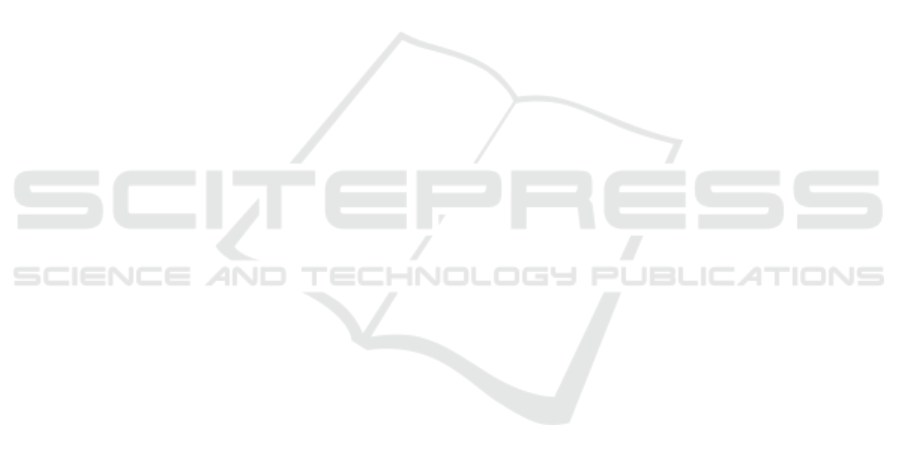
010 (2020/02/Y/ST6/00064), and by the CNRS IEA
project MoSART. For the purpose of open access,
and in fulfilment of the obligations arising from the
grant agreement, the authors have applied a Creative
Commons Attribution 4.0 International (CC BY 4.0)
license to any Author Accepted Manuscript version
arising from this submission.
REFERENCES
Arapinis, M., Cortier, V., and Kremer, S. (2016). When are
three voters enough for privacy properties? In Pro-
ceedings of ESORICS, volume 9879 of Lecture Notes
in Computer Science, pages 241–260. Springer.
Behrmann, G., David, A., and Larsen, K. (2004). A tuto-
rial on UPPAAL. In Formal Methods for the Design of
Real-Time Systems: SFM-RT, number 3185 in LNCS,
pages 200–236. Springer.
Bulling, N., Goranko, V., and Jamroga, W. (2015). Logics
for reasoning about strategic abilities in multi-player
games. In Models of Strategic Reasoning. Logics,
Games, and Communities, volume 8972 of Lecture
Notes in Computer Science, pages 93–136. Springer.
Clarke, E., Henzinger, T., Veith, H., and Bloem, R., editors
(2018). Handbook of Model Checking. Springer.
Conti, M. and Passarella, A. (2018). The internet of peo-
ple: A human and data-centric paradigm for the next
generation internet. Comput. Commun., 131:51–65.
Contucci, P., Kertesz, J., and Osabutey, G. (2022). Human-
ai ecosystem with abrupt changes as a function of the
composition. PLOS ONE, 17(5):1–12.
Dolev, D. and Yao, A. C. (1983). On the security of public
key protocols. IEEE Trans. Inf. Theory, 29(2):198–
207.
Drainakis, G., Katsaros, K. V., Pantazopoulos, P., Sourlas,
V., and Amditis, A. (2020). Federated vs. centralized
machine learning under privacy-elastic users: A com-
parative analysis. In Proceedings of NCA, pages 1–8.
IEEE.
Emerson, E. (1990). Temporal and modal logic. In van
Leeuwen, J., editor, Handbook of Theoretical Com-
puter Science, volume B, pages 995–1072. Elsevier.
Fuchs, A., Passarella, A., and Conti, M. (2022). Model-
ing human behavior part I - learning and belief ap-
proaches. CoRR, abs/2205.06485.
Gollmann, D. (2011). Computer Security (3. ed.). Wiley.
Goodfellow, I. J., McDaniel, P. D., and Papernot, N. (2018).
