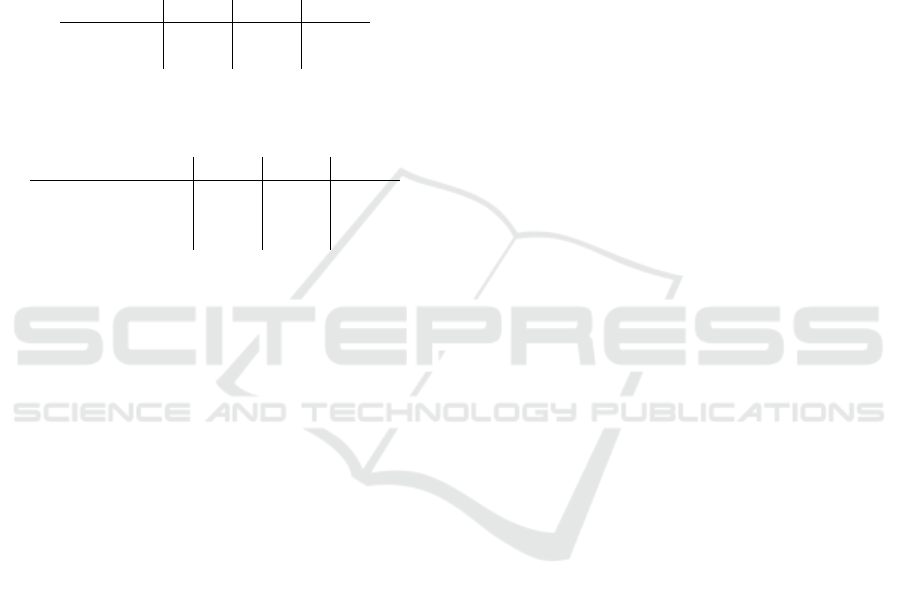
stead of a bib number-based metric, the reported pre-
cision (P), recall (R), and F-score (F) for the YOLOv7
detector are 0.776, 0.779, and 0.778, respectively,
which suggests promising performance also in severe
conditions. We remind again that among the 3223 bib
numbers contained in TGCRBNW, only 1000 were
captured in daylight. The reported values, as seen in
Table 3, suggest a slightly easier scenario with day-
light illumination conditions; however, there is still
much room for improvement.
Table 2: Bibs recognition results for TGCRBNW.
Approach P R F
EasyOCR 0.259 0.002 0.004
YOLOv7 0.216 0.047 0.077
Table 3: Digits recognition results for TGCRBNW includ-
ing overall and daylight and nightlight splits metrics.
TGCRBNW split P R F
Complete 0.776 0.779 0.777
Nightlight 0.714 0.714 0.714
Daylight 0.825 0.831 0.827
5 CONCLUSIONS
In this paper, we analyzed RBN recognition using
two available datasets: RBNR and TGCRBNW. To
achieve this, we evaluated two well-known general-
purpose OCRs and a specific digit detector trained
with samples from a different scenario, specifically
street numbers, using a general object detection ar-
chitecture. After an initial evaluation of RBNR, we
defined a new digit-based metric instead of an RBN-
based one to get more precise evidence of the par-
tially recognized RBNs. The results suggest that
the scenario defined by datasets similar to RBNR
is now solvable. However, the scenario presented
by TGCRBNW poses a real in-the-wild benchmark,
likely due to challenging features such as illumina-
tion conditions (nightlight, shadows, etc.), RBN reso-
lution, and motion blur, among others.
In any case, relying solely on RBN for recognition
is not enough due to the presence of occlusions, which
are common in such scenarios. Integrating other cues,
such as biometric traits, might help achieve coherent
recognition of specific runners, especially if a video
stream is available instead of single frames. However,
this concept is beyond the scope of the present paper.
ACKNOWLEDGEMENTS
This work is partially supported by the the Span-
ish Ministry of Science and Innovation under
project PID2021-122402OB-C22 and by the ACIISI-
Gobierno de Canarias and European FEDER funds
under projects ProID2021010012, ULPGC Facilities
Net, and Grant EIS 2021 04
REFERENCES
Apap, A. and Seychel, D. (2019). Marathon bib num-
ber recognition using deep learning. In 11th Inter-
national Symposium on Image and Signal Processing
and Analysis (ISPA), pages 21–26, Dubrovnik, Croa-
tia. IEEE.
Baek, Y., Lee, B., Han, D., Yun, S., and Lee, H. (2019).
Character region awareness for text detection. In Pro-
ceedings of the IEEE Conference on Computer Vision
and Pattern Recognition, pages 9365–9374.
Bartz, C., Yang, H., and Meinel, C. (2018). See: To-
wards semi-supervised end-to-end scene text recogni-
tion. Proceedings of the AAAI Conference on Artificial
Intelligence, 32(1).
Ben-Ami, I., (Basha), T. D., and Avidan, S. (2012). Racing
bib number recognition. In British Machine Vision
Conference, pages 1–10, Surrey, UK. British Machine
Vision Association.
Bochkovskiy, A., Wang, C.-Y., and Liao, H.-Y. M. (2020).
YOLOv4: Optimal speed and accuracy of object de-
tection. arXiv preprint arXiv:2004.10934.
Boonsim, N. (2018). Racing bib number localization on
complex backgrounds. WSEAS Transactions on Sys-
tems and Control, 13:226–231.
Carty, G., Raja, M. A., and Ryan, C. (2021). Running to get
recognised. In Thampi, S. M., Krishnan, S., Hegde,
R. M., Ciuonzo, D., Hanne, T., and Kannan R., J.,
editors, Advances in Signal Processing and Intelligent
Recognition Systems, pages 3–17, Singapore. Springer
Singapore.
Choi, Y., Napolean, Y., and van Gemert, J. C. (2021). The
arm-swing is discriminative in video gait recognition
for athlete re-identification. In IEEE International
Conference on Image Processing.
de Jes
´
us, W. M. and Borges, D. L. (2018). An improved
stroke width transform to detect race bib numbers.
In Proceedings of the Mexican Conference on Pat-
tern Recognition, pages 267–276, Puebla, Mexico.
Springer.
Hern
´
andez-Carrascosa, P., Penate-Sanchez, A., Lorenzo-
Navarro, J. F. O., and David; Castrill
´
on-Santana, M.
(2020). TGCRBNW: A dataset for runner bib num-
ber detection (and recognition) in the wild. In Pro-
ceedings International Conference on Pattern Recog-
nition, Milan, Italy.
Ivarsson, E. and Mueller, R. M. (2019). Racing bib num-
ber recognition using deep learning. In Proceedings of
the 25th Americas Conference on Information Systems
ICPRAM 2024 - 13th International Conference on Pattern Recognition Applications and Methods
916