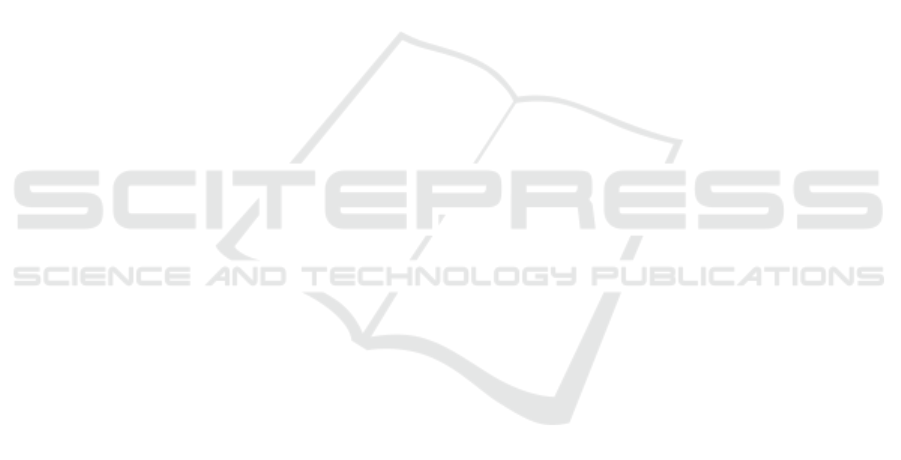
CIRAD-AFD (2021).
´
Etude sur les freins et leviers
`
a l’autosuffisance alimentaire : vers de nouveaux
mod
`
eles agricoles dans les d
´
epartements et r
´
egions
d’outre-mer. Technical report.
DAAF (2017). Chapitre 4: Gestion de l’eau et irriga-
tion. Technical report, Direction de l’Alimentation,
de l’Agriculture et de la For
ˆ
et.
DEAL (2020). Programmation pluriannuelle de l’
´
energie de
la r
´
eunion 2019-2028. Technical report, DEAL RE-
UNION.
Degenne, P. and Lo Seen, D. (2016). Ocelet: Simulat-
ing processes of landscape changes using interaction
graphs. SoftwareX, 5:89–95.
EDF-SEI (2023). Limitations de production au titre de la
s
ˆ
uret
´
e syst
`
eme. https://opendata-reunion.edf.fr/page
s/home0/.
Etalab (2023). Cadastre Etalab. https://cadastre.data.gouv.f
r/datasets/cadastre-etalab.
FAO (2001). FOOD BALANCE SHEETS - A Handbook.
Food and Agriculture Organization of the United Na-
tions.
Genave, A., Blancard, S., and Garabedian, S. (2020). An
assessment of energy vulnerability in small island de-
veloping states. Ecological Economics, 171:106595.
Guell, C., Brown, C. R., Navunicagi, O. W., Iese, V.,
Badrie, N., Wairiu, M., Saint Ville, A., and Unwin,
N. (2022). Perspectives on strengthening local food
systems in small island developing states. Food Secu-
rity, 14(5):1227–1240.
Holding, S., Allen, D. M., Foster, S., Hsieh, A., Larocque,
I., Klassen, J., and Van Pelt, S. C. (2016). Ground-
water vulnerability on small islands. Nature Climate
Change, 6(12):1100–1103.
INSEE (2018). Les besoins en logements
`
a la r
´
eunion
`
a
l’horizon 2035. Technical report, INSEE La R
´
eunion-
Mayotte.
Kim, K., Burnett, K., and Ghimire, J. (2015). Assessing the
potential for food and energy self-sufficiency on the
island of kauai, hawaii. Food Policy, 54:44–51.
Lajoie, G. and Hagen-Zanker, A. (2007). La simulation de
l’
´
etalement urbain
`
a la r
´
eunion : apport de l’automate
cellulaire metronamica® pour la prospective territori-
ale. Cybergeo: European Journal of Geography.
Le M
´
ezo, L., Dupuy, S., and Gaetano, R. (2022). La
R
´
eunion - occupation du sol - carte 2021 (Spot6/7)
- 1.5m. CIRAD.
Lin, Y.-C., Lin, C.-C., Lee, M., Chiueh, P.-T., Lo, S.-L., and
Liou, M.-L. (2019). Comprehensive assessment of re-
gional food-energy-water nexus with GIS-based tool.
Resources, Conservation and Recycling, 151:104457.
Lotfi, A., Mohammadi-Ivatloo, B., and Asadi, S. (2020).
Introduction to FEW Nexus. In Asadi, S. and
Mohammadi-Ivatloo, B., editors, Food-Energy-Water
Nexus Resilience and Sustainable Development:
Decision-Making Methods, Planning, and Trade-Off
Analysis, pages 29–56. Springer International Pub-
lishing, Cham.
Makungwe, M., Chabala, L. M., Van Dijk, M., Chishala,
B. H., and Lark, R. M. (2021). Assessing land suit-
ability for rainfed paddy rice production in zambia.
Geoderma Regional, 27:e00438.
Nebey, A. H., Taye, B. Z., and Workineh, T. G. (2020). Site
Suitability Analysis of Solar PV Power Generation in
South Gondar, Amhara Region. Journal of Energy,
2020:1–15.
OER (2023a). Bilan
´
energ
´
etique de la r
´
eunion - chiffres cl
´
es
2022. Technical report, SPL Energies R
´
eunion.
OER (2023b). Consommation d’
´
electricit
´
e. Technical re-
port, SPL Energies R
´
eunion.
OER (2023c).
´
Energies renouvelables la r
´
eunion. Technical
report, SPL Energies R
´
eunion.
PEIGEO (2021). Parc national de la r
´
eunion 2021. http:
//41.213.202.31:8080/geonetwork/srv/api/records/PN
Run.
Rodr
´
ıguez-Urrego, D., Ca
˜
nadillas-Ramallo, D., Gonz
´
alez-
D
´
ıaz, B., and Guerrero-Lemus, R. (2022). Analysis of
the water-energy nexus applied to an insular system:
Case study of tenerife. Sustainability, 14(3):1653.
Russeil, V. (2023). Perspectives d’autonomisation
alimentaire-
´
electrique de l’
ˆ
ıle de La R
´
eunion : en-
seignements de la mod
´
elisation spatiale dynamique et
de l’anticipation participative. PhD thesis,
´
Ecole doc-
torale Sciences, Technologies et Sant
´
e (Saint-Denis,
La R
´
eunion).
Russeil, V., Lo Seen, D., Broust, F., Bonin, M., and
Praene, J.-P. (2023). Food and electricity self-
sufficiency trade-offs in reunion island: Modelling
land-use change scenarios with stakeholders. Land
Use Policy, 132:106784.
Samara, F., Tampekis, S., Sakellariou, S., and
Christopoulou, O. (2015). Sustainable indica-
tors for land use planning evaluation: The case of a
small greek island. Fresenius Environmental Bulletin,
24:652–655.
Teng, P. (2020). Assuring food security in singapore, a
small island state facing COVID-19. Food Security,
12(4):801–804.
Trainor, A. M., McDonald, R. I., and Fargione, J. (2016).
Energy sprawl is the largest driver of land use change
in united states. PLOS ONE, 11(9):e0162269.
Weir, T. and Kumar, M. (2020). Renewable energy can en-
hance resilience of small islands. Natural Hazards,
104(3):2719–2725.
World Bank Group (2020). Solar resource maps
of Reunion. https://solargis.com/maps-and-gis-
data/download/reunion.
A Water-Energy-Food Nexus Approach to Assess Land Use Trade-Offs in Small Islands
37