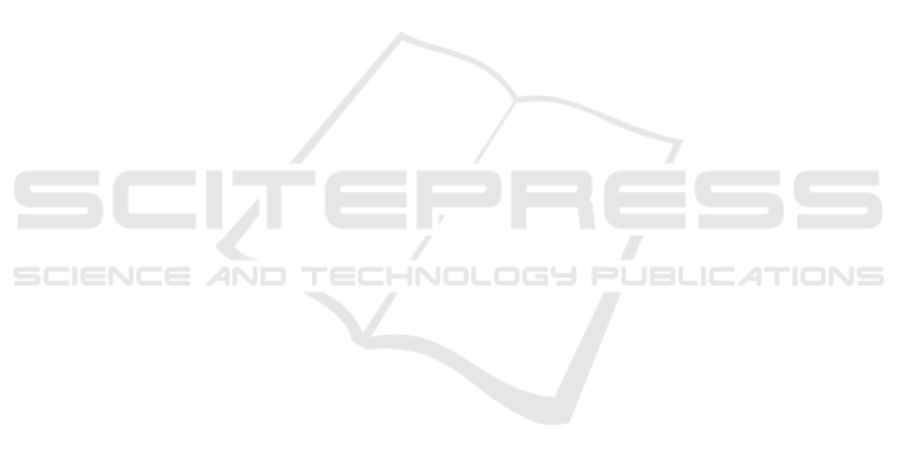
8 CONCLUSION
This paper proposes a Bayesian Network that uses ac-
cident data analysis to label and predict congestion
states. There are various approaches to define conges-
tion from accident datasets. In this work, a novel tech-
nique for labeling congestions uses formula-based
and hotspot-based approaches. Furthermore, to ob-
serve the model performance, the congestion states
were classified into 3-class states (low, medium, and
high) and 2-class states (low, high). The results
show that the proposed model performance is higher
in 2-class predictions, especially with the formula-
based approach of 89.1% accuracy compared to the
hotspot approach. This is the novelty of our ap-
proach. This performance is compared with differ-
ent machine learning models (Random Forest, Deci-
sion Tree, SVM, Logistic Regression), which show
that the proposed model has slightly better accuracy
and precision. It also demonstrated comparable per-
formance with ML models.
The main limitation of this work is that we re-
strict our focus to accident information. Even though
it provides valuable insights, it does not consider all
the other factors causing congestion. Moreover, we
acknowledge the need for further refinement on a
hotspot-based approach to improve its performance,
and a dedicated Bayesian model needs to be imple-
mented. Further, we will build a Dynamic Bayesian
Network focusing on the hotspot approach to label
the congestion and follow its development trends. We
will also use various factors near the hotspot, like the
speed of other surrounding vehicles, junction type,
and other points of interest (Schools, Hospitals, etc.).
Besides accidents, future work will also focus on
the root causes of non-recurring congestion due to
unforeseen events, like construction works, weather-
related, and special events. Social media blogs and
platforms can provide further insights into accident
modeling. Moreover, it is also significant to under-
stand the correlation between road safety measures,
congestion, and their joint impact on urban mobility.
REFERENCES
Afrin, T. and Yodo, N. (2021). A probabilistic estimation of
traffic congestion using bayesian network. Measure-
ment, 174:109051.
Cambridgeshire County Council (2018). Cam-
bridgeshire road traffic collision data. https:
//data.cambridgeshireinsight.org.uk/dataset/
cambridgeshire-road-traffic-collision-data. Ac-
cessed: October 10, 2023.
Chang, H., Li, L., Huang, J., Zhang, Q., and Chin, K.-S.
(2022). Tracking traffic congestion and accidents us-
ing social media data: A case study of shanghai. Ac-
cident Analysis & Prevention, 169:106618.
Dias, C., Miska, M., Kuwahara, M., and Warita, H. (2009).
Relationship between congestion and traffic accidents
on expressways: an investigation with bayesian belief
networks. In Proceedings of 40th Annual Meeting of
Infrastructure Planning (JSCE), Japan.
Gupta, U., Varun, M., and Srinivasa, G. (2022). A compre-
hensive study of road traffic accidents: Hotspot anal-
ysis and severity prediction using machine learning.
In 2022 IEEE Bombay Section Signature Conference
(IBSSC), pages 1–6. IEEE.
Ji, X., Yue, W., Li, C., Chen, Y., Xue, N., and Sha, Z.
(2022). Digital twin empowered model free predic-
tion of accident-induced congestion in urban road net-
works. In 2022 IEEE 95th Vehicular Technology
Conference:(VTC2022-Spring), pages 1–6. IEEE.
Kjaerulff, U. B. and Madsen, A. L. (2008). Bayesian net-
works and influence diagrams. Springer Science+
Business Media, 200:114.
Ma, X., Ding, C., Luan, S., Wang, Y., and Wang, Y.
(2017). Prioritizing influential factors for freeway
incident clearance time prediction using the gradient
boosting decision trees method. IEEE Transactions on
Intelligent Transportation Systems, 18(9):2303–2310.
Nagarajan, R., Scutari, M., and L
`
ebre, S. (2013). Bayesian
networks in r. Springer, 122:125–127.
Santos, D., Saias, J., Quaresma, P., and Nogueira, V. B.
(2021). Machine learning approaches to traffic ac-
cident analysis and hotspot prediction. Computers,
10(12):157.
Wang, C. (2010). The relationship between traffic conges-
tion and road accidents: an econometric approach us-
ing GIS. PhD thesis, © Chao Wang.
Yang, Y., Gao, X., Guo, Z., and Chen, D. (2019). Learning
bayesian networks using the constrained maximum a
posteriori probability method. Pattern Recognition,
91:123–134.
Zeng, L., Hu, X., Han, Q., Ye, L., Wang, R., He, X., and Xu,
Y. (2016). Abnormal hotspots detection method based
on region real-time congestion factor. In 2016 IEEE
19th International Conference on Intelligent Trans-
portation Systems (ITSC), pages 749–753. IEEE.
Zhang, J., Junhua, W., and Shou’en, F. (2019). Prediction of
urban expressway total traffic accident duration based
on multiple linear regression and artificial neural net-
work. In 2019 5th International Conference on Trans-
portation Information and Safety (ICTIS), pages 503–
510. IEEE.
VEHITS 2024 - 10th International Conference on Vehicle Technology and Intelligent Transport Systems
30