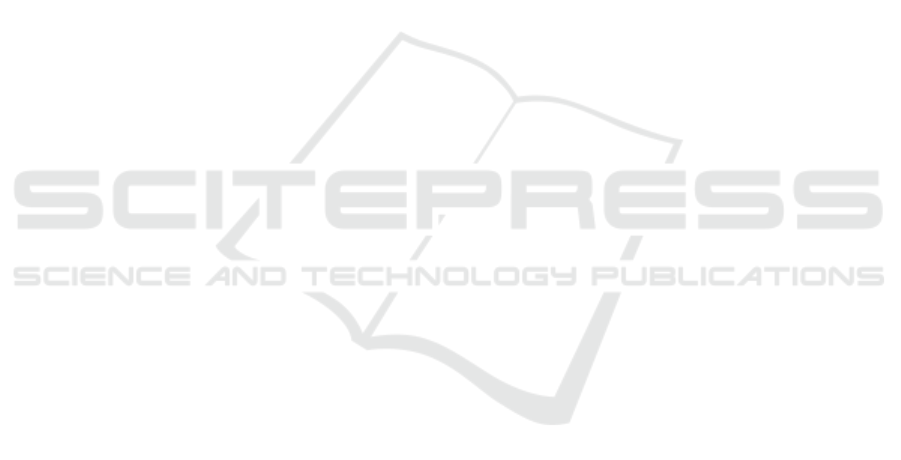
INSEE (2010). Les transports et deplacements des habitants
des Pays de la Loire.
Jordahl, K., Bossche, J. V. D., Fleischmann, M., Wasser-
man, J., McBride, J., Gerard, J., Tratner, J., Perry, M.,
Badaracco, A. G., Farmer, C., Hjelle, G. A., Snow,
A. D., Cochran, M., Gillies, S., Culbertson, L., Bartos,
M., Eubank, N., Maxalbert, Bilogur, A., Rey, S., Ren,
C., Arribas-Bel, D., Wasser, L., Wolf, L. J., Journois,
M., Wilson, J., Greenhall, A., Holdgraf, C., Filipe, and
Leblanc, F. (2020). Geopandas/geopandas: V0.8.1.
Zenodo.
Lam, T. M., Wang, Z., Vaartjes, I., Karssenberg, D., Ettema,
D., Helbich, M., Timmermans, E. J., Frank, L. D., den
Braver, N. R., Wagtendonk, A. J., Beulens, J. W. J.,
and Lakerveld, J. (2022). Development of an ob-
jectively measured walkability index for the Nether-
lands. International Journal of Behavioral Nutrition
and Physical Activity, 19(1):50.
Lynch, K. (1960). The Image of the City. Publication of
the Joint Center for Urban Studies. M.I.T. Press, Cam-
bridge, Mass., 33. print edition.
Maslow, A. H. (1943). A theory of human motivation. Psy-
chological Review, 50(4):370–396.
Moreno, C., Allam, Z., Chabaud, D., Gall, C., and Prat-
long, F. (2021). Introducing the “15-minute city”:
Sustainability, resilience and place identity in future
post-pandemic cities. Smart Cities, 4(1):93–111.
Nicoletti, L., Sirenko, M., and Verma, T. (2023). Disadvan-
taged communities have lower access to urban infras-
tructure. Environment and Planning B: Urban Analyt-
ics and City Science, 50(3):831–849.
P
´
aez, A., Scott, D. M., and Morency, C. (2012). Measuring
accessibility: Positive and normative implementations
of various accessibility indicators. Journal of Trans-
port Geography, 25:141–153.
python-visualization (2020). Folium, https://python-
visualization.github.io/folium/.
Rosso, A. L., Auchincloss, A. H., and Michael, Y. L.
(2011). The urban built environment and mobility in
older adults: A comprehensive review. Journal of Ag-
ing Research, 2011:816106.