Making machine learning robust against adversarial
inputs. Commun. ACM, 61(7):56–66.
Heged
¨
us, I., Danner, G., and Jelasity, M. (2019). Gos-
sip learning as a decentralized alternative to federated
learning. In Proceedings of IFIP DAIS, volume 11534
of Lecture Notes in Computer Science, pages 74–90.
Springer.
Heged
¨
us, I., Danner, G., and Jelasity, M. (2021). Decen-
tralized learning works: An empirical comparison of
gossip learning and federated learning. J. Parallel Dis-
tributed Comput., 148:109–124.
Jamroga, W. and Kim, Y. (2023a). Practical abstraction for
model checking of multi-agent systems. In Proceed-
ings of KR, pages 384–394.
Jamroga, W. and Kim, Y. (2023b). Practical model re-
ductions for verification of multi-agent systems. In
Proceedings of the Thirty-Second International Joint
Conference on Artificial Intelligence, IJCAI, pages
7135–7139. ijcai.org.
Kianpour, M. and Wen, S. (2019). Timing attacks on ma-
chine learning: State of the art. In IntelliSys Volume 1,
volume 1037 of Advances in Intelligent Systems and
Computing, pages 111–125. Springer.
Kumar, R. S. S., Nystr
¨
om, M., Lambert, J., Marshall, A.,
Goertzel, M., Comissoneru, A., Swann, M., and Xia,
S. (2020). Adversarial machine learning-industry per-
spectives. In IEEE Security and Privacy Workshops,
pages 69–75. IEEE.
Kurpiewski, D., Jamroga, W., and Sidoruk, T. (2023). To-
wards modelling and verification of social explainable
AI. In Proceedings of ICAART, pages 396–403.
Kurpiewski, D., Pazderski, W., Jamroga, W., and Kim, Y.
(2021). STV+Reductions: Towards practical verifi-
cation of strategic ability using model reductions. In
Proceedings of AAMAS, pages 1770–1772. ACM.
Lorenzo, V., Boldrini, C., and Passarella, A. (2022). SAI
simulator for social AI gossiping. https://zenodo.org/
record/5780042.
Ottun, A.-R., Mane, P. C., Yin, Z., Paul, S., Liyanage,
M., Pridmore, J., Ding, A. Y., Sharma, R., Nurmi,
P., and Flores, H. (2022). Social-aware federated
learning: Challenges and opportunities in collabora-
tive data training. IEEE Internet Computing, pages
1–7.
Palmieri, L., Boldrini, C., Valerio, L., Passarella, A., and
Conti, M. (2023a). Exploring the impact of disrupted
peer-to-peer communications on fully decentralized
learning in disaster scenarios. In International Con-
ference on Information and Communication Technolo-
gies for Disaster Management, ICT-DM, pages 1–6.
IEEE.
Palmieri, L., Valerio, L., Boldrini, C., and Passarella, A.
(2023b). The effect of network topologies on fully
decentralized learning: a preliminary investigation.
CoRR, abs/2307.15947.
Priese, L. (1983). Automata and concurrency. Theoretical
Computer Science, 25:221–265.
Shoham, Y. and Leyton-Brown, K. (2009). Multiagent
Systems - Algorithmic, Game-Theoretic, and Logical
Foundations. Cambridge University Press.
Social AI gossiping. Micro-project in Humane-AI-Net
(2022). Project website. https://www.ai4europe.eu/
research/research-bundles/social-ai-gossiping.
Social Explainable AI, CHIST-ERA (2021–24). Project
website. http://www.sai-project.eu/.
Toprak, M., Boldrini, C., Passarella, A., and Conti, M.
(2021). Harnessing the power of ego network layers
for link prediction in online social networks. CoRR,
abs/2109.09190.
Weiss, G., editor (1999). Multiagent Systems. A Modern
Approach to Distributed Artificial Intelligence. MIT
Press: Cambridge, Mass.
ICAART 2024 - 16th International Conference on Agents and Artificial Intelligence
158