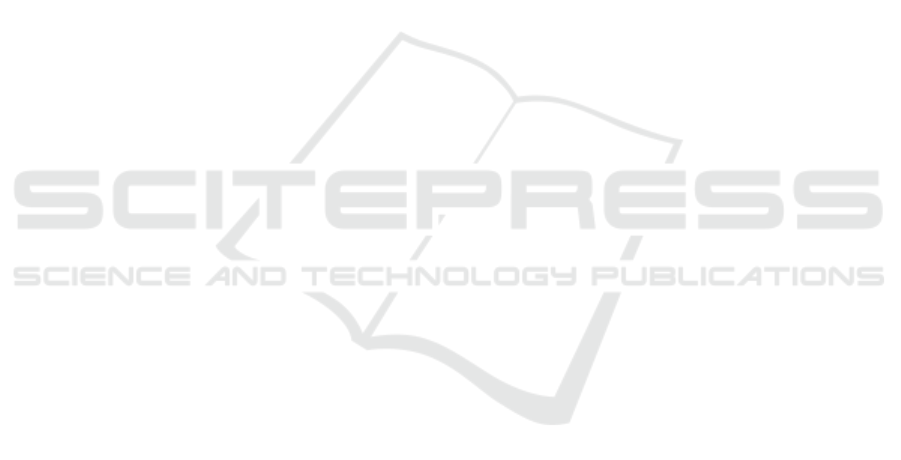
Meske, C., Bunde, E., Schneider, J., and Gersch, M. (2022).
Explainable Artificial Intelligence: Objectives, Stake-
holders, and Future Research Opportunities. Informa-
tion Systems Management, 39(1):53–63.
Miller, T. (2019). Explanation in artificial intelligence: In-
sights from the social sciences. Artificial Intelligence,
267:1–38.
Nocker, M., Drexel, D., Rader, M., Montuoro, A., and
Sch
¨
ottle, P. (2023). HE-MAN – Homomorphically
Encrypted MAchine Learning with ONnx Models. In
Proceedings of the 8th International Conference on
Machine Learning Technologies, ICMLT ’23, page
35–45, Stockholm, Sweden. ACM.
Nunes, P., Santos, J. P., and Rocha, E. M. (2023). Chal-
lenges in predictive maintenance – A review. CIRP
Journal of Manufacturing Science and Technology,
40:53–67.
Ogrezeanu, I., Vizitiu, A., Cius
,
del, C., Puiu, A., Coman,
S., Boldis
,
or, C., Itu, A., Demeter, R., Moldoveanu,
F., Suciu, C., and Itu, L. (2022). Privacy-Preserving
and Explainable AI in Industrial Applications. Ap-
plied Sciences, 12(13):6395.
Papenmeier, A., Kern, D., Englebienne, G., and Seifert, C.
(2022). It’s Complicated: The Relationship between
User Trust, Model Accuracy and Explanations in AI.
ACM Transactions on Computer-Human Interaction,
29(4):35.
Phong, L. T., Aono, Y., Hayashi, T., Wang, L., and Moriai,
S. (2017). Privacy-preserving deep learning via addi-
tively homomorphic encryption. IEEE Transactions
on Information Forensics and Security, 13(5):1333–
1345.
Preece, A. (2018). Asking ‘Why’ in AI: Explainability of
intelligent systems - perspectives and challenges. In-
telligent Systems in Accounting, Finance and Manage-
ment, 25(2):63–72.
Saeed, W. and Omlin, C. (2023). Explainable AI (XAI):
A systematic meta-survey of current challenges and
future opportunities. Knowledge-Based Systems,
263:110273.
Saghiri, A. M., Vahidipour, S. M., Jabbarpour, M. R.,
Sookhak, M., and Forestiero, A. (2022). A Survey
of Artificial Intelligence Challenges: Analyzing the
Definitions, Relationships, and Evolutions. Applied
Sciences, 12(8):4054.
Samek, W., Montavon, G., Vedaldi, A., Hansen, L. K., and
M
¨
uller, K.-R. (2019). Explainable AI: Interpreting,
Explaining and Visualizing Deep Learning. Springer.
