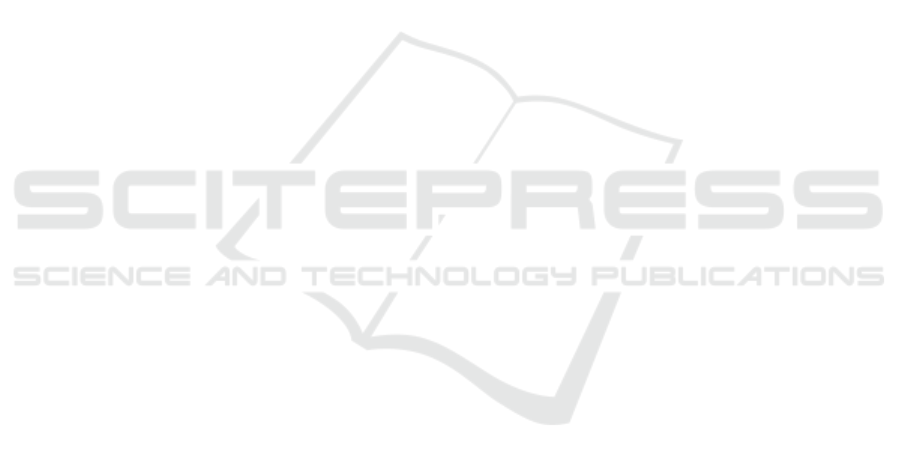
REFERENCES
Abadi, M., Agarwal, A., Barham, P., Brevdo, E., Chen,
Z., Citro, C., Corrado, G. S., Davis, A., Dean, J.,
Devin, M., Ghemawat, S., Goodfellow, I., Harp, A.,
Irving, G., Isard, M., Jia, Y., Jozefowicz, R., Kaiser,
L., Kudlur, M., Levenberg, J., Mane, D., Monga, R.,
Moore, S., Murray, D., Olah, C., Schuster, M., Shlens,
J., Steiner, B., Sutskever, I., Talwar, K., Tucker, P.,
Vanhoucke, V., Vasudevan, V., Viegas, F., Vinyals,
O., Warden, P., Wattenberg, M., Wicke, M., Yu, Y.,
and Zheng, X. (2016). TensorFlow: Large-Scale Ma-
chine Learning on Heterogeneous Distributed Sys-
tems. arXiv:1603.04467 [cs].
Alonso, I. and Murillo, A. C. (2019). EV-SegNet: Seman-
tic Segmentation for Event-Based Cameras. In 2019
IEEE/CVF Conference on Computer Vision and Pat-
tern Recognition Workshops (CVPRW), pages 1624–
1633, Long Beach, CA, USA. IEEE.
Benosman, R., Clercq, C., Lagorce, X., Ieng, S.-H., and
Bartolozzi, C. (2014). Event-Based Visual Flow.
IEEE Transactions on Neural Networks and Learn-
ing Systems, 25(2):407–417. Conference Name: IEEE
Transactions on Neural Networks and Learning Sys-
tems.
Bisulco, A., Cladera Ojeda, F., Isler, V., and Lee, D. D.
(2020). Near-Chip Dynamic Vision Filtering for Low-
Bandwidth Pedestrian Detection. In 2020 IEEE Com-
puter Society Annual Symposium on VLSI (ISVLSI),
pages 234–239. ISSN: 2159-3477.
Bolten, T., Neumann, C., Pohle-Fr
¨
ohlich, R., and T
¨
onnies,
K. (2023). N-MuPeTS: Event Camera Dataset for
Multi-Person Tracking and Instance Segmentation:.
In Proceedings of the 18th International Joint Con-
ference on Computer Vision, Imaging and Computer
Graphics Theory and Applications, pages 290–300,
Lisbon, Portugal. SCITEPRESS - Science and Tech-
nology Publications.
Bolten, T., Pohle-Frohlich, R., and Tonnies, K. D. (2021).
DVS-OUTLAB: A Neuromorphic Event-Based Long
Time Monitoring Dataset for Real-World Outdoor
Scenarios. In 2021 IEEE/CVF Conference on Com-
puter Vision and Pattern Recognition Workshops
(CVPRW), pages 1348–1357, Nashville, TN, USA.
IEEE.
Catalano, A., Bruno, F. A., Galliano, C., Pisco, M., Per-
siano, G. V., Cutolo, A., and Cusano, A. (2017). An
optical fiber intrusion detection system for railway se-
curity. Sensors and Actuators A: Physical, 253:91–
100.
de Tournemire, P., Nitti, D., Perot, E., Migliore, D., and
Sironi, A. (2020). A Large Scale Event-based Detec-
tion Dataset for Automotive. arXiv:2001.08499 [cs,
eess].
