teoarthritis initiative. Protocol for the cohort study,
1.
Roemer, F. W., Kwoh, C. K., Hayashi, D., Felson, D. T., and
Guermazi, A. (2018). The role of radiography and mri
for eligibility assessment in dmoad trials of knee oa.
Nature Reviews Rheumatology, 14(6):372–380.
Russakovsky, O., Deng, J., Su, H., Krause, J., Satheesh, S.,
Ma, S., Huang, Z., Karpathy, A., Khosla, A., Bern-
stein, M., Berg, A. C., and Fei-Fei, L. (2015). Ima-
geNet Large Scale Visual Recognition Challenge. In-
ternational Journal of Computer Vision, 115(3):211–
252.
Schiratti, J.-B., Dubois, R., Herent, P., Cahan
´
e, D., Dachary,
J., Clozel, T., Wainrib, G., Keime-Guibert, F., La-
lande, A., Pueyo, M., Guillier, R., Gabarroca, C., and
Moingeon, P. (2021). A deep learning method for pre-
dicting knee osteoarthritis radiographic progression
from mri. Arthritis Research & Therapy, 23(1):262.
Shamir, L., Ling, S. M., Scott, W. W., Bos, A., Orlov, N.,
Macura, T. J., Eckley, D. M., Ferrucci, L., and Gold-
berg, I. G. (2008). Knee x-ray image analysis method
for automated detection of osteoarthritis. IEEE Trans-
actions on Biomedical Engineering, 56(2):407–415.
Tack, A., Ambellan, F., and Zachow, S. (2021). Towards
novel osteoarthritis biomarkers: Multi-criteria evalua-
tion of 46,996 segmented knee mri data from the os-
teoarthritis initiative. PloS one, 16(10):e0258855.
Taha, A. A. and Hanbury, A. (2015). Metrics for evaluating
3d medical image segmentation: analysis, selection,
and tool. BMC medical imaging, 15(1):1–28.
Thomas, K. A., Łukasz Kidzi
´
nski, Halilaj, E., Fleming,
S. L., Venkataraman, G. R., Oei, E. H. G., Gold, G. E.,
and Delp, S. L. (2020). Automated classification of
radiographic knee osteoarthritis severity using deep
neural networks. Radiology: Artificial Intelligence,
2(2):e190065.
Tiulpin, A., Thevenot, J., Rahtu, E., Lehenkari, P., and
Saarakkala, S. (2018). Automatic knee osteoarthri-
tis diagnosis from plain radiographs: a deep learning-
based approach. Scientific reports, 8(1):1727.
Vaishya, R., Pariyo, G. B., Agarwal, A. K., and Vijay, V.
(2016). Non-operative management of osteoarthritis
of the knee joint. Journal of clinical orthopaedics and
trauma, 7(3):170–176.
Wang, C., Deng, C., and Wang, S. (2020). Imbalance-
xgboost: leveraging weighted and focal losses for
binary label-imbalanced classification with xgboost.
Pattern Recognition Letters, 136:190–197.
Prediction of Kellgren-Lawrence Grade of Knee Osteoarthritis by Deep Residual Networks Using MR Image with Segmented Image and
Slice Position
1307