Sapankevych, N. I. and Sankar, R. (2009). Time Series Pre-
diction Using Support Vector Machines: A Survey.
IEEE Computational Intelligence Magazine, 4(2):24–
38.
Schonlau, M. and Zou, R. Y. (2020). The Random Forest
Algorithm for Statistical Learning. The Stata Journal,
20(1):3–29.
Sheikh, H., Prins, C., and Schrijvers, E. (2023). Mission AI
- The New System Technology. Springer Cham.
Song, Y.-Y. and Lu, Y. (2015). Decision tree methods: ap-
plications for classification and prediction. Shanghai
Archives of Psychiatry, 27(2):130–135.
Strobelt, H., Gehrmann, S., Pfister, H., and Rush, A. M.
(2018). LSTMVis: A Tool for Visual Analysis of Hid-
den State Dynamics in Recurrent Neural Networks.
IEEE Trans. on Visualization and Computer Graph-
ics, 24(1):667–676.
Sukhodolskiy, I. and Zapechnikov, S. (2020). Analysis
of Secure Protocols and Authentication Methods for
Messaging. Procedia Computer Science, 169:407–
411.
Timan, T., Mann, Z., Curry, E., Metzger, A., Zillner, S.,
Pazzaglia, J.-C., and Robles, A. G. (2021). Data Pro-
tection in the Era of Artificial Intelligence: Trends,
Existing Solutions and Recommendations for Privacy-
Preserving Technologies, pages 153–175. Springer Int
Publishing.
Wang, J., Wang, L., Zheng, Y., Yeh, C.-C. M., Jain, S., and
Zhang, W. (2023). Learning-From-Disagreement: A
Model Comparison and Visual Analytics Framework.
IEEE Trans. on Visualization and Computer Graphics,
29(9):3809–3825.
Wang, Y. and Tuerhong, G. (2022). A Survey of Inter-
pretable Machine Learning Methods. In Proceed-
ings of the International Conference on Virtual Re-
ality, Human-Computer Interaction and Artificial In-
telligence, VRHCIAI ’22, pages 232–237, Changsha,
China.
Wang, Z. and Srinivasan, R. S. (2017). A review of artifi-
cial intelligence based building energy use prediction:
Contrasting the capabilities of single and ensemble
prediction models. Renewable and Sustainable En-
ergy Reviews, 75:796–808.
Ward, M., Grinstein, G. G., and Keim, D. (2015). Interac-
tive data visualization: Foundations, Techniques, and
Applications. AK Peters.
Xenopoulos, P., Rulff, J., Nonato, L. G., Barr, B., and Silva,
C. (2023). Calibrate: Interactive Analysis of Proba-
bilistic Model Output. IEEE Trans. on Visualization
and Computer Graphics, 29(1):853–863.
Xu, F., Uszkoreit, H., Du, Y., Fan, W., Zhao, D., and Zhu,
J. (2019). Explainable AI: A Brief Survey on His-
tory, Research Areas, Approaches and Challenges. In
Proceedings of the CCF International Conference on
Natural Language Processing and Chinese Comput-
ing, NLPCC ’19, pages 563–574, Dunhuang, China.
Springer Int Publishing.
Yang, W., Wei, Y., Wei, H., Chen, Y., Huang, G., Li, X.,
Li, R., Yao, N., Wang, X., Gu, X., Amin, M. B., and
Kang, B. (2023). Survey on Explainable AI: From
Approaches, Limitations and Applications Aspects.
Human-Centric Intelligent Systems, 3:161–188.
Yi, X., Paulet, R., and Bertino, E. (2014). Homomorphic
Encryption, pages 27–46. Springer Int Publishing.
Zhang, C. and Lu, Y. (2021). Study on artificial intelligence:
The state of the art and future prospects. Journal of
Industrial Information Integration, 23:100224.
Zhang, C., Xie, Y., Bai, H., Yu, B., Li, W., and Gao, Y.
(2021). A Survey on Federated Learning. Knowledge-
Based Systems, 216:106775.
Navigating the Trade-Off Between Explainability and Privacy
733