Delbruck, T. (2008). Frame-free dynamic digital vision. In
Proceedings of Intl. Symp. on Secure-Life Electron-
ics, Advanced Electronics for Quality Life and Soci-
ety, volume 1, pages 21–26. Citeseer.
D’Amore, P. and Tedesco, A. (2015). Technologies for
the Implementation of a Security System on Rail
Transportation Infrastructures. In Setola, R., Sforza,
A., Vittorini, V., and Pragliola, C., editors, Railway
Infrastructure Security, volume 27, pages 123–141.
Springer International Publishing, Cham. Series Ti-
tle: Topics in Safety, Risk, Reliability and Quality.
Iaboni, C., Kelly, T., and Abichandani, P. (2023). NU-
AIR – A Neuromorphic Urban Aerial Dataset for De-
tection and Localization of Pedestrians and Vehicles.
arXiv:2302.09429 [cs].
Jiang, Z., Xia, P., Huang, K., Stechele, W., Chen, G., Bing,
Z., and Knoll, A. (2019). Mixed Frame-/Event-Driven
Fast Pedestrian Detection. In 2019 International Con-
ference on Robotics and Automation (ICRA), pages
8332–8338, Montreal, QC, Canada. IEEE.
Jocher, G., Chaurasia, A., and Qiu, J. (2023). YOLO
by Ultralytics (Version 8.0.0) [Computer software].
https://github.com/ultralytics/ultralytics.
Miao, S., Chen, G., Ning, X., Zi, Y., Ren, K., Bing, Z.,
and Knoll, A. (2019). Neuromorphic Vision Datasets
for Pedestrian Detection, Action Recognition, and Fall
Detection. Frontiers in Neurorobotics, 13.
Mueggler, E., Forster, C., Baumli, N., Gallego, G., and
Scaramuzza, D. (2015). Lifetime estimation of events
from Dynamic Vision Sensors. In 2015 IEEE In-
ternational Conference on Robotics and Automation
(ICRA), pages 4874–4881. ISSN: 1050-4729.
Perez-Cutino, M., Eguiluz, A. G., Dios, J. M.-d., and
Ollero, A. (2021). Event-based human intrusion de-
tection in UAS using Deep Learning. In 2021 Inter-
national Conference on Unmanned Aircraft Systems
(ICUAS), pages 91–100, Athens, Greece. IEEE.
Perot, E., de Tournemire, P., Nitti, D., Masci, J., and
Sironi, A. (2020). Learning to Detect Objects with
a 1 Megapixel Event Camera. In Advances in Neu-
ral Information Processing Systems, volume 33, pages
16639–16652. Curran Associates, Inc.
Prophesee (2023). Metavision SDK by Prophesee (Version
4.1.0) [Computer Software].
Russakovsky, O., Deng, J., Su, H., Krause, J., Satheesh, S.,
Ma, S., Huang, Z., Karpathy, A., Khosla, A., Bern-
stein, M., Berg, A. C., and Fei-Fei, L. (2015). Ima-
geNet Large Scale Visual Recognition Challenge. In-
ternational Journal of Computer Vision, 115(3):211–
252.
Sandler, M., Howard, A., Zhu, M., Zhmoginov, A., and
Chen, L.-C. (2018). MobileNetV2: Inverted Resid-
uals and Linear Bottlenecks. In 2018 IEEE/CVF Con-
ference on Computer Vision and Pattern Recognition,
pages 4510–4520, Salt Lake City, UT. IEEE.
Siraj, A., Vaughn, R., and Bridges, S. (2004). Intrusion
sensor data fusion in an intelligent intrusion detection
system architecture. In 37th Annual Hawaii Interna-
tional Conference on System Sciences, 2004. Proceed-
ings of the, page 10 pp., Big Island, HI, USA. IEEE.
Wan, J., Xia, M., Huang, Z., Tian, L., Zheng, X., Chang, V.,
Zhu, Y., and Wang, H. (2021). Event-Based Pedes-
trian Detection Using Dynamic Vision Sensors. Elec-
tronics, 10(8):888. Number: 8 Publisher: Multidisci-
plinary Digital Publishing Institute.
Zhu, A. Z., Yuan, L., Chaney, K., and Daniilidis, K. (2019).
Unsupervised Event-Based Learning of Optical Flow,
Depth, and Egomotion. pages 989–997.
Intrusion Detection at Railway Tunnel Entrances Using Dynamic Vision Sensors
909