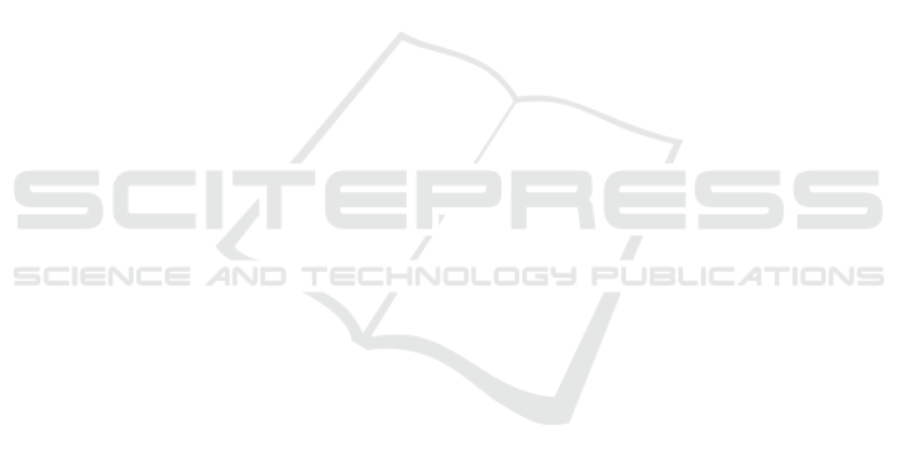
Ericsson, K. A. and Lehmann, A. C. (1996). Expert and ex-
ceptional performance: Evidence of maximal adapta-
tion to task constraints. Annual review of psychology.
Fleiss, J. L. (1971). Measuring nominal scale agreement
among many raters. Psychological bulletin.
Gaševi
´
c, D., Dawson, S., Rogers, T., and Gasevic, D.
(2016). Learning analytics should not promote one
size fits all: The effects of instructional conditions in
predicting academic success. IHE, 28:68–84.
Greller, W. and Drachsler, H. (2012). Translating learning
into numbers: A generic framework for learning ana-
lytics. ET&S, 15(3):42–57.
Gruber, M. (2019). Designing for great teaching with
learning design cards. UZH philosophy facultydigital
teaching and research.
Harrington, K., , et al. (2014). Engagement through part-
nership: Students as partners in learning and teaching
in higher education.
Hoffman, R. R. (1987). The problem of extracting the
knowledge of experts from the perspective of exper-
imental psychology. AI magazine, 8(2):53–53.
Ko, A., Pick, C. M., Kwon, J. Y., Barlev, M., Krems, et al.
(2020). Family matters: Rethinking the psychology of
human social motivation. PPS, 15(1):173–201.
Landis, J. R. and Koch, G. G. (1977). The measurement of
observer agreement for categorical data. biometrics,
pages 159–174.
Laurillard, D. (2013). Teaching as a design science: Build-
ing pedagogical patterns for learning and technology.
Leclercq, D. and Poumay, M. (2005). The 8 learning events
model and its principles (release 2005-1).
Lockyer, L. and Dawson, S. (2011). Learning designs and
learning analytics. In LAK ’11, pages 153–156.
Mangaroska, K. and Giannakos, M. (2018). Learning an-
alytics for learning design: A systematic literature
review of analytics-driven design to enhance learn-
ing. IEEE Transactions on Learning Technologies,
12(4):516–534.
Martin, F., Ndoye, A., and Wilkins, P. (2016). Using learn-
ing analytics to enhance student learning in online
courses based on quality matters standards. Journal
of Educational Technology Systems, 45(2):165–187.
Morrison, D. (2006). Critical thinking in a collaborative
online learning environment. Advanced Technology
for Learning, 3(4):255–262.
Nguyen, Q., Rienties, B., and Whitelock, D. (2022). In-
forming learning design in online education using
learning analytics of student engagement. Open World
Learn, 1:189–207.
Peer, E., Vosgerau, J., and Acquisti, A. (2014). Reputa-
tion as a sufficient condition for data quality on ama-
zon mechanical turk. Behavior research methods,
46:1023–1031.
Raes, A., Detienne, L., Windey, I., and Depaepe, F. (2020).
A systematic literature review on synchronous hybrid
learning: gaps identified. Learning Environments Re-
search, 23:269–290.
Ruis, A., Siebert-Evenstone, A., Pozen, R., Eagan, B., and
Shaffer, D. W. (2019). Finding common ground: a
method for measuring recent temporal context in anal-
yses of complex, collaborative thinking.
Ryan, R. M. and Deci, E. L. (2022). Self-determination
theory. In Encyclopedia of quality of life and well-
being research, pages 1–7. Springer.
Schmitz, M., Scheffel, M., Bemelmans, R., and Drachsler,
H. (2022). Fola 2–a method for co-creating learn-
ing analytics-supported learning design. Journal of
Learning Analytics, 9(2):265–281.
Schmitz, M., Scheffel, M., Bemelmans, R., and Drachsler,
H. (2023). Evaluating the impact of fola2 on learning
analytics knowledge creation and acceptance during
the co-design of learning activities. Interaction De-
sign and Architecture (s), 55:9–33.
Schmitz, M., Van Limbeek, E., Greller, W., Sloep, P., and
Drachsler, H. (2017). Opportunities and challenges
in using learning analytics in learning design. In EC-
TEL 2017, Tallinn, Estonia, September 12–15, 2017,
Proceedings 12, pages 209–223. Springer.
Shaffer, D. and Ruis, A. (2017). Epistemic network analy-
sis: A worked example of theory-based learning ana-
lytics. Handbook of LA.
Shulman, L. (1987). Knowledge and teaching: Founda-
tions of the new reform. Harvard educational review,
57(1):1–23.
Siebert-Evenstone, A. L., Irgens, G. A., Collier, W.,
Swiecki, Z., Ruis, A. R., and Shaffer, D. W. (2017). In
search of conversational grain size: Modeling seman-
tic structure using moving stanza windows. Journal of
Learning Analytics, 4(3):123–139.
Siemens, G. and Long, P. (2011). Penetrating the fog: An-
alytics in learning and education.
Sitzmann, T. (2011). A meta-analytic examination of the
instructional effectiveness of computer-based simula-
tion games. Personnel psychology, 64(2):489–528.
Stasser, G., Stewart, D. D., and Wittenbaum, G. M. (1995).
Expert roles and information exchange during dis-
cussion: The importance of knowing who knows
what. Journal of experimental social psychology,
31(3):244–265.
Suskie, L. (2018). Assessing student learning: A common
sense guide. John Wiley & Sons.
Verpoorten, D., Poumay, M., and Leclercq, D. (2007). The
eight learning events model: A pedagogic conceptual
tool supporting diversification of learning methods.
Interactive Learning Environments, 15(2):151–160.
Vezzoli, Y., Mavrikis, M., and Vasalou, A. (2020). Inspi-
ration cards workshops with primary teachers in the
early co-design stages of learning analytics. In LAK
’10, pages 73–82.
Weitze, C. L., Ørngreen, R., and Levinsen, K. (2013). The
global classroom video conferencing model and first
evaluations. In ECEL ’12, pages 503–510. Academic
Conferences and Publishing International.
Wiggins, G. P. and McTighe, J. (2005). Understanding by
design. Ascd.
Zhu, M., Bonk, C. J., and Sari, A. R. (2018). Instructor ex-
periences designing moocs in higher education: Ped-
agogical, resource, and logistical considerations and
challenges. Online Learning, 22(4):203–241.
CSEDU 2024 - 16th International Conference on Computer Supported Education
26