Santos, A. C., Willumsen, J., Meheus, F., Ilbawi, A., and
Bull, F. C. (2023). The cost of inaction on physical in-
activity to public health-care systems: A population-
attributable fraction analysis. The Lancet Global
Health, 11(1):e32–e39.
Su, S., Pi, J., Xie, H., Cai, Z., and Weng, M. (2017). Com-
munity deprivation, walkability, and public health:
Highlighting the social inequalities in land use plan-
ning for health promotion. Land Use Policy, 67:315–
326.
Virtanen, P., Gommers, R., Oliphant, T. E., Haberland, M.,
Reddy, T., Cournapeau, D., Burovski, E., Peterson, P.,
Weckesser, W., Bright, J., Van Der Walt, S. J., Brett,
M., Wilson, J., Millman, K. J., Mayorov, N., Nel-
son, A. R. J., Jones, E., Kern, R., Larson, E., Carey,
C. J., Polat,
˙
I., Feng, Y., Moore, E. W., VanderPlas,
J., Laxalde, D., Perktold, J., Cimrman, R., Henrik-
sen, I., Quintero, E. A., Harris, C. R., Archibald,
A. M., Ribeiro, A. H., Pedregosa, F., Van Mulbregt,
P., SciPy 1.0 Contributors, Vijaykumar, A., Bardelli,
A. P., Rothberg, A., Hilboll, A., Kloeckner, A., Sco-
patz, A., Lee, A., Rokem, A., Woods, C. N., Ful-
ton, C., Masson, C., H
¨
aggstr
¨
om, C., Fitzgerald, C.,
Nicholson, D. A., Hagen, D. R., Pasechnik, D. V.,
Olivetti, E., Martin, E., Wieser, E., Silva, F., Lenders,
F., Wilhelm, F., Young, G., Price, G. A., Ingold, G.-
L., Allen, G. E., Lee, G. R., Audren, H., Probst, I.,
Dietrich, J. P., Silterra, J., Webber, J. T., Slavi
ˇ
c, J.,
Nothman, J., Buchner, J., Kulick, J., Sch
¨
onberger,
J. L., De Miranda Cardoso, J. V., Reimer, J., Har-
rington, J., Rodr
´
ıguez, J. L. C., Nunez-Iglesias, J.,
Kuczynski, J., Tritz, K., Thoma, M., Newville, M.,
K
¨
ummerer, M., Bolingbroke, M., Tartre, M., Pak, M.,
Smith, N. J., Nowaczyk, N., Shebanov, N., Pavlyk,
O., Brodtkorb, P. A., Lee, P., McGibbon, R. T., Feld-
bauer, R., Lewis, S., Tygier, S., Sievert, S., Vigna, S.,
Peterson, S., More, S., Pudlik, T., Oshima, T., Pin-
gel, T. J., Robitaille, T. P., Spura, T., Jones, T. R.,
Cera, T., Leslie, T., Zito, T., Krauss, T., Upadhyay,
U., Halchenko, Y. O., and V
´
azquez-Baeza, Y. (2020).
SciPy 1.0: Fundamental algorithms for scientific com-
puting in Python. Nature Methods, 17(3):261–272.
Xu, Y., Olmos, L. E., Abbar, S., and Gonz
´
alez, M. C.
(2020). Deconstructing laws of accessibility and
facility distribution in cities. Science Advances,
6(37):eabb4112.
Yang, Y. and Diez-Roux, A. V. (2012). Walking Distance
by Trip Purpose and Population Subgroups. American
Journal of Preventive Medicine, 43(1):11–19.
Zhao, J., Sun, G., and Webster, C. (2021). Walkability scor-
ing: Why and how does a three-dimensional pedes-
trian network matter? Environment and Planning B:
Urban Analytics and City Science, 48(8):2418–2435.
GISTAM 2024 - 10th International Conference on Geographical Information Systems Theory, Applications and Management
50