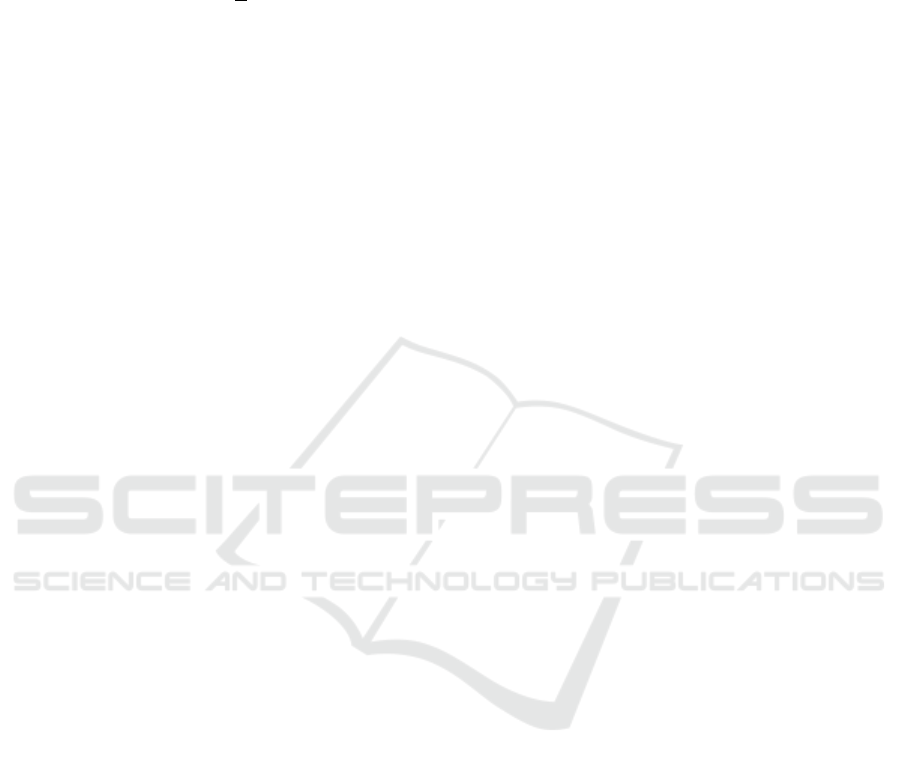
gories, risk score categories) : S1 {(0),(1-10)} ; S2
{(0),(6-10)} ; S3 {(0-2),(3-10)} and S4 {(0-5),(6-
10)}. In view of the performances, the S2 strategy
is the most suitable for risk discrimination between
the different modalities. By excluding low scores, it’s
easier to separate the R and R classes. Small scores
are often more open to human interpretation, and tend
to vary between different users, particularly with re-
gard to user-based risk.
5 CONCLUSIONS
We propose the first framework on risk analysis
for autonomous railway driving, named SMART-RD.
Based on the RailSem19 dataset, SMART-RD pro-
vides additional annotations on the general risk based
on weather, light, users and context. A first study is
performed on these data according to a binary dan-
ger or non-danger classification. The proposed base-
line is based on traditional learning models in image
processing and does not take into account recent ad-
vances in the literature for risk analysis. In future
work, we explore more complex decision models bet-
ter adapted to risk assessment, taking into account the
per-modality annotation to assess the general risk.
REFERENCES
Cicchetti, D. (1994). Guidelines, criteria, and rules of
thumb for evaluating normed and standardized assess-
ment instrument in psychology. Psychological Assess-
ment, 6:284–290.
Corcoran, G.-P. and Clark, J. (2019). Traffic Risk As-
sessment: A Two-Stream Approach Using Dynamic-
Attention. In 2019 16th Conference on Computer and
Robot Vision (CRV), pages 166–173, Kingston, QC,
Canada. IEEE.
Deng, J., Dong, W., Socher, R., Li, L.-J., Li, K., and Fei-
Fei, L. (2009). Imagenet: A large-scale hierarchical
image database. In 2009 IEEE Conference on Com-
puter Vision and Pattern Recognition, pages 248–255.
Dosovitskiy, A., Beyer, L., Kolesnikov, A., Weissenborn,
D., Zhai, X., Unterthiner, T., Dehghani, M., Minderer,
M., Heigold, G., Gelly, S., Uszkoreit, J., and Houlsby,
N. (2021). An image is worth 16x16 words: Trans-
formers for image recognition at scale.
