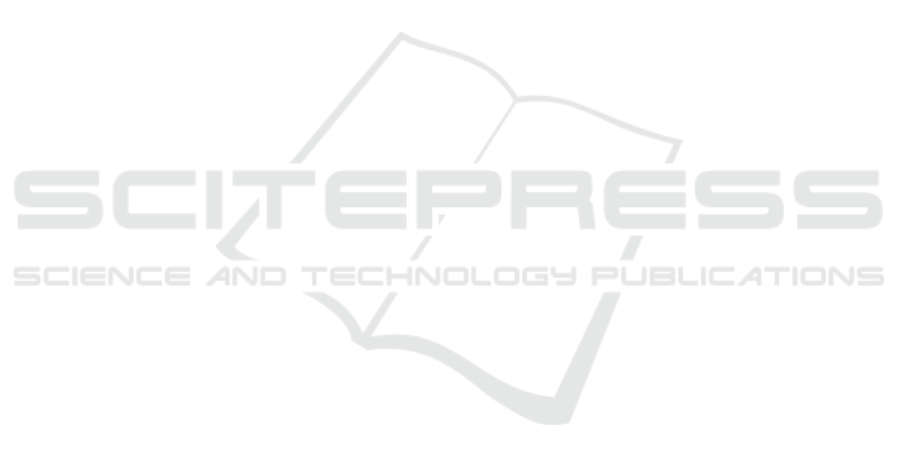
or smaller university courses could not be answered,
but difficulties were identified that should be consid-
ered in the future: On the one hand, that contact is
often not established when the task of making contact
is left to the students themselves. On the other hand,
that even if contact is made, it does not also mean that
meetings of the study group will take place. This is
probably due to scheduling difficulties.
The paper thus provides initial indications of what
may be important to students when learning in study
groups and what needs to be considered when im-
plementing a tool for the formation of self-organised
study groups. Future studies should, for example, ex-
amine which goals students pursue with learning in
study groups. The present work indicates that there
are apparently different goals that motivate students
to learn in study groups, and that common goals may
be important for group formation. In addition, future
research would be needed to examine how the students
perceive learning in the groups formed by the genetic
algorithm presented in this paper. With regard to the
implementation of a tool for the formation of study
groups, the question of how to facilitate contact re-
mains to be answered. Future studies could explore
this question further and, for example, try to get in-
formation through surveys about what could motivate
students to contact others and what tends to prevent
them from doing so. In addition, it could be help-
ful to consider characteristics such as the number of
semesters or the subject of study when forming study
groups in order to make it easier for the students to
schedule meetings.
All in all, an application for finding study groups
seems to be considered useful by the students – the fact
that the prototype presented in this paper was used by
287 students overall indicates that there is a need for
such a tool in higher education. Nevertheless, further
research is needed to fully ensure the expectations of
students – especially regarding their different goals –
are met and to facilitate the contact establishment and
scheduling of meetings.
The source code of the registration form (incl. the
questionnaire) as well as the grouping tool (Schenk and
Strickroth, 2024a), and the evaluation questionnaire as
well as the raw data (Schenk and Strickroth, 2024b)
are available on Zenodo.
ACKNOWLEDGEMENTS
The authors thank the two educators Ruediger Wester-
mann and Armin Egetenmeier at Technical University
of Munich respective Aalen University of Applied Sci-
ences and also all participants.
REFERENCES
Benighaus, C. and Benighaus, L. (2012). Moderation,
Gespr
¨
achsaufbau und Dynamik in Fokusgruppen. In
Fokusgruppen in der empirischen Sozialwissenschaft:
Von der Konzeption bis zur Auswertung, pages 111–
132. Springer.
Brodbeck, F. C., Anderson, N., and West, M. (2000). Das
Teamklima-Inventar. Hogrefe.
Cook-Sather, A. (2002). Authorizing students’ perspectives:
Toward trust, dialogue, and change in education. Edu-
cational researcher, 31(4):3–14.
Costa, P. T. and McCrae, R. R. (1989). The NEO PI/FFI
Manual Supplement. Psychological Assessment Re-
sources.
Cruz, W. M. and Isotani, S. (2014). Group formation algo-
rithms in collaborative learning contexts: A systematic
mapping of the literature. In Proc. CRIWG, pages
199–214. Springer.
Davidson, N., Major, C. H., and Michaelsen, L. K.
(2014). Small-group learning in higher educa-
tion—cooperative, collaborative, problem-based, and
team-based learning: an introduction by the guest edi-
tors. JECT, 25(3-4):1–6.
Dillenbourg, P. (1999). What do you mean by collabora-
tive learning? Collaborative-learning: Cognitive and
Computational Approaches, 1:1–19.
Dillenbourg, P. and Fischer, F. (2007). Computer-supported
collaborative learning: The basics. Zeitschrift f
¨
ur
Berufs- und Wirtschaftsp
¨
adagogik, 21:111–130.
Ennen, N. L., Stark, E., and Lassiter, A. (2015). The impor-
tance of trust for satisfaction, motivation, and academic
performance in student learning groups. Social Psy-
chology of education, 18:615–633.
Fleckenstein, J., Schmidt, F. T., and M
¨
oller, J. (2014). Wer
hat biss? Beharrlichkeit und best
¨
andiges interesse von
lehramtsstudierenden; Eine deutsche adaptation der
12-ltem Grit Scale. Psychologie in Erziehung und
Unterricht, 61(4):281–286.
Freeman, S., Theobald, R., Crowe, A. J., and Wenderoth,
M. P. (2017). Likes attract: Students self-sort in a class-
room by gender, demography, and academic character-
istics. Active Learning in Higher Education, 18(2):115–
126.
Gibbs, A. (2012). Focus groups and group interviews.
Research methods and methodologies in education,
186:192.
Gillies, R. M. (2016). Cooperative learning: Review of
research and practice. Australian Journal of Teacher
Education (Online), 41(3):39–54.
Jacobs, G. M. (2015). Collaborative learning or coopera-
tive learning? the name is not important; flexibility is.
Online Submission, 3(1):32–52.
Kauffeld, S. and Frieling, E. (2004). Fragebogen zur Arbeit
im Team: FAT. Hogrefe.
Konert, J., Burlak, D., and Steinmetz, R. (2014).
GroupAL: ein Algorithmus zur Formation und
Qualit
¨
atsbewertung von Lerngruppen in E-Learning-
Szenarien. i-com, 13(1):70–81.
Formation of Study Groups: Exploring Students’ Needs and Practical Challenges
657