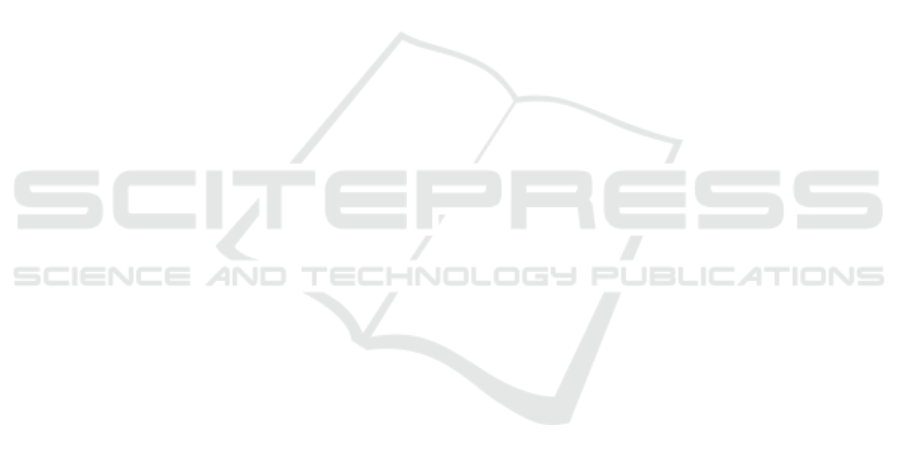
“if a child can produce the consonant clusters XY and
ZW in a specific position, then they can also produce
XW and ZY in the same position”. The phonological
assessment model studied in this work consists of 84
words, a considerably high number for a 3–7 years old
child to verbalize in a speech therapy session. With
our method, we could eliminate words from phono-
logical assessments by inferring consonant clusters
that the child can produce indirectly.
To validate our approach, we analyzed 1294
phonological assessments conducted in Southern
Brazil. Each assessment contained the 84 words pro-
posed by (Ceron et al., 2020), which were verbalized
by the children and transcribed in our database by ex-
perts in the field. In each assessment, we obtained a
list of consonant clusters that the child produced and
that were present in the target words, which are the
clusters expected to be produced by the child during
the assessment. The list of clusters that the child did
not produce was also collected.
Finally, we reanalyzed each assessment with our
method, going through all transcriptions and breaking
down the consonant clusters that the child produced
and did not produce into smaller phonetic parts. At
the end of the analysis, our method was able to pre-
dict with 97% accuracy the articulation ability of the
inferred consonant clusters. However, our approach
had a false positive rate of 12% and a false negative
rate of 8%, indicating that the method predicts more
than ideal and still has room for improvements.
As future work, we will include adaptations to the
method to predict consonant clusters that the child
would not be able to produce, as the negative predic-
tive value was 74%, a value slightly low compared
with the general accuracy of the method. Addition-
ally, we believe that there is potential to apply the
same logic to predict other types of phonemes in the
language, based on their similarity and position in
words.
In conclusion, our approach introduces a fast way
to infer the child’s capability of producing consonant
clusters, eliminating the need for more words in the
phonological assessment tool. By proposing a new
set of target words for an assessment, our method can
be used to discard the need to directly evaluate cer-
tain consonant clusters in the language. This also re-
duces the assessment time and subsequent report fill-
ing by the speech therapist, as fewer words would be
needed in the analysis. Thus, the assessment used
by speech therapists would become more efficient,
achieving equally reliable results with less effort.
REFERENCES
Armostis, S., Petinou, K., and Kyprianou, A. (2022). The
implementation of the ‘testing of phonological skills
(tops)’ tool: a computer-based phonological analysis
algorithm. Clinical Linguistics & Phonetics, 0(0):1–
20.
Attwell, G. A., Bennin, K. E., and Tekinerdogan, B. (2022).
A systematic review of online speech therapy systems
for intervention in childhood speech communication
disorders. Sensors, 22(24).
Bahar, N., Namasivayam, A. K., and van Lieshout, P.
(2022). Telehealth intervention and childhood apraxia
of speech: a scoping review. Speech, Language and
Hearing, 25(4):450 – 462.
Ceron, M. I., Gubiani, M. B., Oliveira, C. R. d., and Keske-
Soares, M. (2017). Factors influencing consonant ac-
quisition in brazilian portuguese–speaking children.
Journal of Speech, Language, and Hearing Research,
60(4):759–771.
Ceron, M. I., Gubiani, M. B., Oliveira, C. R. d., and Keske-
Soares, M. (2020). Phonological assessment instru-
ment (infono): A pilot study. CoDAS, 32(4).
Chronopoulos, S. K., Kosma, E. I., Peppas, K. P., Tafiadis,
D., Drosos, K., Ziavra, N., and Toki, E. I. (2021).
Exploring the speech language therapy through infor-
mation communication technologies, machine learn-
ing and neural networks. In 2021 5th International
Symposium on Multidisciplinary Studies and Innova-
tive Technologies (ISMSIT), pages 193–198.
Combiths, P., Amberg, R., Hedlund, G., Rose, Y., and Bar-
low, J. A. (2022). Automated phonological analysis
and treatment target selection using autopatt. Clini-
cal Linguistics & Phonetics, 36(2-3):203–218. PMID:
34085574.
Franciscatto, M. H., Lima, J. a. C. D., Trois, C., Maran,
V., Soares, M. K., and Rocha, C. C. d. (2019). A
case-based approach using phonological knowledge
for identifying error patterns in children’s speech. In
Proceedings of the 34th ACM/SIGAPP Symposium on
Applied Computing, SAC ’19, page 968–975, New
York, NY, USA. Association for Computing Machin-
ery.
Gallant, A., Watermeyer, J., and Sawasawa, C. (2023). Ex-
periences of south african speech-language therapists
providing telepractice during the covid-19 pandemic:
A qualitative survey. International journal of lan-
guage & communication disorders, 58.
H
´
odi, A. and T
´
oth, E. (2023). Exploring the opportuni-
ties for online assessment of phonological awareness
at the beginning of schooling. International Journal
of Early Childhood, pages 1–20.
Jothi, K. R. and Mamatha, V. L. (2020). A systematic re-
view of machine learning based automatic speech as-
sessment system to evaluate speech impairment. In
2020 3rd International Conference on Intelligent Sus-
tainable Systems (ICISS), pages 175–185.
Marques., J., Lima., J., Keske-Soares., M., Rocha., C., Ru-
bin., F., and Miollo., R. (2023). Algorithm for select-
ing words to compose phonological assessments. In
ICEIS 2024 - 26th International Conference on Enterprise Information Systems
222