Feth, P., Akram, M. N., Schuster, R., and Wasenm
¨
uller,
O. (2018). Dynamic Risk Assessment for Vehicles
of Higher Automation Levels by Deep Learning. In
Hoshi, M. and Seki, S., editors, Developments in
Language Theory, volume 11088, pages 535–547.
Springer International Publishing, Cham. Series Ti-
tle: Lecture Notes in Computer Science.
Grigorescu, S., Trasnea, B., Cocias, T., and Macesanu,
G. (2020). A survey of deep learning techniques
for autonomous driving. Journal of Field Robotics,
37(3):362–386.
Guo, J., Junyao Guo, Kurup, U., Shah, M., and Shah, M.
(2020). Is it Safe to Drive? An Overview of Factors,
Metrics, and Datasets for Driveability Assessment in
Autonomous Driving. IEEE Transactions on Intelli-
gent Transportation Systems, 21(8):3135–3151.
Janai, J., G
¨
uney, F., Behl, A., and Geiger, A. (2020).
Computer vision for autonomous vehicles: Problems,
datasets and state of the art. Foundations and Trends®
in Computer Graphics and Vision, 12(1–3):1–308.
Kuutti, S., Bowden, R., Jin, Y., Barber, P., and Fallah,
S. (2021). A Survey of Deep Learning Applications
to Autonomous Vehicle Control. IEEE Transactions
on Intelligent Transportation Systems, 22(2):712–733.
Conference Name: IEEE Transactions on Intelligent
Transportation Systems.
Loshchilov, I. and Hutter, F. (2019). Decoupled weight
decay regularization. In International Conference on
Learning Representations.
Nunnally, J. C. (1978). Psychometric theory / Jum C. Nun-
nally. McGraw-Hill New York, 2d ed. edition.
Pappaterra, M. J., Flammini, F., Vittorini, V., and Be
ˇ
sinovi
´
c,
N. (2021). A Systematic Review of Artificial Intelli-
gence Public Datasets for Railway Applications. In-
frastructures, 6(10):136. Number: 10 Publisher: Mul-
tidisciplinary Digital Publishing Institute.
Van Brummelen, J., O’Brien, M., Gruyer, D., and Najjaran,
H. (2018). Autonomous vehicle perception: The tech-
nology of today and tomorrow. Transportation Re-
search Part C: Emerging Technologies, 89:384–406.
Vargas, J., Alsweiss, S., Toker, O., Razdan, R., and Santos,
J. (2021). An Overview of Autonomous Vehicles Sen-
sors and Their Vulnerability to Weather Conditions.
Sensors, 21(16):5397.
Wang, Y. and Kato, J. (2017). Collision Risk Rating of
Traffic Scene from Dashboard Cameras. In 2017 In-
ternational Conference on Digital Image Computing:
Techniques and Applications (DICTA), pages 1–6.
Yurtsever, E., Lambert, J., Carballo, A., and Takeda, K.
(2020). A Survey of Autonomous Driving: Common
Practices and Emerging Technologies. IEEE Access,
8:58443–58469.
Yurtsever, E., Liu, Y., Lambert, J., Miyajima, C., Takeuchi,
E., Takeda, K., and Hansen, J. H. L. (2019). Risky
Action Recognition in Lane Change Video Clips us-
ing Deep Spatiotemporal Networks with Segmenta-
tion Mask Transfer. In 2019 IEEE Intelligent Trans-
portation Systems Conference (ITSC), pages 3100–
3107.
Zendel, O., Murschitz, M., Zeilinger, M., Steininger, D.,
Abbasi, S., and Beleznai, C. (2019). RailSem19:
A Dataset for Semantic Rail Scene Understanding.
In 2019 IEEE/CVF Conference on Computer Vision
and Pattern Recognition Workshops (CVPRW), pages
1221–1229. ISSN: 2160-7516.
Zhang, Y., Carballo, A., Yang, H., and Takeda, K. (2023).
Perception and sensing for autonomous vehicles under
adverse weather conditions: A survey. ISPRS Journal
of Photogrammetry and Remote Sensing, 196:146–
177.
SMART-RD: Towards a Risk Assessment Framework for Autonomous Railway Driving
811